What is multimodal AI? It’s a question we hear often these days, isn’t it? Whether during lunch breaks, in office chat groups, or while chatting with friends in the evening, it seems that everyone is abuzz with talk of GPT-4.
The recent release of GPT-4 has sparked a flurry of excitement and speculation within the AI community and beyond. As the latest addition to OpenAI’s impressive line of AI language models, GPT-4 boasts a range of advanced capabilities, particularly in the realm of multimodal AI.
With the ability to process and integrate inputs from multiple modalities, such as text, images, and sounds, GPT-4 represents a significant breakthrough in the field of AI and has generated considerable interest and attention from researchers, developers, and enthusiasts alike.
Since GPT-4’s release, everybody is discussing about the possibilities offered by multimodal AI. Let’s shed some light on this topic by going back to 6 months earlier first.
6 months earlier: Discussing multimodal AI
In a podcast interview titled “AI for the Next Era,” OpenAI’s CEO Sam Altman shared his insights on the upcoming advancements in AI technology. One of the highlights of the conversation was Altman’s revelation that a multimodal model is on the horizon.
The term “multimodal” refers to an AI’s ability to function in multiple modes, including text, images, and sounds.
OpenAI’s interactions with humans were restricted to text inputs, be it through Dall-E or ChatGPT. However, a multimodal AI would be capable of interacting through speech, enabling it to listen to commands, provide information, and even perform tasks. With the release of GPT-4, this might change for good.
I think we’ll get multimodal models in not that much longer, and that’ll open up new things. I think people are doing amazing work with agents that can use computers to do things for you, use programs and this idea of a language interface where you say a natural language – what you want in this kind of dialogue back and forth. You can iterate and refine it, and the computer just does it for you. You see some of this with DALL-E and CoPilot in very early ways.
-Altman
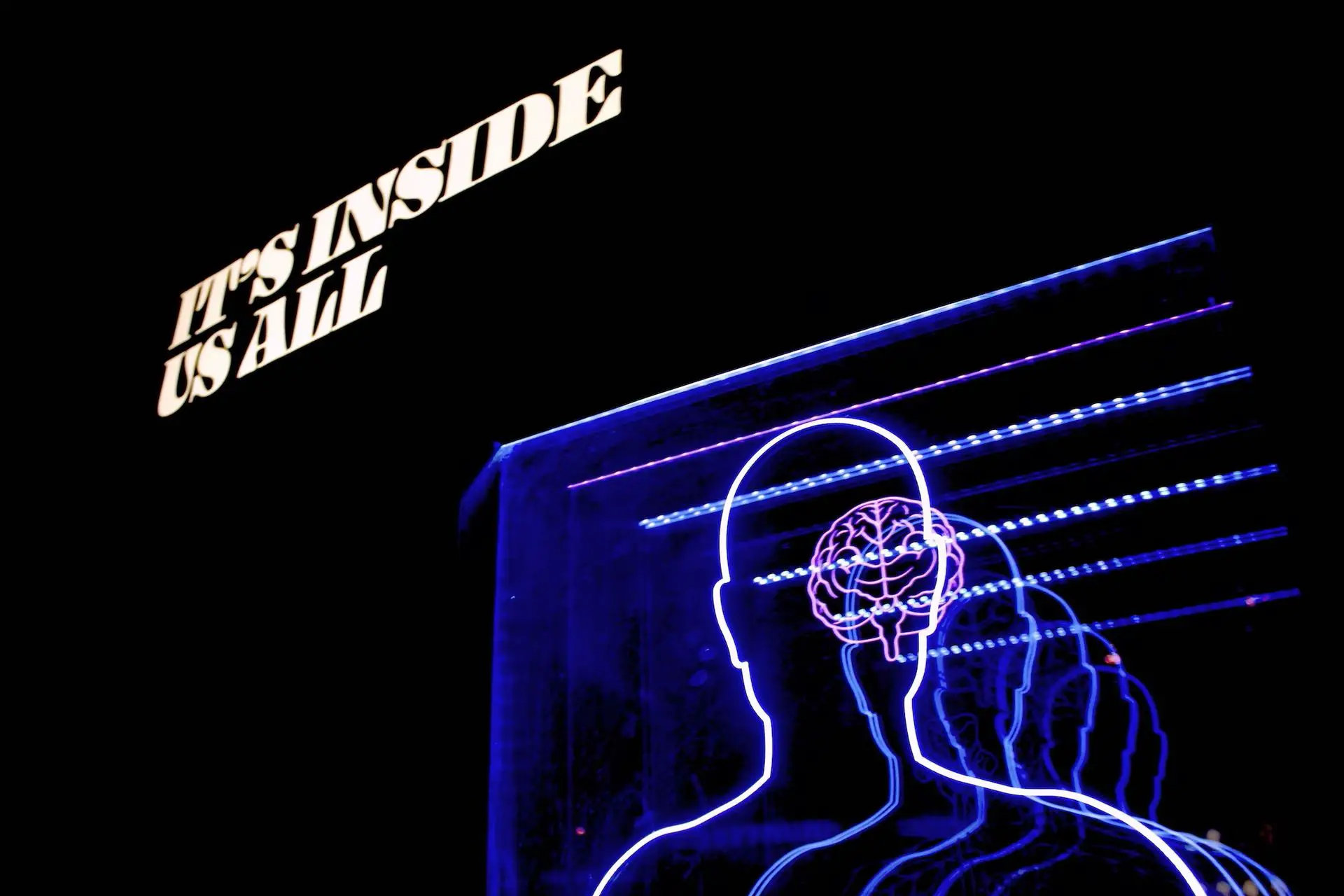
Although Altman did not explicitly confirm that GPT-4 would be multimodal in that time, he did suggest that such technology is on the horizon and will arrive in the near future. One intriguing aspect of his vision for multimodal AI is its potential to create new business models that are not currently feasible.
Altman drew a parallel to the mobile platform, which created countless opportunities for new ventures and jobs. In the same way, a multimodal AI platform could unlock a host of innovative possibilities and transform the way we live and work. It’s an exciting prospect that underscores the transformative power of AI and its capacity to reshape our world in ways we can only imagine.
…I think this is going to be a massive trend, and very large businesses will get built with this as the interface, and more generally [I think] that these very powerful models will be one of the genuine new technological platforms, which we haven’t really had since mobile. And there’s always an explosion of new companies right after, so that’ll be cool. I think we will get true multimodal models working. And so not just text and images but every modality you have in one model is able to easily fluidly move between things.
-Altman
A truly self-learning AI
One area that receives comparatively little attention in the realm of AI research is the quest to create a self-learning AI. While current models are capable of spontaneous understanding, or “emergence,” where new abilities arise from increased training data, a truly self-learning AI would represent a major leap forward.
OpenAI’s Altman spoke of an AI that can learn and upgrade its abilities on its own, rather than being dependent on the size of its training data. This kind of AI would transcend the traditional software version paradigm, where companies release incremental updates, instead growing and improving autonomously.
Although Altman did not suggest that GPT-4 will possess this capability, he did suggest that it is something that OpenAI is working towards and is entirely within the realm of possibility. The idea of a self-learning AI is an intriguing one that could have far-reaching implications for the future of AI and our world.
Visual ChatGPT brings AI image generation to the popular chatbot
Back to the present: GPT-4 is released
The much-anticipated release of GPT-4 is now available to some Plus subscribers, featuring a new multimodal language model that accepts text, speech, images, and video as inputs and provides text-based answers.
OpenAI has touted GPT-4 as a significant milestone in its efforts to scale up deep learning, noting that while it may not outperform humans in many real-world scenarios, it delivers human-level performance on various professional and academic benchmarks.
The popularity of ChatGPT, which utilizes GPT-3 AI technology to generate human-like responses to search queries based on data gathered from the internet, has surged since its debut on November 30th.
The launch of ChatGPT, a conversational chatbot, has sparked an AI arms race between Microsoft and Google, both of which aim to integrate content-creating generative AI technologies into their internet search and office productivity products. The release of GPT-4 and the ongoing competition among tech giants highlights the growing importance of AI and its potential to transform the way we interact with technology.
To better understand the topic, we invite you to delve into a deeper and more technical discussion of multimodal AI.
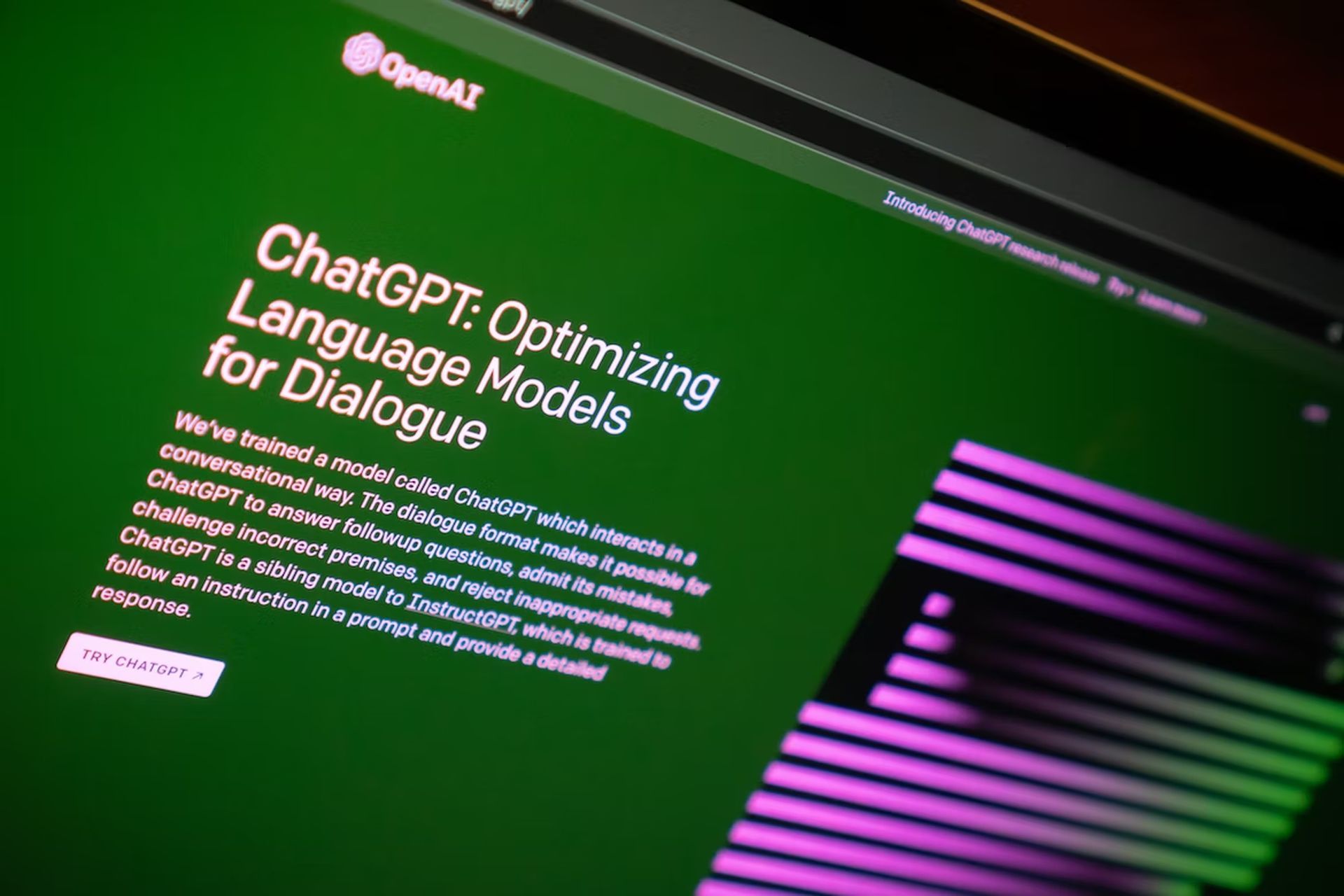
What is multimodal AI?
Multimodal AI is a type of artificial intelligence that has the ability to process and understand inputs from different modes or modalities, including text, speech, images, and videos. This means that it can recognize and interpret various forms of data, not just one type, which makes it more versatile and adaptable to different situations. In essence, multimodal AI can “see,” “hear,” and “understand” like a human, allowing it to interact with the world in a more natural and intuitive way.
Applications of multimodal AI
The abilities of multimodal AI are vast and wide-ranging. Here are some examples of what multimodal AI can do:
- Speech recognition: Multimodal AI can understand and transcribe spoken language, allowing it to interact with users through voice commands and natural language processing.
- Image and video recognition: Multimodal AI can analyze and interpret visual data, such as images and videos, to identify objects, people, and activities.
- Textual analysis: Multimodal AI can process and understand written text, including natural language processing, sentiment analysis, and language translation.
- Multimodal integration: Multimodal AI can combine inputs from different modalities to form a more complete understanding of a situation. For example, it can use both visual and audio cues to recognize a person’s emotions.
How does multimodal AI work?
Multimodal neural networks are typically composed of several unimodal neural networks, with an audiovisual model being an example of two such networks – one for visual data and one for audio data. These individual networks process their respective inputs separately, in a process known as encoding.
Once unimodal encoding is completed, the extracted information from each model needs to be combined. Various fusion techniques have been proposed for this purpose, ranging from basic concatenation to the use of attention mechanisms. Multimodal data fusion is a critical factor in achieving success in these models.
After fusion, the final stage involves a “decision” network that accepts the encoded and fused information and is trained on the specific task.
In essence, multimodal architectures consist of three essential components – unimodal encoders for each input modality, a fusion network that combines the features of the different modalities, and a classifier that makes predictions based on the fused data.
Comparison with current AI models
Compared to traditional AI models that can only handle one type of data at a time, multimodal AI has several advantages, including:
- Versatility: Multimodal AI can handle multiple types of data, making it more adaptable to different situations and use cases.
- Natural interaction: By integrating multiple modalities, multimodal AI can interact with users in a more natural and intuitive way, similar to how humans communicate.
- Improved accuracy: By combining inputs from different modalities, multimodal AI can improve the accuracy of its predictions and classifications.
Here’s a summary table comparing different AI models:
AI Model | Data Type | Applications |
Text-based AI | Text | Natural Language Processing, Chatbots, Sentiment Analysis |
Image-based AI | Images | Object Detection, Image Classification, Facial Recognition |
Speech-based AI | Audio | Voice Assistants, Speech Recognition, Transcription |
Multimodal AI | Text, Images, Audio, Video | Natural Interaction, Contextual Understanding, Improved Accuracy |
Why multimodal AI is important?
Multimodal AI is important because it has the potential to transform how we interact with technology and machines. By enabling more natural and intuitive interactions through multiple modalities, multimodal AI can create more seamless and personalized user experiences. This can be especially beneficial in areas such as:
- Healthcare: Multimodal AI can help doctors and patients communicate more effectively, especially for those who have limited mobility or are non-native speakers of a language.
- Education: Multimodal AI can improve learning outcomes by providing more personalized and interactive instruction that adapts to a student’s individual needs and learning style.
- Entertainment: Multimodal AI can create more immersive and engaging experiences in video games, movies, and other forms of media.
Advantages of multimodal AI
Here are some of the key advantages of multimodal AI:
- Contextual understanding: By combining inputs from multiple modalities, multimodal AI can gain a more complete understanding of a situation, including the context and meaning behind the data.
- Natural interaction: By enabling more natural and intuitive interactions through multiple modalities, multimodal AI can create more seamless and personalized user experiences.
- Improved accuracy: By integrating multiple sources of data, multimodal AI can improve the accuracy of its predictions and classifications.
Creating an artificial intelligence 101
Potential for creating new business models
Multimodal AI also has the potential to create new business models and revenue streams. Here are some examples:
- Voice assistants: Multimodal AI can enable more sophisticated and personalized voice assistants that can interact with users through speech, text, and visual displays.
- Smart homes: Multimodal AI can create more intelligent and responsive homes that can understand and adapt to a user’s preferences and behaviors.
- Virtual shopping assistants: Multimodal AI can help customers navigate and personalize their shopping experience through voice and visual interactions.
Future of AI technology
The future of AI technology is exciting, with researchers exploring new ways to create more advanced and sophisticated AI models. Here are some key areas of focus:
- Self-learning AI: AI researchers aim to create AI that can learn and improve on its own, without the need for human intervention. This could lead to more adaptable and resilient AI models that can handle a wide range of tasks and situations.
- Multimodal AI: As discussed earlier, multimodal AI has the potential to transform how we interact with technology and machines. AI experts are working on creating more sophisticated and versatile multimodal AI models that can understand and process inputs from multiple modalities.
- Ethics and governance: As AI becomes more powerful and ubiquitous, it’s essential to ensure that it’s used ethically and responsibly. AI researchers are exploring ways to create more transparent and accountable AI systems that are aligned with human values and priorities.
How AI researchers aim to create AI that can learn by itself?
AI researchers are exploring several approaches to creating AI that can learn by itself. One promising area of research is called reinforcement learning, which involves teaching an AI model to make decisions and take actions based on feedback from the environment. Another approach is called unsupervised learning, which involves training an AI model on unstructured data and letting it find patterns and relationships on its own. By combining these and other approaches, AI researchers hope to create more advanced and autonomous AI models that can improve and adapt over time.
All about autonomous intelligence: A comprehensive overview
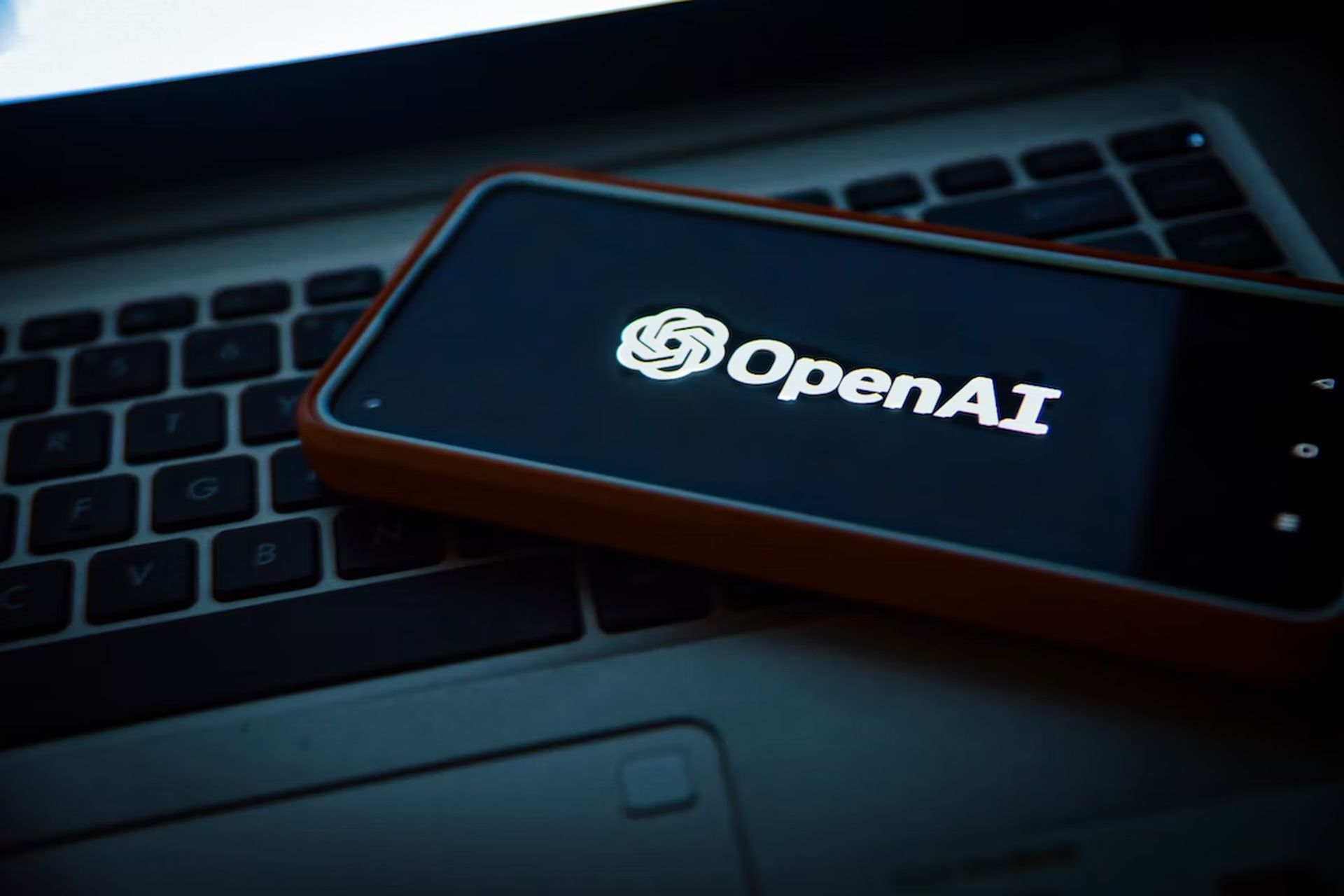
Potential for improved AI models
Improved AI models have the potential to transform how we live and work. Here are some potential benefits of improved AI models:
- Improved accuracy: As AI models become more sophisticated and advanced, they can improve their accuracy and reduce errors in areas such as medical diagnosis, financial forecasting, and risk assessment.
- More personalized experiences: Advanced AI models can personalize user experiences by understanding individual preferences and behaviors. For example, a music streaming service can recommend songs based on a user’s listening history and mood.
- Automation of tedious tasks: AI can automate tedious and repetitive tasks, freeing up time for humans to focus on more creative and high-level tasks.
GPT-4 and multimodal AI
After much anticipation and speculation, OpenAI has finally revealed the latest addition to its impressive line of AI language models. Dubbed GPT-4, the system promises to deliver groundbreaking advancements in multimodal AI, albeit with a more limited range of input modalities than some had predicted.
Announcing GPT-4, a large multimodal model, with our best-ever results on capabilities and alignment: https://t.co/TwLFssyALF pic.twitter.com/lYWwPjZbSg
— OpenAI (@OpenAI) March 14, 2023
According to OpenAI, the model can process both textual and visual inputs, providing text-based outputs that demonstrate a sophisticated level of comprehension. With its ability to simultaneously interpret and integrate multiple modes of input, GPT-4 marks a significant milestone in the development of AI language models that have been building momentum for several years before capturing mainstream attention in recent months.
OpenAI’s groundbreaking GPT models have captured the imagination of the AI community since the publication of the original research paper in 2018. Following the announcement of GPT-2 in 2019 and GPT-3 in 2020, these models have been trained on vast datasets of text, primarily sourced from the internet, which is then analyzed for statistical patterns. This simple yet highly effective approach enables the models to generate and summarize writing, as well as perform a range of text-based tasks such as translation and code generation.
Despite concerns over the potential misuse of GPT models, OpenAI finally launched its ChatGPT chatbot based on GPT-3.5 in late 2022, making the technology accessible to a wider audience. This move triggered a wave of excitement and anticipation in the tech industry, with other major players such as Microsoft and Google quickly following suit with their own AI chatbots, including Bing as part of the Bing search engine. The launch of these chatbots demonstrates the growing importance of GPT models in shaping the future of AI, and their potential to transform the way we communicate and interact with technology.
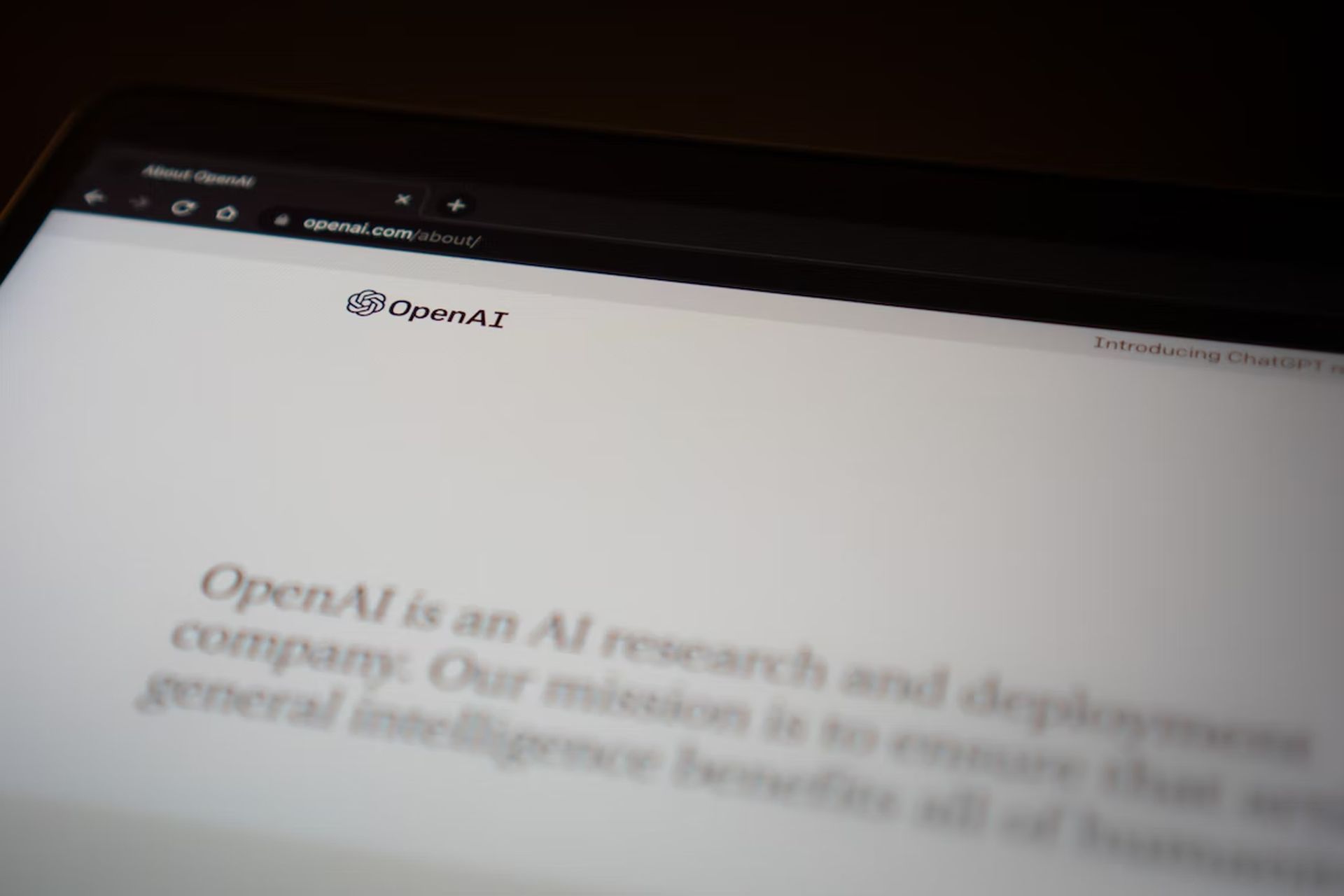
As expected, the increasing accessibility of AI language models has presented a range of problems and challenges for various sectors. For example, the education system has struggled to cope with the emergence of software that is capable of generating high-quality college essays. Likewise, online platforms such as Stack Overflow and Clarkesworld have been forced to halt submissions due to an overwhelming influx of AI-generated content. Even early applications of AI writing tools in journalism have encountered difficulties.
Despite these challenges, some experts contend that the negative impacts have been somewhat less severe than initially predicted. As with any new technology, the introduction of AI language models has required careful consideration and adaptation to ensure that the benefits of the technology are maximized while minimizing any adverse effects.
Accoring to OpenAI, GPT-4 had gone through six months of safety training, and that in internal tests, it was “82 percent less likely to respond to requests for disallowed content and 40 percent more likely to produce factual responses than GPT-3.5.”
Bottom line
Circling back to our initial topic: What is multimodal AI? Just six months ago, the concept of multimodal AI was still largely confined to the realm of theoretical speculation and research. However, with the recent release of GPT-4, we are now witnessing a major shift in the development and adoption of this technology. The capabilities of GPT-4, particularly in its ability to process and integrate inputs from multiple modalities, have opened up a whole new world of possibilities and opportunities for the field of AI and beyond.
We will see a rapid expansion of multimodal AI applications across a wide range of industries and sectors. From healthcare and education to entertainment and gaming, the ability of AI models to understand and respond to inputs from multiple modalities is transforming how we interact with technology and machines. This technology is enabling us to communicate and collaborate with machines in a more natural and intuitive manner, with significant implications for the future of work and productivity.
- SEO Powered Content & PR Distribution. Get Amplified Today.
- Platoblockchain. Web3 Metaverse Intelligence. Knowledge Amplified. Access Here.
- Source: https://dataconomy.com/2023/03/what-is-multimodal-ai-gpt-4/