Do you remember when Google’s Bard, now called Gemini, couldn’t answer a question about the James Webb Space Telescope in its launch ad? Let me jog your memory about this famous case—it’s one of the biggest examples of AI hallucinations we’ve seen.
What went down? During the ad, the Google Bard demo was asked, “What new discoveries from the James Webb Space Telescope can I share with my 9-year-old?” After a brief pause, the Google Bard demo gave two correct responses. However, its final answer was incorrect. According to the Google Bard demo, the telescope had captured the first images of a planet outside our solar system. However, in reality, the Very Large Telescope at the European Southern Observatory had already snapped images of these “exoplanets,” which were stored in NASA’s archives.
Not to be a ~well, actually~ jerk, and I’m sure Bard will be impressive, but for the record: JWST did not take “the very first image of a planet outside our solar system”.
the first image was instead done by Chauvin et al. (2004) with the VLT/NACO using adaptive optics. https://t.co/bSBb5TOeUW pic.twitter.com/KnrZ1SSz7h
— Grant Tremblay (@astrogrant) February 7, 2023
Why can’t tech giants like Google even prevent such mistakes? Let’s find out!
What is an AI hallucination?
An AI hallucination occurs when a computer program, typically powered by artificial intelligence (AI), produces outputs that are incorrect, nonsensical, or misleading. This term is often used to describe situations where AI models generate responses that are completely off track or unrelated to the input they were given. It’s like asking a question and getting an answer that doesn’t make any sense or isn’t at all what you expected.
To understand why AI hallucinations happen, let’s look at how AI works. AI models, such as chatbots or image recognition systems, are trained on large datasets to learn patterns and make predictions. However, if the training data is flawed, incomplete, or biased, the AI model may learn incorrect patterns or associations. This can lead to hallucinated outputs where the AI generates responses that are based on faulty or misunderstood information. And it occurs a lot even though you might not noticed.
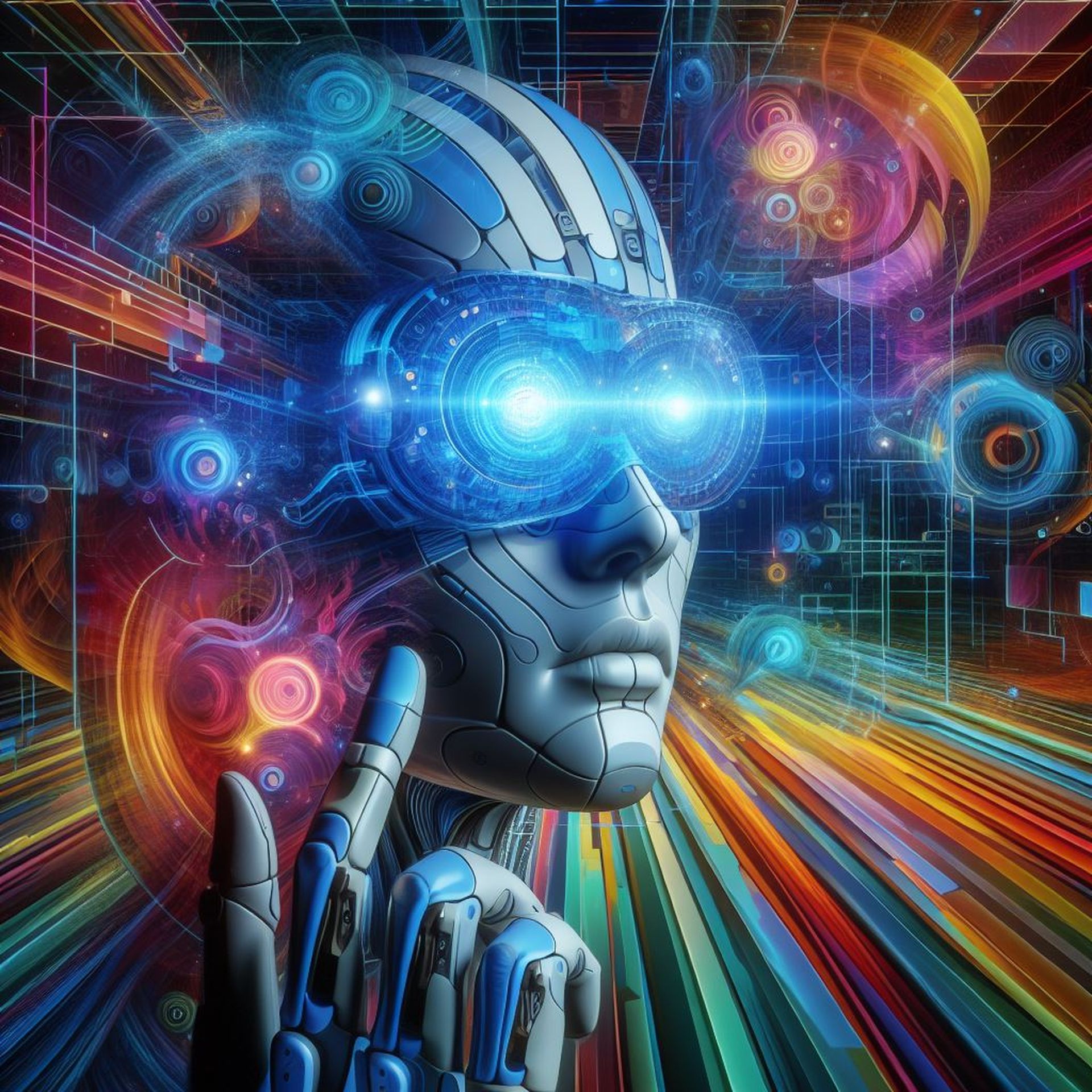
AI hallucinations can manifest in various forms, each highlighting different challenges and intricacies within artificial intelligence systems. Here are some common types of AI hallucinations:
- Sentence AI hallucination:
- In this scenario, the AI model generates a sentence that conflicts with a previous statement within the same context. For instance:
- Prompt: “Describe a character’s journey in three-word sentences.”
- Output: “She walked slowly. Suddenly, she ran. Then, she walked.”
- In this scenario, the AI model generates a sentence that conflicts with a previous statement within the same context. For instance:
The last sentence contradicts the earlier action of running, disrupting the coherence of the narrative.
- Prompt AI hallucination:
- This type occurs when the AI-generated response clashes with the intended prompt or query. For example:
- Prompt: “Write a recipe for chocolate cake.”
- Output: “Here’s a poem about stars.”
- This type occurs when the AI-generated response clashes with the intended prompt or query. For example:
The generated content does not fulfill the cooking-related prompt, indicating a failure to grasp the context.
- Factual AI hallucination:
- Factual contradictions arise when the AI model presents false information as accurate. For instance:
- Prompt: “List three elements in the periodic table.”
- Output: “Hydrogen, Oxygen, Carbonite.”
- Factual contradictions arise when the AI model presents false information as accurate. For instance:
“Carbonite” is not an element, leading to misinformation in the response.
- Irrelevant or random AI hallucinations:
- These hallucinations involve the generation of irrelevant or nonsensical information unrelated to the input. For example:
- Prompt: “Describe a day at the beach.”
- Output: “The sand was warm. Seagulls sang jazz. Penguins danced ballet.”
- These hallucinations involve the generation of irrelevant or nonsensical information unrelated to the input. For example:
The mention of penguins and ballet does not align with the typical beach scene, demonstrating a lack of coherence in the generated narrative.
These types of hallucinations underscore the challenges AI systems face in understanding and contextualizing information accurately. Addressing these issues requires improving the quality of training data, refining language models’ understanding of context, and implementing robust validation mechanisms to ensure the coherence and accuracy of AI-generated outputs.
AI hallucinations can have serious consequences, especially in applications where AI is used to make important decisions, such as medical diagnosis or financial trading. If an AI system hallucinates and provides inaccurate information in these contexts, it could lead to harmful outcomes.
What can you do about AI hallucinations?
Reducing AI hallucinations involves a few key steps to make AI systems more accurate and reliable:
Firstly, it’s crucial to use good quality data to train AI. This means making sure the information AI learns from is diverse, accurate, and free from biases.
Simplifying AI models can also help. Complex models can sometimes lead to unexpected mistakes. By keeping things simple, we can reduce the chances of errors.
Clear and easy-to-understand instructions are important too. When AI gets clear inputs, it’s less likely to get confused and make mistakes.
Regular testing helps catch any mistakes early on. By checking how well AI is performing, we can fix any issues and make improvements.
Adding checks within AI systems can also help. These checks look out for mistakes and correct them before they cause problems.
Most importantly, human oversight is essential. Having people double-check AI-generated outputs ensures accuracy and reliability.
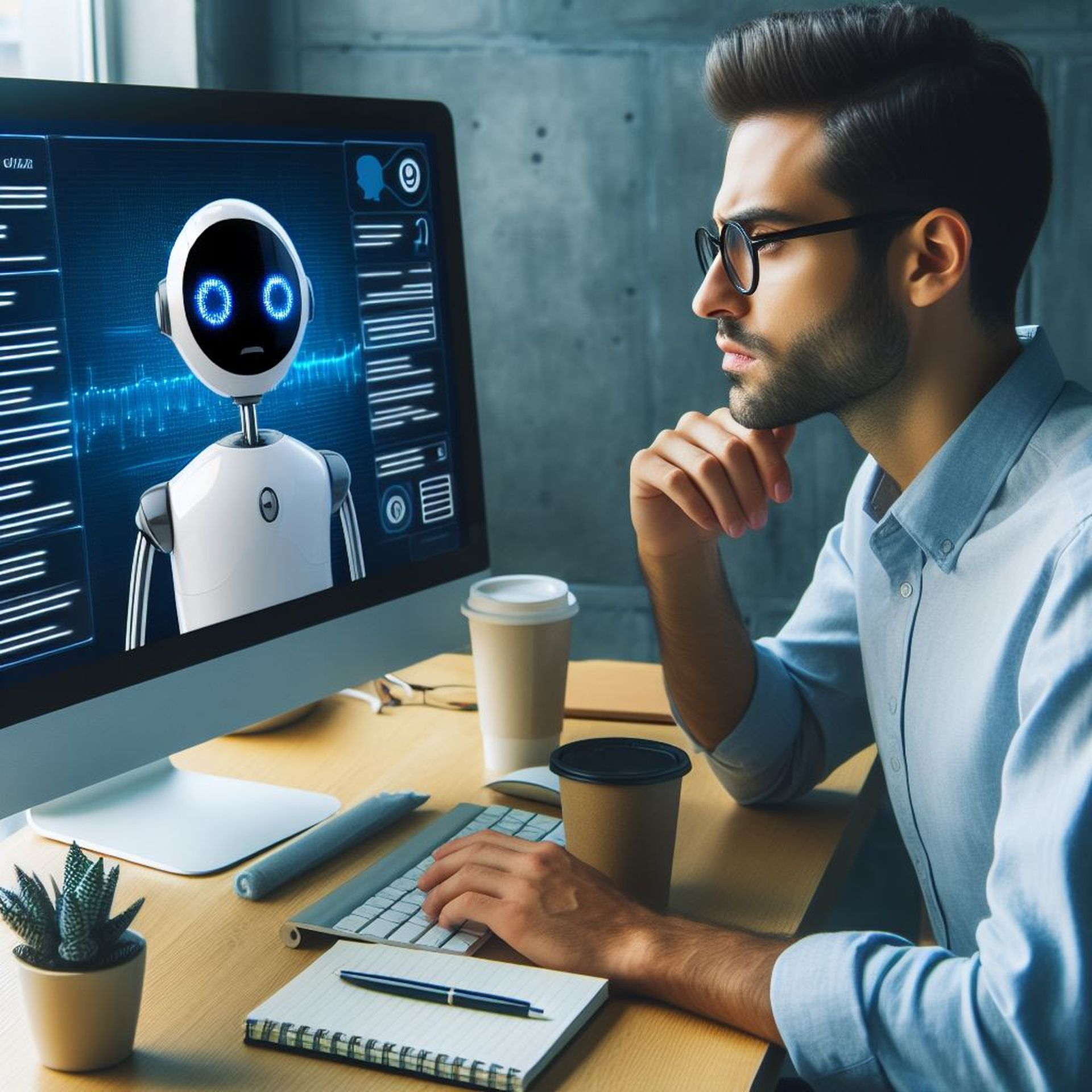
Lastly, training AI to defend against attacks can make it more resilient. This helps AI recognize and deal with attempts to manipulate or trick it.
By following these steps, we can make AI systems more reliable and reduce the chances of hallucinated outputs.
How do you make an AI hallucinate?
If you want to take advantage of this flaw and have some fun, you can do a few things:
- Change the input: You can tweak the information given to the AI. Even small changes can make it produce strange or incorrect answers.
- Trick the model: Craft special inputs that fool the AI into giving wrong answers. These tricks exploit the model’s weaknesses to create hallucinated outputs.
- Mess with the data: By adding misleading or incorrect information to the AI’s training data or your prompt, you can make it learn the wrong things and produce hallucinations.
- Adjust the model: Modify the AI’s settings or structure to introduce flaws or biases. These changes can cause it to generate strange or nonsensical outputs.
- Give confusing inputs: Provide the AI with unclear or contradictory instructions. This can confuse the AI and lead to incorrect or nonsensical responses.
Or you can just ask questions and try your luck! For example, we tried and make ChatGPT hallucinate:
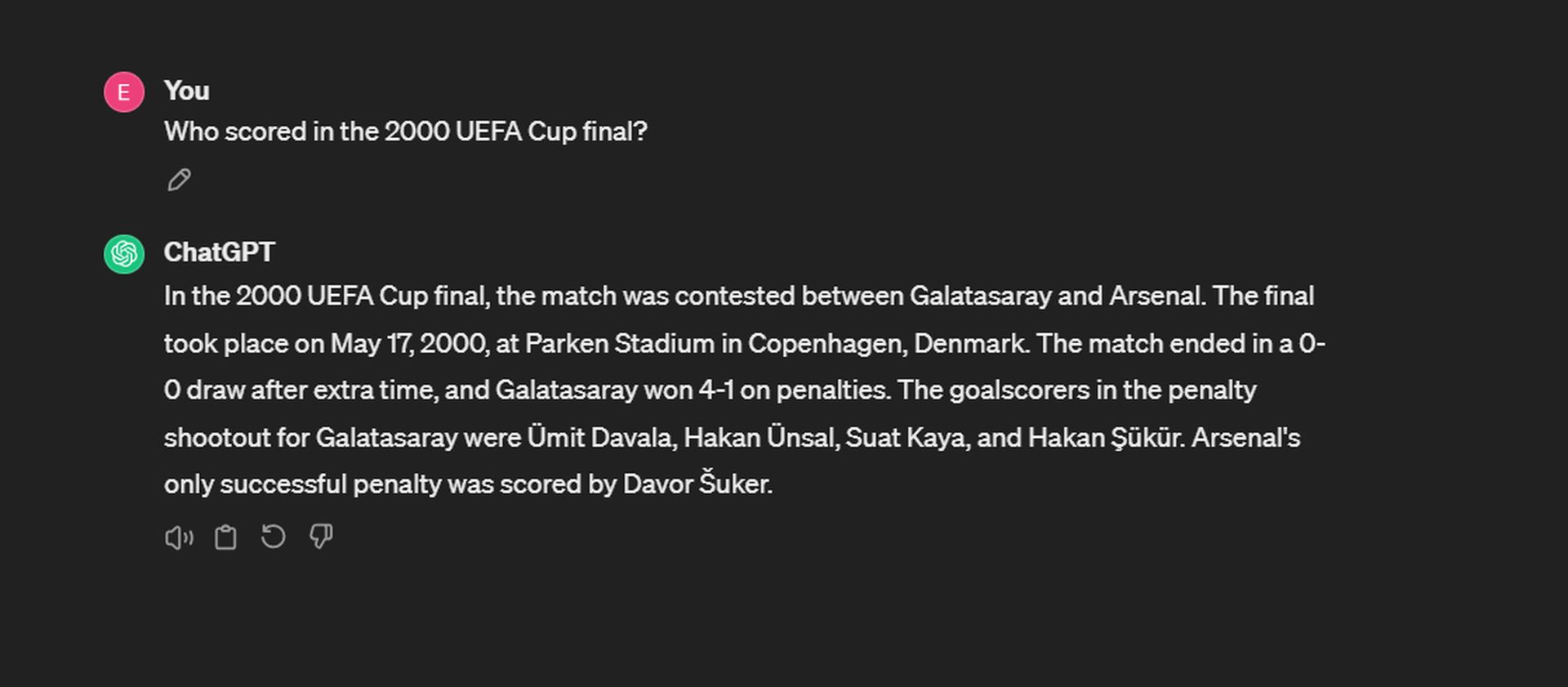
While ChatGPT accurately remembers the date and score, it falters in recalling the penalty shootout’s scorers. For Galatasaray, the goals were netted by Ergün Penbe, Hakan Şükür, Ümit Davala, and Popescu. On the Arsenal side, Ray Parlour was the sole successful penalty scorer.
While making AI hallucinate can help us understand its limitations, it’s important to use this knowledge responsibly and ensure that AI systems remain reliable and trustworthy.
Does GPT 4 hallucinate less?
Yes, according to the evaluation conducted by the Palo Alto-based company using their Hallucination Evaluation Model, GPT-4 demonstrates a lower hallucination rate compared to other large language models, except Intel Neural Chat 7B (97.2%). With an accuracy rate of 97% and a hallucination rate of 3%, GPT-4 exhibits a high level of accuracy and a relatively low tendency to introduce hallucinations when summarizing documents. This indicates that GPT-4 is less prone to generating incorrect or nonsensical outputs compared to other models tested in the evaluation.
On the flip side, few of the least effective models came from Google. Google Palm 2 demonstrated an accuracy rate of 90% and a hallucination rate of 10%. Its chat-refined counterpart performed even worse, with an accuracy rate of only 84.2% and the highest hallucination score of any model on the leaderboard at 16.2 %. Here is the list:
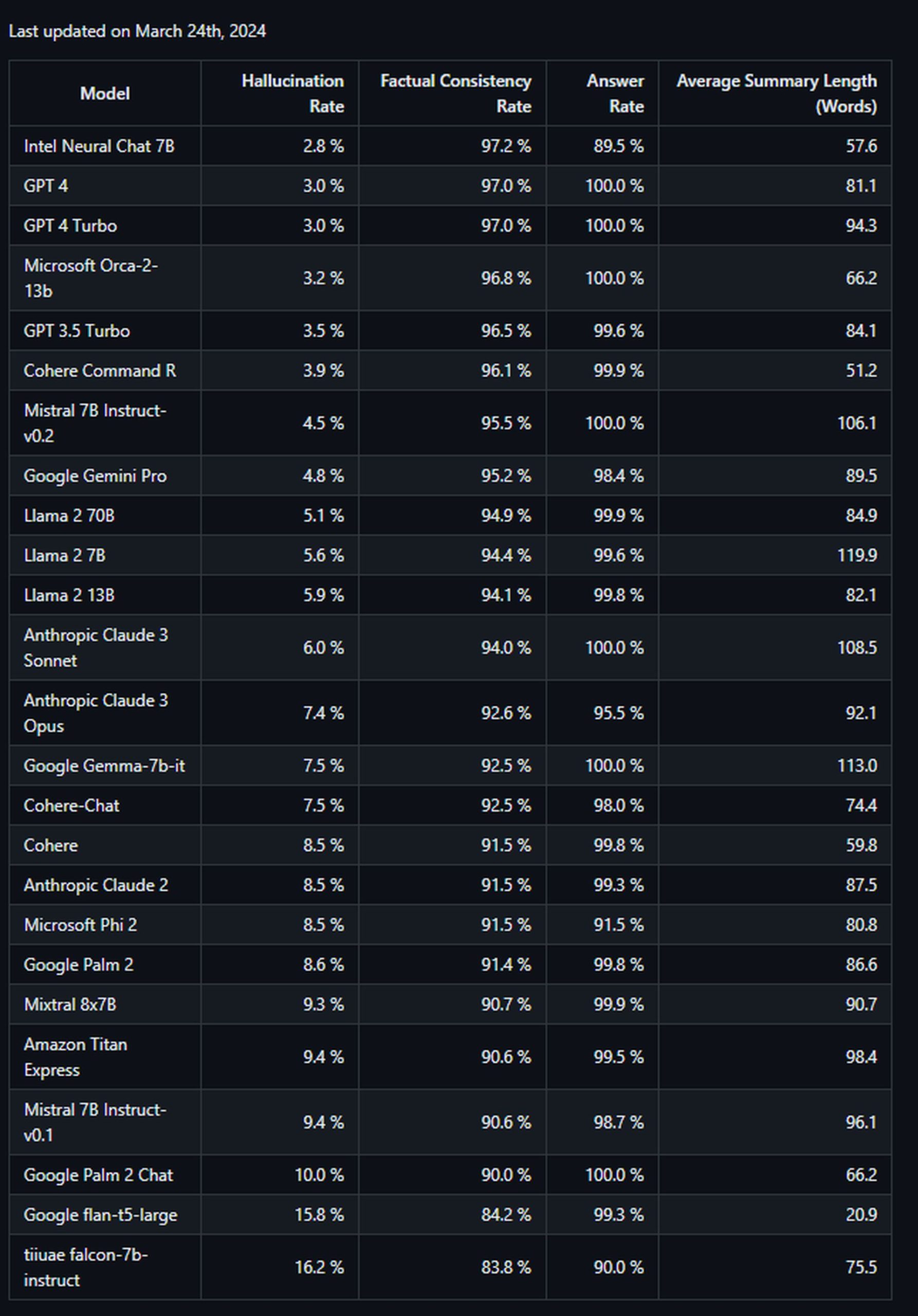
In summary, an AI hallucination is a mistake made by AI systems where they produce outputs that are nonsensical or incorrect due to flaws in the training data or the way they process information. It’s a fascinating, yet challenging aspect of AI that researchers and developers are working to address.
- SEO Powered Content & PR Distribution. Get Amplified Today.
- PlatoData.Network Vertical Generative Ai. Empower Yourself. Access Here.
- PlatoAiStream. Web3 Intelligence. Knowledge Amplified. Access Here.
- PlatoESG. Carbon, CleanTech, Energy, Environment, Solar, Waste Management. Access Here.
- PlatoHealth. Biotech and Clinical Trials Intelligence. Access Here.
- Source: https://dataconomy.com/2024/04/11/what-exactly-is-an-ai-hallucination-and-why-does-it-matter/