<!—->
<!– –>

The emergence of generative AI prompted several prominent companies to restrict its use because of the mishandling of sensitive internal data. According to CNN, some companies imposed internal bans on generative AI tools while they seek to better understand the technology and many have also blocked the use of internal ChatGPT.
Companies still often accept the risk of using internal data when exploring large language models (LLMs) because this contextual data is what enables LLMs to change from general-purpose to domain-specific knowledge. In the generative AI or traditional AI development cycle, data ingestion serves as the entry point. Here, raw data that is tailored to a company’s requirements can be gathered, preprocessed, masked and transformed into a format suitable for LLMs or other models. Currently, no standardized process exists for overcoming data ingestion’s challenges, but the model’s accuracy depends on it.
4 risks of poorly ingested data
- Misinformation generation: When an LLM is trained on contaminated data (data that contains errors or inaccuracies), it can generate incorrect answers, leading to flawed decision-making and potential cascading issues.
- Increased variance: Variance measures consistency. Insufficient data can lead to varying answers over time, or misleading outliers, particularly impacting smaller data sets. High variance in a model may indicate the model works with training data but be inadequate for real-world industry use cases.
- Limited data scope and non-representative answers: When data sources are restrictive, homogeneous or contain mistaken duplicates, statistical errors like sampling bias can skew all results. This may cause the model to exclude entire areas, departments, demographics, industries or sources from the conversation.
- Challenges in rectifying biased data: If the data is biased from the beginning, “the only way to retroactively remove a portion of that data is by retraining the algorithm from scratch.” It is difficult for LLM models to unlearn answers that are derived from unrepresentative or contaminated data when it’s been vectorized. These models tend to reinforce their understanding based on previously assimilated answers.
Data ingestion must be done properly from the start, as mishandling it can lead to a host of new issues. The groundwork of training data in an AI model is comparable to piloting an airplane. If the takeoff angle is a single degree off, you might land on an entirely new continent than expected.
The entire generative AI pipeline hinges on the data pipelines that empower it, making it imperative to take the correct precautions.
4 key components to ensure reliable data ingestion
- Data quality and governance: Data quality means ensuring the security of data sources, maintaining holistic data and providing clear metadata. This may also entail working with new data through methods like web scraping or uploading. Data governance is an ongoing process in the data lifecycle to help ensure compliance with laws and company best practices.
- Data integration: These tools enable companies to combine disparate data sources into one secure location. A popular method is extract, load, transform (ELT). In an ELT system, data sets are selected from siloed warehouses, transformed and then loaded into source or target data pools. ELT tools such as IBM® DataStage® facilitate fast and secure transformations through parallel processing engines. In 2023, the average enterprise receives hundreds of disparate data streams, making efficient and accurate data transformations crucial for traditional and new AI model development.
- Data cleaning and preprocessing: This includes formatting data to meet specific LLM training requirements, orchestration tools or data types. Text data can be chunked or tokenized while imaging data can be stored as embeddings. Comprehensive transformations can be carried out using data integration tools. Also, there may be a need to directly manipulate raw data by deleting duplicates or changing data types.
- Data storage: After data is cleaned and processed, the challenge of data storage arises. Most data is hosted either on cloud or on-premises, requiring companies to make decisions about where to store their data. It’s important to caution using external LLMs for handling sensitive information such as personal data, internal documents or customer data. However, LLMs play a critical role in fine-tuning or implementing a retrieval-augmented generation (RAG) based- approach. To mitigate risks, it’s important to run as many data integration processes as possible on internal servers. One potential solution is to use remote runtime options like .
Start your data ingestion with IBM
IBM DataStage streamlines data integration by combining various tools, allowing you to effortlessly pull, organize, transform and store data that is needed for AI training models in a hybrid cloud environment. Data practitioners of all skill levels can engage with the tool by using no-code GUIs or access APIs with guided custom code.
The new DataStage as a Service Anywhere remote runtime option provides flexibility to run your data transformations. It empowers you to use the parallel engine from anywhere, giving you unprecedented control over its location. DataStage as a Service Anywhere manifests as a lightweight container, allowing you to run all data transformation capabilities in any environment. This allows you to avoid many of the pitfalls of poor data ingestion as you run data integration, cleaning and preprocessing within your virtual private cloud. With DataStage, you maintain complete control over security, data quality and efficacy, addressing all your data needs for generative AI initiatives.
While there are virtually no limits to what can be achieved with generative AI, there are limits on the data a model uses—and that data may as well make all the difference.
Try DataStage with the data integration trial
More from Artificial intelligence
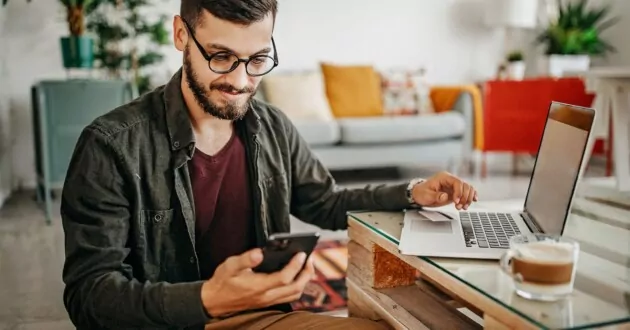
January 3, 2024
IBM’s new watsonx large speech model brings generative AI to the phone
3 min read – Most everyone has heard of large language models, or LLMs, since generative AI has entered our daily lexicon through its amazing text and image generating capabilities, and its promise as a revolution in how enterprises handle core business functions. Now, more than ever, the thought of talking to AI through a chat interface or have it perform specific tasks for you, is a tangible reality. Enormous strides are taking place to adopt this technology to positively impact daily experiences as individuals and…
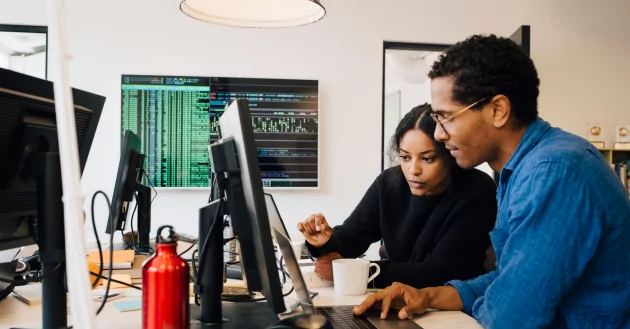
December 20, 2023
Five machine learning types to know
5 min read – Machine learning (ML) technologies can drive decision-making in virtually all industries, from healthcare to human resources to finance and in myriad use cases, like computer vision, large language models (LLMs), speech recognition, self-driving cars and more. However, the growing influence of ML isn’t without complications. The validation and training datasets that undergird ML technology are often aggregated by human beings, and humans are susceptible to bias and prone to error. Even in cases where an ML model isn’t itself biased…
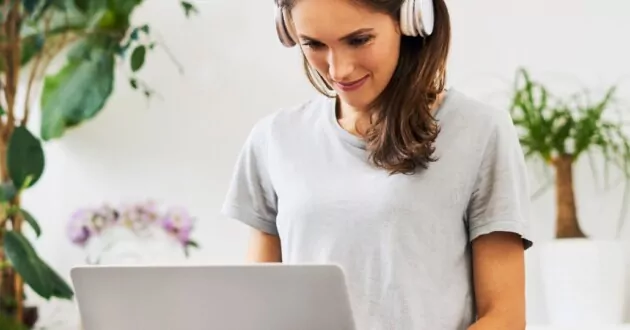
December 15, 2023
Customer service trends winning organizations need to follow
4 min read – Paying attention to the latest customer service trends ensures that an organization is prepared to meet changing customer expectations. Customer loyalty is waning, spurred on by the COVID-19 pandemic, social influences and the ease of switching brands. More than ever, organizations must stay on top of changes in the customer service experience to improve customer satisfaction and meet increased customer needs. A 2023 Gartner study found that 58% of leaders identified business growth as one of their most important goals.…
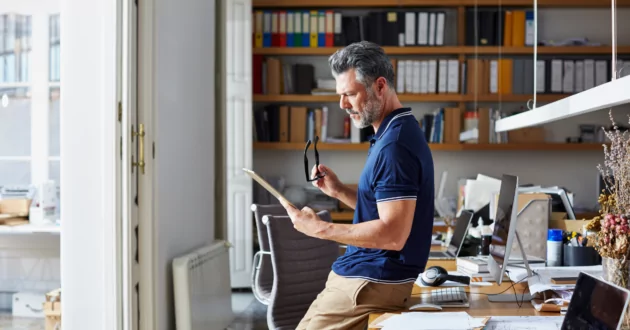
December 15, 2023
Five open-source AI tools to know
5 min read – Open-source artificial intelligence (AI) refers to AI technologies where the source code is freely available for anyone to use, modify and distribute. When AI algorithms, pre-trained models, and data sets are available for public use and experimentation, creative AI applications emerge as a community of volunteer enthusiasts builds upon existing work and accelerates the development of practical AI solutions. As a result, these technologies quite often lead to the best tools to handle complex challenges across many enterprise use cases.…
IBM Newsletters
Get our newsletters and topic updates that deliver the latest thought leadership and insights on emerging trends.
Subscribe now
More newsletters
- SEO Powered Content & PR Distribution. Get Amplified Today.
- PlatoData.Network Vertical Generative Ai. Empower Yourself. Access Here.
- PlatoAiStream. Web3 Intelligence. Knowledge Amplified. Access Here.
- PlatoESG. Carbon, CleanTech, Energy, Environment, Solar, Waste Management. Access Here.
- PlatoHealth. Biotech and Clinical Trials Intelligence. Access Here.
- Source: https://www.ibm.com/blog/the-importance-of-data-ingestion-and-integration-for-enterprise-ai/