By Mason Lender
Using statistics to predict the success of a venture is not novel. Current work and research within the VC space looks into predicting the success of startups and analyzing which attributes are most important in differentiating exceptionally successful founders.
Many of these efforts employ machine learning, deep learning and other statistical models.
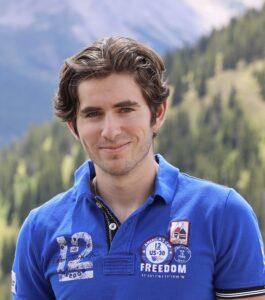
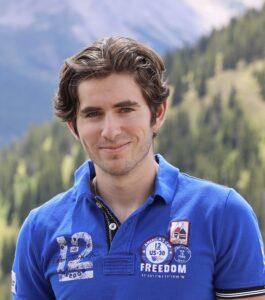
Well-known venture firms such as Correlation Ventures, GV and Ulu Ventures also employ their own models, often using proprietary data to predict the outcome of a venture to better inform their investing decisions.
But while much research has focused on investment decisions, little has been directed at studying the venture capitalists themselves, specifically: What makes a successful venture capitalist?
In the fall of 2000, a team of three authors published a paper titled “What Makes a Successful Venture Capitalist?” attempting to answer that very question. The authors started by conducting a survey of 145 VCs aiming to illuminate any characteristics or circumstances that may lead to success within venture capital.
They probed VCs about their skill sets, experiences and reasons for joining the industry. Among other findings, they discovered that although an MBA or another technical degree “is very helpful, it is not required for success” in the VC industry.
They also noted that “soft skills are valued more highly than quantitative skills” such as accounting or finance and that overall intelligence is cited as “very important.”
To explore if these findings are valid today, and to see how the industry might have changed in the 20 years since this research was originally done, I wrote my thesis while at Yale University looking quantitatively at which factors make a good VC.
For my search, I used Crunchbase and its rich database of private company information, investment insights and personal data. Here’s what I found.
First, what is ‘success’ in VC?
Before creating a model to analyze and predict successful venture capitalists, I needed to define a successful venture capitalist. A common notion in the VC industry is that venture funds are driven by outlier investments; a single excellent investment can have extreme returns of 100x or even 1,000x.
A really successful investor, therefore, will have the ability to uncover these excellent investments. One measure of an incredible investment is one in a unicorn company, or a private company whose value is or exceeds $1 billion. Any venture investment that becomes a unicorn, is thus, an extremely successful investment.
For this analysis, I classified excellent investments as investments in unicorn ventures with current values exceeding $1 billion, since Crunchbase data does not include multiple on invested capital, or MOIC, or other sufficient metrics for categorizing return on investment.
I also used two other datasets. One was CB Insights, which contains information on privately owned unicorn companies.
A different, yet comparable, metric to value public companies is market cap, or the total value of the entirety of a company’s shares of stock. This metric can help in the comparison of the relative size of one company to another. To obtain the total market cap of ventures in the Crunchbase dataset, I used CompaniesMarketCap.
Although unicorn companies are all private, my analysis was agnostic to private or public ventures, categorizing any venture whose value exceeds $1 billion as a “unicorn investment.”
To be a successful venture capitalist, a person must be a good steward of the capital they manage.
In most cases, venture capital firms receive their funding from limited partners. Limited partners select funds they believe align with their investment philosophy and will yield them a high return.
A partner at a venture firm, the highest governing position within the firm, must handle their funds carefully, conducting due diligence on every investment decision to increase the odds of a better return.
Venture partners have a vested interest in the success of their investments. Successful investments and funds are beneficial to partners. It helps the reputation of the investors and the firm, allows for easier raising of future capital from limited partners, and partners receive a carry on the return of the funds and the investments. A successful investor is one who yields a high return on investments. One common way of measuring success is looking at an investor’s MOIC, which measures the performance of an investment or investor. It compares the value of an investment relative to its initial cost.
This metric is often used in private markets, including private equity and venture capital. Calculating an investor’s or fund’s MOIC is done by dividing the total value of investments (realized and unrealized) by the initial investment. MOIC does not capture or produce a time-weighted measurement of success, however. Unfortunately, this metric is not publicly available.
Generating a model to predict success
After cleaning, exploring and trimming the Crunchbase dataset, I fitted a logistic regression model. Just as VCs look to predict and make outlier investments, this study looked to predict outlier venture capitalists. I created several different models looking at how the two factors — education and career — affect success as a venture capitalist.
In each model, the outcome variable is binary — whether or not an investor would make a unicorn investment.
The specific coefficients of the model are discussed below, but, in general, a positive coefficient for a predictor variable indicates that increasing that predictor (or a certain level of that predictor) is associated with an increased log odds of an investment being a unicorn investment (increased probability).
For example, a positive coefficient for having attended a top 25 university indicates an increased log odds ratio for an investment being a unicorn, while a negative coefficient for the founder indicator predictor means a decrease in the predicted log odds ratio of a unicorn investment.
Model 1: Education
The plot above displays the coefficients of the education-related predictors including subject studied, an indicator if the individual completed their degree, and indicator for having attended a top 25 university.
The coefficients are ordered from top to bottom by greatest to least predicted positive impact on the log odds ratio of a unicorn investment. The long blue bands that span each coefficient point represent the 95% confidence interval for the predicted coefficient. All coefficients whose bands do not touch 0 are considered to be statistically significant at the alpha level of 0.05.
What does this model mean in layman’s terms?
When looking at the subject coefficients, it appears that computer science, law and business subjects have the highest coefficients. Thus, the logistic regression model predicts that holding all else equal, for an investment with attribution to an investor who has studied one of those fields would have a significantly positive effect on the log odds ratio of the investment being a unicorn when compared to the baseline category of an arts subject studied.
The following hypothetical may better illustrate this concept. Suppose an investor studied computer science for their undergraduate degree at Yale. If this individual were to make some investment, the following calculation could be used to calculate the log odds of the a unicorn investment:
In the example above, obtaining a degree in computer science at a top 25 university increases the log odds (probability) of an outlier investor (one having made a unicorn investment).
What career tells us about VC success
How should one value an investor’s previous jobs or career choices when predicting their success?
In the second model, I look to answer this question. The subsequent plot interpretation is very similar to that with the education coefficient plot, but below career related variables are plotted instead. Here the plot shows the coefficients of the career related predictors including the prior job of an investor, as well as an indicator if the individual is a past founder.
Once again, coefficients are ordered from top to bottom by greatest (increase in probability) of a unicorn investment to least predicted positive effect.
In this model, two types of prior jobs appear to be statistically significant: investment bankers and management consultants. The baseline category for prior jobs is set to working at a big five investment bank: JPMorgan Chase, Goldman Sachs, BofA Securities, Morgan Stanley and Citigroup.
Model 2: Career
Each beta coefficient corresponds to the difference in log odds ratio/probability of an investment being a unicorn investment from this baseline category.
Founders aren’t necessarily better investors
One interesting outcome of this model is the effect of the founder coefficient. This model predicts that if an investment partner was a previous founder, the predicted probability or log odds of that investment being a unicorn actually decreases.
This is an interesting phenomenon as, intuitively, one might think that a founder would be a better judge of finding unicorn founders.
However, being a founder might in fact do the opposite. This result, however, contradicts Brett Rhyne’s study, which finds that VCs who were once founders of successful startups have higher success rates on their investments when compared to nonfounder VCs.
The model above does not consider the VC founder’s success, but rather if they have been a founder previously. The differing results might be explained by some unsuccessful founders being poor VCs, but more analysis is needed.
What does all of this mean?
Why do all this? And what does this all mean? Just as many VCs conduct analysis and due diligence on a founder and startup before making an investment, this exercise might help limited partners or VCs hire better investors.
Based on the analysis of this project and the models made, LPs might look at further important factors or predictors highlighted in the models above.
When selecting investors to fund, or when VC firms hire or promote partners, similar analysis might prove to be fruitful for better returns. With the limitations of the Crunchbase data, VC firms should not change their hiring strategy and LPs should not change the allocation of funds.
Rather, this project might highlight new avenues of research to be conducted with cleaner, more accurate data. In an industry where passing on an investment could cost billions in returns, selecting the right individual for the job is of the utmost importance.
Based on the analysis and model, venture capital firms and LPs should look into the subjects studied, prior jobs held and education institutions attended by VCs to better inform their allocation of funds.
Further research might look at or include other factors including age, years of experience within a job, or possibly investing style.
Mason Lender is a recent graduate from Yale University with a double major in statistics and data science and global affairs. He is currently building a company with Entrepreneur First in New York. Previously, he worked for Google, McKinsey & Co. and with startups.
Methodology: A note on data
I would like to thank Brian Macdonald, Ph.D., Department of Statistics & Data Science at Yale University, and Jorge Torres, J.D., lecturer, Yale Engineering & Entrepreneurship fellow, Tsai Center for Innovative Thinking at Yale. Their support and guidance throughout this project made it possible. — Mason Lender
I began with data cleaning, exploration and visualization of the Crunchbase dataset. I started by narrowing my focus by mapping out the Crunchbase database as follows:
The Crunchbase dataset is split up by category into multiple spreadsheets with related unique keys for mapping.
It is a large relational database with information on investors (individuals, firms and their funds), ventures (organizations/companies and sub-organizations), and people (investors and founders).
We can visualize the linkage between each csv with the lines connecting their keys above. For my study, I mainly relied on the investor_partners.csv.
This file contains every investment stored in Crunchbase’s dataset that has attribution, or is credited to, an investment partner. These investment partners are the partners at venture capital firms that make the final decisions on investments. Supplementing the data stored within the investor_partners.csv, degrees.csv and people_descriptions.csv were leveraged to add more fields and predictors for my analysis.
Illustration: Dom Guzman
Search less. Close more.
Grow your revenue with all-in-one prospecting solutions powered by the leader in private-company data.
Stay up to date with recent funding rounds, acquisitions, and more with the
Crunchbase Daily.
- SEO Powered Content & PR Distribution. Get Amplified Today.
- PlatoData.Network Vertical Generative Ai. Empower Yourself. Access Here.
- PlatoAiStream. Web3 Intelligence. Knowledge Amplified. Access Here.
- PlatoESG. Carbon, CleanTech, Energy, Environment, Solar, Waste Management. Access Here.
- PlatoHealth. Biotech and Clinical Trials Intelligence. Access Here.
- Source: https://news.crunchbase.com/venture/vc-success-prediction-crunchbase-data-mason-lender/