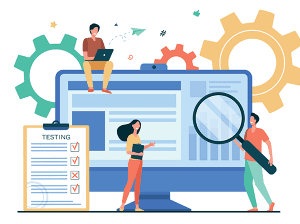
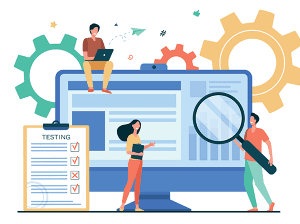
Data Quality (DQ), the degree of business and consumer confidence in data’s usefulness, remains a sticking point to getting business insights and managing risk. According to recent research, it takes an average of 15 hours to resolve a given Data Quality issue or incident, and in 2024 and beyond, 25% or more of revenue will be subjected to DQ issues.
In response to these challenges, over 50% of organizations plan to implement AI and automation solutions, including data enrichment. Data enrichment describes the processes and activities that augment existing data sets to improve Data Quality and generate more profound insights.
Enrichment continues to be a popular request, as demonstrated by more than 19 in every 20 customer experience (CX) leaders investing in it or planning to do so. Additionally, companies find that a 10% improvement in customer lead quality through data enrichment can result in a 40% sales revenue increase.
Yet, data enhancement is not a magic bullet that can solve all Data Quality problems. To truly get Data Quality benefits, businesspeople need to understand the distinctions between Data Quality vs. data enrichment, how they complement each other, and how to enrich data as a Data Quality tactic.
Understanding Data Quality with Consistency
When asked to define Data Quality, businesspeople typically describe having data they can use to complete and succeed with work objectives. However, each person may interpret good DQ slightly differently, depending on the issues and requirements they see and when the question is posed.
Part of this confusion can be explained by how DQ can be assessed. Business units measure acceptable data on various dimensions, including accuracy, completeness, consistency, integrity, uniqueness, and validity. Each team may use a different combination of DQ indicators for various attributes or weight them differently.
Moreover, what constitutes a good objective DQ may not synch up across the firm, especially if the business and Data Strategy are unclear. No wonder a company can easily have salespeople making 85% of embarrassing mistakes because of inaccurate customer data and duplicate records across systems with conflicting information.
Therefore, it is crucial for each enterprise to get a consistent understanding of DQ, especially where any external data source likely has its unique DQ definition. As William McKnight, an advisor to many of the world’s best-known organizations, states in his webinar, DQ improvements happen when people know what cleaner data looks like and take action to get there. This consistent DQ description is pivotal in knowing whether and when to bolster available data.
Understanding Data Enrichment
Data enrichment is a Data Quality tactic encompassing integration processes and actions needed to augment existing datasets. Corporations enhance their data to fill in missing gaps, grasp contextual nuances, and add additional details to describe an entity – commonly a prospective or active customer. For any objective, organizations have the choice of what data to bolster.
Businesses adopt different kinds of data enrichment that target critical attributes and provide insights aligned with business objectives. Examples of enrichment types include:
Typically, data enrichment agrees with other business Data Quality tactics. During this process, IT or the technology team standardizes third-party data or outside data formatting before integration.
This process involves extracting and transforming the external data so the systems receiving it can read and understand it. As a last step of the Extract, Transform, and Load (ETL), the application adds the outside data into the internal systems, appending the current data.
How Data Enrichment Works with Data Quality
The effectiveness of data enrichment may or may not impact Data Quality. Results depend on various factors, such as DQ objectives, the data set added, how that data is integrated, and the caliber of the data set before the enrichment.
To illustrate this point, take an example created with the help of Chat GPT. A company starts the process with its marketing department records, as shown below:


To improve Data Quality for an upcoming campaign, the company’s manager purchases third-party data to fill in missing emails and gather additional details about age. Nothing further is done to the organization’s existing data. Upon completion of data enrichment, IT forwards the updated customer records to marketing for their effort.


While marketers can target customers over 40, the enriched data proves challenging to use. Is Mr. Johnson’s first name Mike? Has Sarah Miller’s email been updated accurately from sarah.miller@verizon.com to the address with email.com? What if the marketers need a corporate email address instead of a personal one to comply with data protection laws?
Marketers or IT may manually complete the data or use a Data Quality tool to fix discrepancies. But getting a corporate email address would prove especially time-consuming to add retroactively.
In this instance, data enrichment did not impact or decrease Data Quality. However, it costs more for the company to sort out the results. When scaled across thousands of records, the consequences of this tactic lead to a data mess.
Implementing a Robust Data Quality Program
The example above shows why companies need to implement a solid Data Quality program before enrichment. Data stewards are at the heart of this effort as they are designated to manage and keep their datasets in good shape, according to a consistent DQ definition.
Data stewards operate in a DQ program through accountable Data Governance, a service that formalizes and harmonizes data roles, communications, processes, and measurements across company teams. Accountable Data Governance guides the Data Quality program and supports its purpose, essential components, and DQ tactics, including data enrichment.
For instance, consider the marketing team in the example above. Through Data Governance services, the team could designate a data steward from the marketing team, update the company, and automate data cleaning processes. That way, before starting any enrichment process, the company could flag and resolve entries missing critical details, such as a first name and an email.
Since reaching a consistent DQ definition is iterative, companies must regularly monitor and correct DQ to reduce risks from inaccurate data, ensuring enough trust and integrity in their data before adding more records. Especially as companies collect streaming or real-time data, they must assess and clean data routinely.
Moreover, having a DQ program serviced by accountable Data Governance minimizes risks of violating data regulations, like the GDPR. For example, integrating additional demographic data from other sources with European customers without explicit consent can lead to significant fines. Data transfers from one source to another are subject to legal protections, making a DQ program with Data Government services imperative before enrichment.
Applying Data Enrichment to Enhance Data Quality
Once an organization has established a robust Data Quality program and achieved sufficient progress in owning adequate data assets, even if small and targeted, it should consider data enrichment for further DQ improvement. This tactic contributes to improved DQ through enhanced granularity when executed well. Examples include the following:
- Vevo implemented targeted ads and market segmentation across 35 Connected TV (CTV) platforms using AnalyticsIQ identity data.
- A theme park increased its attendance and revenue during the COVID-19 pandemic by driving 15% higher conversions, 117% higher landing page views, and a 62% lower cost per landing page view.
- The United Nations adopted a 15-year sustainable plan, including Sustainable Development Goals (SDGs), and improved Data Quality around performance indicators so that they could help other countries make evidence-based strategic decisions.
- Insurance agencies leveraged geospatial enrichment, adding location information to their customers. This practice enabled a more comprehensive assessment of property risks and made insurance rates more realistic.
- American Express significantly reduced fraudulent transactions by 60% through data enrichment. The company bolstered its data with context by adding IP addresses, email addresses, and shipping addresses provided by the merchants. American Express cross-referenced this enhanced data with information stored in its systems, demonstrating a successful use case in fraud prevention.
Conclusion
As the use cases above show, data enrichment can work to improve Data Quality. When this happens, organizations see significant and visible improvement toward their business goals with more accurate pictures of their customers, performance goals, and assets.
However, these outcomes require corporations to work to establish a DQ program with accountable Data Governance support. That way, organizations know the best tactics to make DQ acceptable and do them at the appropriate time. Once enterprises connect their DQ tactics with the available resources allocated for their DQ program, they should consider data enrichment to power decisions and open up business opportunities.
- SEO Powered Content & PR Distribution. Get Amplified Today.
- PlatoData.Network Vertical Generative Ai. Empower Yourself. Access Here.
- PlatoAiStream. Web3 Intelligence. Knowledge Amplified. Access Here.
- PlatoESG. Carbon, CleanTech, Energy, Environment, Solar, Waste Management. Access Here.
- PlatoHealth. Biotech and Clinical Trials Intelligence. Access Here.
- Source: https://www.dataversity.net/data-quality-vs-data-enrichment/