本日、Mistral AI が開発した Mistral 7B 基礎モデルが、以下を通じてお客様に提供されることを発表できることを嬉しく思います。 Amazon SageMaker ジャンプスタート ワンクリックでデプロイして推論を実行できます。 7 億のパラメータを備えた Mistral 7B は、簡単にカスタマイズして迅速に導入できます。 このモデルは、アルゴリズムとモデルへのアクセスを提供する機械学習 (ML) ハブである SageMaker JumpStart を使用して試すことができ、ML をすぐに始めることができます。 この投稿では、Mistral 7B モデルを検出してデプロイする方法を説明します。
ミストラル7Bとは
Mistral 7B は、Mistral AI によって開発された基盤モデルで、英語のテキストとコード生成機能をサポートします。 テキストの要約、分類、テキスト補完、コード補完など、さまざまなユースケースをサポートします。 モデルの簡単なカスタマイズ性を実証するために、Mistral AI は、公開されているさまざまな会話データセットを使用して微調整された、チャットのユースケース向けの Mistral 7B Instruct モデルもリリースしました。
Mistral 7B はトランスフォーマー モデルであり、グループ化されたクエリ アテンションとスライディング ウィンドウ アテンションを使用して、より高速な推論 (低遅延) を実現し、より長いシーケンスを処理します。 グループ クエリ アテンションは、マルチクエリとマルチヘッド アテンションを組み合わせて、マルチヘッド アテンションに近い出力品質とマルチクエリ アテンションと同等の速度を実現するアーキテクチャです。 スライディング ウィンドウ アテンションでは、トランスフォーマーの積み重ねられたレイヤーを使用して、ウィンドウ サイズを超えて過去にアテンションし、コンテキストの長さを増やします。 Mistral 7B は 8,000 トークンのコンテキスト長を持ち、低レイテンシと高スループットを示し、より大きなモデルの代替品と比較した場合に強力なパフォーマンスを示し、7B モデル サイズでメモリ要件を低く抑えます。 モデルは許容条件下で利用可能です Apache 2.0ライセンス、制限なく使用できます。
SageMaker JumpStart とは
SageMaker JumpStart を使用すると、ML 実践者は、増え続ける最高のパフォーマンスの基盤モデルのリストから選択できます。 ML 実践者は基礎モデルを専用のモデルにデプロイできます。 アマゾンセージメーカー ネットワーク分離環境内のインスタンスを構築し、モデルのトレーニングとデプロイメントに SageMaker を使用してモデルをカスタマイズします。
数回クリックするだけで、Mistral 7B を検出して展開できるようになりました。 Amazon SageMakerスタジオ または、SageMaker Python SDK を介してプログラム的に、次のような SageMaker 機能を使用してモデルのパフォーマンスと MLOps コントロールを導き出すことができます。 AmazonSageMakerパイプライン, Amazon SageMakerデバッガ、またはコンテナーのログ。 モデルは AWS の安全な環境にデプロイされ、VPC の制御下に置かれるため、データのセキュリティが確保されます。
モデルを発見する
Mistral 7B 基盤モデルには、SageMaker Studio UI の SageMaker JumpStart および SageMaker Python SDK を通じてアクセスできます。 このセクションでは、SageMaker Studio でモデルを検出する方法について説明します。
SageMaker Studio は、単一の Web ベースのビジュアル インターフェイスを提供する統合開発環境 (IDE) であり、専用ツールにアクセスして、データの準備から ML モデルの構築、トレーニング、デプロイまで、すべての ML 開発ステップを実行できます。 SageMaker Studio の開始方法とセットアップ方法の詳細については、以下を参照してください。 Amazon SageMakerスタジオ.
SageMaker Studio では、事前トレーニングされたモデル、ノートブック、事前構築されたソリューションを含む SageMaker JumpStart にアクセスできます。 事前に構築された自動化されたソリューション.

SageMaker JumpStart ランディング ページから、ソリューション、モデル、ノートブック、その他のリソースを参照できます。 ミストラル 7B は、 基礎モデル: テキスト生成 カルーセル

を選択して、他のモデル バリエーションを見つけることもできます。 すべてのテキスト モデルを探索する または「ミストラル」で検索してください。

モデル カードを選択すると、ライセンス、トレーニングに使用されるデータ、使用方法などのモデルに関する詳細が表示されます。 ボタンも XNUMX つあります。 配備します & ノートを開くこれは、モデルの使用に役立ちます (次のスクリーンショットは、 配備します オプション)。
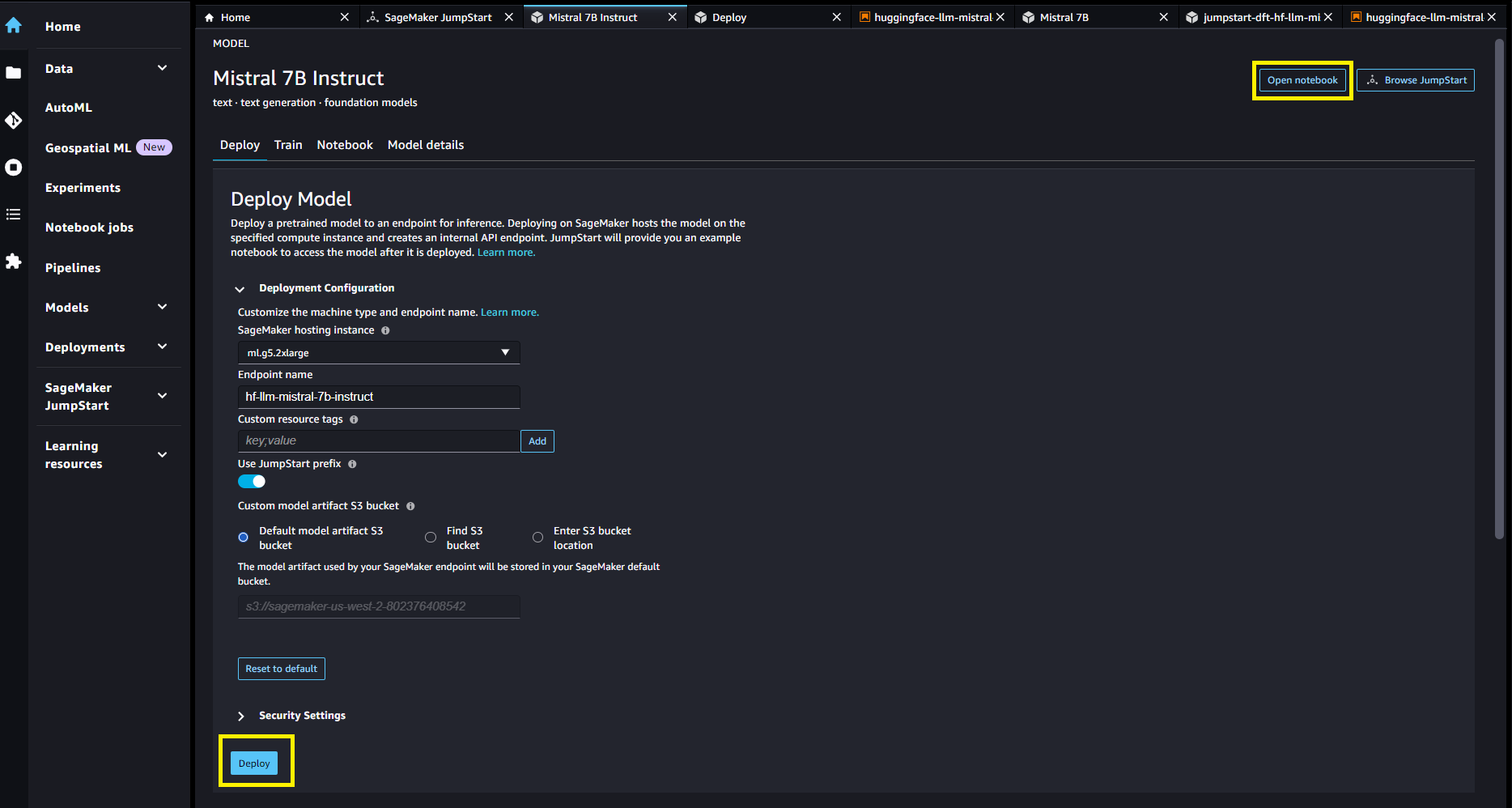
モデルを展開する
選択すると展開が開始されます 配備します。 または、選択したときに表示されるサンプル ノートブックを通じてデプロイすることもできます。 ノートを開く。 サンプル ノートブックは、推論用のモデルをデプロイし、リソースをクリーンアップする方法に関するエンドツーエンドのガイダンスを提供します。
ノートブックを使用して展開するには、まず、 model_id
。 次のコードを使用して、選択したモデルのいずれかを SageMaker にデプロイできます。
from sagemaker.jumpstart.model import JumpStartModel model = JumpStartModel(model_id="huggingface-llm-mistral-7b-instruct")
predictor = model.deploy()
これにより、デフォルトのインスタンス タイプ (ml.g5.2xlarge) およびデフォルトの VPC 構成を含むデフォルト構成でモデルが SageMaker にデプロイされます。 これらの構成は、デフォルト以外の値を指定することで変更できます。 ジャンプスタートモデル。 デプロイ後、SageMaker プレディクターを介して、デプロイされたエンドポイントに対して推論を実行できます。
payload = {"inputs": "<s>[INST] Hello! [/INST]"}
predictor.predict(payload)
導入構成の最適化
Mistral モデルは、Text Generation Inference (TGI バージョン 1.1) モデル提供を使用します。 TGI 深層学習コンテナ (DLC) を使用してモデルをデプロイする場合、さまざまな設定を行うことができます。 ランチャーの引数 エンドポイントをデプロイするときに環境変数経由で。 Mistral 8,000B モデルの 7 トークンのコンテキスト長をサポートするために、SageMaker JumpStart はデフォルトで次のパラメータの一部を設定しています。 MAX_INPUT_LENGTH
& MAX_TOTAL_TOKENS
それぞれ8191と8192に。 モデル オブジェクトを検査すると、完全なリストを表示できます。
デフォルトでは、SageMaker JumpStart は環境変数を介して同時ユーザーをクランプしません。 MAX_CONCURRENT_REQUESTS
これは、TGI のデフォルト値である 128 よりも小さいです。その理由は、一部のユーザーがペイロード コンテキスト長が短い一般的なワークロードを使用しており、高い同時実行性を必要とする可能性があるためです。 SageMaker TGI DLC は、ローリング バッチを通じて複数の同時ユーザーをサポートしていることに注意してください。 アプリケーションのエンドポイントをデプロイするときは、クランプする必要があるかどうかを検討することがあります。 MAX_TOTAL_TOKENS
or MAX_CONCURRENT_REQUESTS
ワークロードに最高のパフォーマンスを提供するには、展開前に次のことを行います。
model.env["MAX_CONCURRENT_REQUESTS"] = "4"
ここでは、典型的なエンドポイント ワークロードに対してモデルのパフォーマンスがどのように異なるかを示します。 次の表では、小規模なクエリ (128 個の入力ワードと 128 個の出力トークン) が多数の同時ユーザーの下で非常にパフォーマンスが高く、1,000 秒あたり 512 トークン程度のトークン スループットに達していることがわかります。 ただし、入力ワード数が 16 入力ワードに増加すると、エンドポイントはバッチ処理能力 (同時に処理できる同時リクエストの数) を飽和させ、その結果、同時ユーザー数が 6,400 人あたりからスループットが頭打ちになり、レイテンシが大幅に低下します。 最後に、複数の同時ユーザーが同時に大規模な入力コンテキスト (たとえば、60 ワード) を使用してエンドポイントにクエリを実行すると、このスループットのプラトーは比較的早く発生し、SageMaker アカウントが過負荷のリクエストに対して XNUMX 秒の応答タイムアウト制限に遭遇し始める点に達します。 。
. |
スループット (トークン/秒) |
同時ユーザー |
1 |
2 |
4 |
8 |
16 |
32 |
64 |
128 |
|
インスタンスタイプ |
単語を入力する |
出力トークン |
. |
ミストラル-7b-命令 |
ml.g5.2xラージ |
128 |
128 |
30 |
54 |
89 |
166 |
287 |
499 |
793 |
1030 |
512 |
128 |
29 |
50 |
80 |
140 |
210 |
315 |
383 |
458 |
6400 |
128 |
17 |
25 |
30 |
35 |
- |
- |
- |
- |
. |
p50 レイテンシー (ミリ秒/トークン) |
同時ユーザー |
1 |
2 |
4 |
8 |
16 |
32 |
64 |
128 |
|
インスタンスタイプ |
単語を入力する |
出力トークン |
. |
ミストラル-7b-命令 |
ml.g5.2xラージ |
128 |
128 |
32 |
33 |
34 |
36 |
41 |
46 |
59 |
88 |
512 |
128 |
34 |
36 |
39 |
43 |
54 |
71 |
112 |
213 |
6400 |
128 |
57 |
71 |
98 |
154 |
- |
- |
- |
- |
推論とプロンプトの例
ミストラル 7B
標準的なテキスト生成モデルと同様に、基本の Mistral 7B モデルを操作できます。モデルは入力シーケンスを処理し、シーケンス内の予測された次の単語を出力します。 以下は、マルチショット学習を使用した簡単な例です。モデルにはいくつかの例が提供され、これらの前の例のコンテキスト知識を使用して最終的な応答例が生成されます。
> Input
Tweet: "I get sad when my phone battery dies."
Sentiment: Negative
###
Tweet: "My day has been :+1:"
Sentiment: Positive
###
Tweet: "This is the link to the article"
Sentiment: Neutral
###
Tweet: "This new music video was incredibile"
Sentiment: > Output Positive
ミストラル7Bの指示
命令調整バージョンの Mistral は、会話の役割がユーザー プロンプトで始まり、ユーザーとアシスタントの間で交互に行われる形式の命令を受け入れます。 単純なユーザー プロンプトは次のようになります。
<s>[INST] {user_prompt} [/INST]
複数ターンのプロンプトは次のようになります。
<s>[INST] {user_prompt_1} [/INST] {assistant_response_1} </s><s>[INST] {user_prompt_1} [/INST]
このパターンは、会話のターン数に関係なく繰り返されます。
次のセクションでは、Mistral 7B Instruct モデルを使用したいくつかの例を検討します。
知識検索
以下はナレッジ検索の例です。
> Input
<s>[INST] Which country has the most natural lakes? Answer with only the country name. [/INST] > Output
1. Canada
大規模なコンテキストに関する質問への回答
このモデルを使用して長い入力コンテキスト長をサポートする方法を示すために、次の例には Robert Sullivan の「Rats」というタイトルの一節が埋め込まれています (参照)、MCAS グレード 10 の英語芸術読解テストから入力プロンプト命令に組み込まれ、モデルにテキストに関する指示された質問をします。
> Input
<s>[INST] A rat is a rodent, the most common mammal in the world. Rattus norvegicus is one of the approximately four hundred different kinds of rodents, and it is known by many names, each of which describes a trait or a perceived trait or sometimes a habitat: the earth rat, the roving rat, the barn rat, the fi eld rat, the migratory rat, the house rat, the sewer rat, the water rat, the wharf rat, the alley rat, the gray rat, the brown rat, and the common rat. The average brown rat is large and stocky; it grows to be approximately sixteen inches long from its nose to its tail—the size of a large adult human male’s foot—and weighs about a pound, though brown rats have been measured by scientists and exterminators at twenty inches and up to two pounds. The brown rat is sometimes confused with the black rat, or Rattus rattus, which is smaller and once inhabited New York City and all of the cities of America but, since Rattus norvegicus pushed it out, is now relegated to a minor role. (The two species still survive alongside each other in some Southern coastal cities and on the West Coast, in places like Los Angeles, for example, where the black rat lives in attics and palm trees.) The black rat is always a very dark gray, almost black, and the brown rat is gray or brown, with a belly that can be light gray, yellow, or even a pure-seeming white. One spring, beneath the Brooklyn Bridge, I saw a red-haired brown rat that had been run over by a car. Both pet rats and laboratory rats are Rattus norvegicus, but they are not wild and therefore, I would emphasize, not the subject of this book. Sometimes pet rats are called fancy rats. But if anyone has picked up this book to learn about fancy rats, then they should put this book down right away; none of the rats mentioned herein are at all fancy. Rats are nocturnal, and out in the night the brown rat’s eyes are small and black and shiny; when a fl ashlight shines into them in the dark, the eyes of a rat light up like the eyes of a deer. Though it forages* in darkness, the brown rat has poor eyesight. It makes up for this with, fi rst of all, an excellent sense of smell. . . . They have an excellent sense of taste, detecting the most minute amounts of poison, down to one part per million. A brown rat has strong feet, the two front paws each equipped with four clawlike nails, the rear paws even longer and stronger. It can run and climb with squirrel-like agility. It is an excellent swimmer, surviving in rivers and bays, in sewer streams and toilet bowls. The brown rat’s teeth are yellow, the front two incisors being especially long and sharp, like buckteeth. When the brown rat bites, its front two teeth spread apart. When it gnaws, a fl ap of skin plugs the space behind its incisors. Hence, when the rat gnaws on indigestible materials—concrete or steel, for example—the shavings don’t go down the rat’s throat and kill it. Its incisors grow at a rate of fi ve inches per year. Rats always gnaw, and no one is certain why—there are few modern rat studies. It is sometimes erroneously stated that the rat gnaws solely to limit the length of its incisors, which would otherwise grow out of its head, but this is not the case: the incisors wear down naturally. In terms of hardness, the brown rat’s teeth are stronger than aluminum, copper, lead, and iron. They are comparable to steel. With the alligator-like structure of their jaws, rats can exert a biting pressure of up to seven thousand pounds per square inch. Rats, like mice, seem to be attracted to wires—to utility wires, computer wires, wires in vehicles, in addition to gas and water pipes. One rat expert theorizes that wires may be attractive to rats because of their resemblance to vines and the stalks of plants; cables are the vines of the city. By one estimate, 26 percent of all electric-cable breaks and 18 percent of all phone-cable disruptions are caused by rats. According to one study, as many as 25 percent of all fi res of unknown origin are rat-caused. Rats chew electrical cables. Sitting in a nest of tattered rags and newspapers, in the fl oorboards of an old tenement, a rat gnaws the head of a match—the lightning in the city forest. When it is not gnawing or feeding on trash, the brown rat digs. Anywhere there is dirt in a city, brown rats are likely to be digging—in parks, in fl owerbeds, in little dirt-poor backyards. They dig holes to enter buildings and to make nests. Rat nests can be in the floorboards of apartments, in the waste-stuffed corners of subway stations, in sewers, or beneath old furniture in basements. “Cluttered and unkempt alleyways in cities provide ideal rat habitat, especially those alleyways associated with food-serving establishments,” writes Robert Corrigan in Rodent Control, a pest control manual. “Alley rats can forage safely within the shadows created by the alleyway, as well as quickly retreat to the safety of cover in these narrow channels.” Often, rats burrow under concrete sidewalk slabs. Entrance to a typical under-the-sidewalk rat’s nest is gained through a two-inch-wide hole—their skeletons collapse and they can squeeze into a hole as small as three quarters of an inch wide, the average width of their skull. This tunnel then travels about a foot down to where it widens into a nest or den. The den is lined with soft debris, often shredded plastic garbage or shopping bags, but sometimes even grasses or plants; some rat nests have been found stuffed with the gnawed shavings of the wood-based, spring-loaded snap traps that are used in attempts to kill them. The back of the den then narrows into a long tunnel that opens up on another hole back on the street. This second hole is called a bolt hole; it is an emergency exit. A bolt hole is typically covered lightly with dirt or trash—camoufl age. Sometimes there are networks of burrows, which can stretch beneath a few concrete squares on a sidewalk, or a number of backyards, or even an entire city block—when Rattus norvegicus fi rst came to Selkirk, England, in 1776, there were so many burrows that people feared the town might sink. Rats can also nest in basements, sewers, manholes, abandoned pipes of any kind, fl oorboards, or any hole or depression. “Often,” Robert Corrigan writes, “‘city rats’ will live unbeknownst to people right beneath their feet.” Rats also inhabit subways, as most people in New York City and any city with a subway system are well aware. Every once in a while, there are reports of rats boarding trains, but for the most part rats stay on the tracks—subway workers I have talked to refer to rats as “track rabbits.” People tend to think that the subways are fi lled with rats, but in fact rats are not everywhere in the system; they live in the subways according to the supply of discarded human food and sewer leaks. Sometimes, rats use the subway purely for nesting purposes; they fi nd ways through the walls of the subway stations leading from the tracks to the restaurants and stores on the street—the vibrations of subway trains tend to create rat-size cracks and holes. Many subway rats tend to live near stations that are themselves near fast-food restaurants. At the various subway stations near Herald Square, for example, people come down from the streets and throw the food that they have not eaten onto the tracks, along with newspapers and soda bottles and, I have noticed, thousands of nolonger-charged AA batteries, waiting to leak acid. The rats eat freely from the waste and sit at the side of the little streams of creamy brown sewery water that fl ows between the rails. They sip the water the way rats do, either with their front paws or by scooping it up with their incisors. What is the most likely reason the author states, "cables are the vines of the city"? [/INST] > Output
1. The author states that rats are attracted to wires, such as utility wires, computer wires, and vehicle wires, which may be due to their resemblance to vines and the stalks of plants.
数学と推理
ミストラル モデルは、数学的精度における強みも報告しています。 Mistral は、次の数学ロジックのような理解を提供します。
> Input
<s>[INST] I bought an ice cream for 6 kids. Each cone was $1.25 and I paid with a $10 bill. How many dollars did I get back? Explain first before answering. [/INST] > Output
1. First, let's figure out how much the ice cream cost in total. Since each cone was $1.25 and there were 6 kids, the total cost would be: Total cost = Number of cones * Cost per cone
Total cost = 6 * $1.25
Total cost = $7.50 2. Next, we'll subtract the total cost from the amount paid ($10) to find out how much money was returned: Money returned = Amount paid - Total cost
Money returned = $10 - $7.50
Money returned = $2.50 So, you got back $2.50 after buying the ice cream for the 6 kids.
コーディング
以下はコーディング プロンプトの例です。
> Input
<s>[INST] In Bash, how do I list all text files in the current directory (excluding subdirectories) that have been modified in the last month? [/INST] > Output
1. Use the `find` command to search for text files in the current directory (excluding subdirectories) that have been modified in the last month. The `-type f` option specifies that we only want to search for files, not directories. The `-name "*.txt"` option specifies that we only want to search for files with the `.txt` extension. The `-mtime -30` option specifies that we only want to search for files that have been modified in the last 30 days (1 month). ```bash
find . -type f -name "*.txt" -mtime -30
``` This command will list all text files in the current directory (excluding subdirectories) that have been modified in the last month.
クリーンアップ
ノートブックの実行が完了したら、請求が停止されるように、プロセス中に作成したすべてのリソースを必ず削除してください。 次のコードを使用します。
predictor.delete_model()
predictor.delete_endpoint()
まとめ
この投稿では、SageMaker Studio で Mistral 7B の使用を開始し、推論用のモデルをデプロイする方法を説明しました。 基礎モデルは事前にトレーニングされているため、トレーニングとインフラストラクチャのコストを削減し、ユースケースに合わせたカスタマイズを可能にすることができます。 訪問 Amazon SageMaker ジャンプスタート 今すぐ始めましょう。
リソース
著者について
カイル・ウルリッヒ博士 Amazon SageMaker JumpStart チームの応用科学者です。 彼の研究対象には、スケーラブルな機械学習アルゴリズム、コンピューター ビジョン、時系列、ベイジアン ノンパラメトリック、ガウス プロセスなどがあります。 彼はデューク大学で博士号を取得しており、NeurIPS、Cell、および Neuron で論文を発表しています。
アシッシュ・ケタン博士 Amazon SageMaker JumpStart の上級応用科学者であり、機械学習アルゴリズムの開発を支援しています。 イリノイ大学アーバナ・シャンペーン校で博士号を取得しました。 彼は機械学習と統計的推論の積極的な研究者であり、NeurIPS、ICML、ICLR、JMLR、ACL、および EMNLP カンファレンスで多くの論文を発表しています。
Vivek Singh Amazon SageMaker JumpStart のプロダクトマネージャーです。 彼は、顧客が SageMaker JumpStart をオンボードして、生成 AI アプリケーションを構築するための ML の取り組みを簡素化および加速できるようにすることに重点を置いています。
ロイ・アレラ は、ドイツのミュンヘンを拠点とする AWS のシニア AI/ML スペシャリスト ソリューション アーキテクトです。 Roy は、小規模なスタートアップから大企業まで、AWS の顧客が AWS 上で大規模な言語モデルを効率的にトレーニングしてデプロイできるよう支援します。 Roy は、計算最適化問題と AI ワークロードのパフォーマンスの向上に情熱を注いでいます。