<!—->
<!– –>
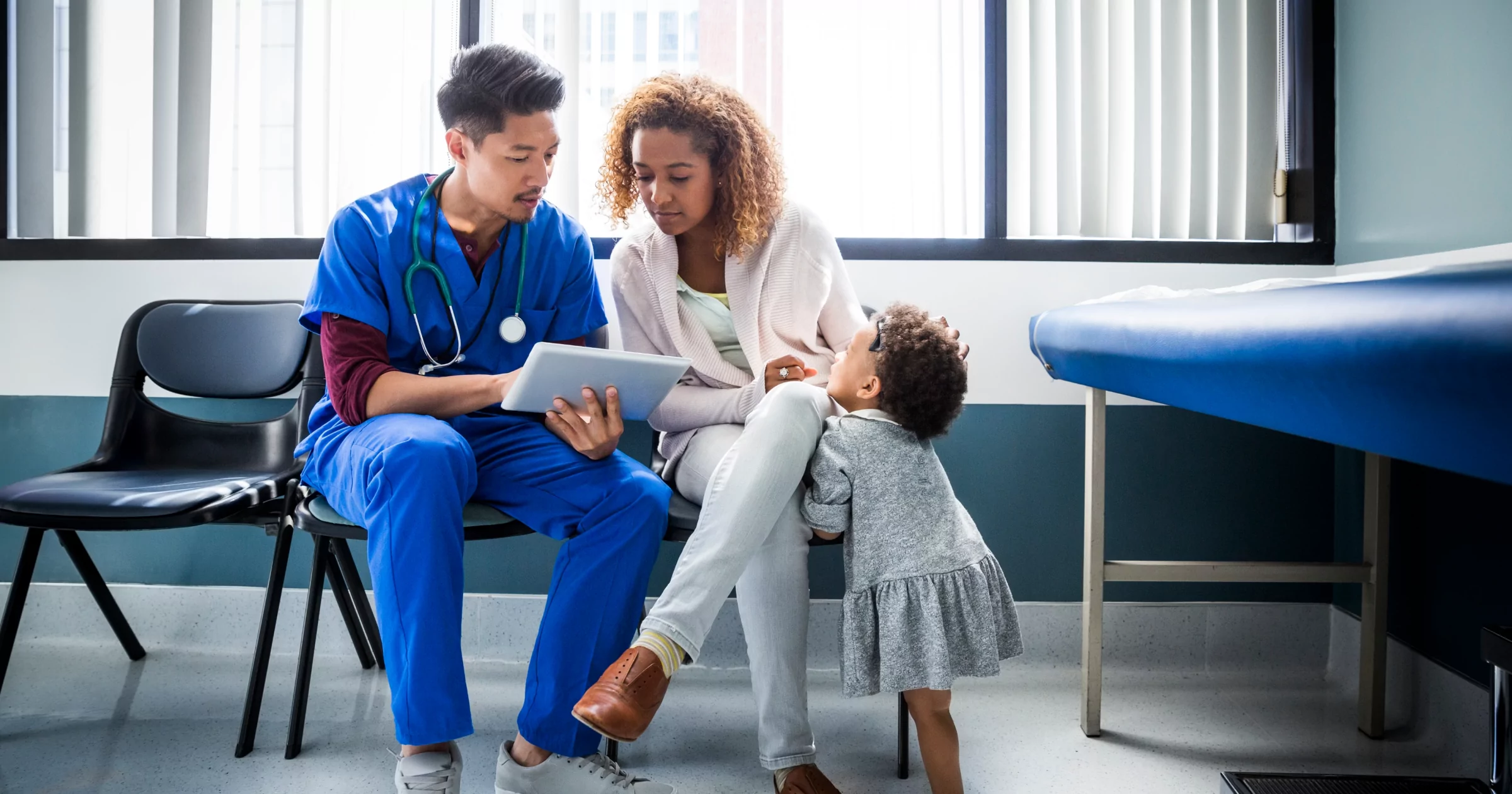
The COVID-19 pandemic revealed disturbing data about health inequity. In 2020, the National Institute for Health (NIH) published a report stating that Black Americans died from COVID-19 at higher rates than White Americans, even though they make up a smaller percentage of the population. According to the NIH, these disparities were due to limited access to care, inadequacies in public policy and a disproportionate burden of comorbidities, including cardiovascular disease, diabetes and lung diseases.
The NIH further stated that between 47.5 million and 51.6 million Americans cannot afford to go to a doctor. There is a high likelihood that historically underserved communities may use a generative transformer, especially one that is embedded unknowingly into a search engine, to ask for medical advice. It is not inconceivable that individuals would go to a popular search engine with an embedded AI agent and query, “My dad can’t afford the heart medication that was prescribed to him anymore. What is available over the counter that may work instead?”
According to researchers at Long Island University, ChatGPT is inaccurate 75% of the time, and according to CNN, the chatbot even furnished dangerous advice sometimes, such as approving the combination of two medications that could have serious adverse reactions.
Given that generative transformers do not understand meaning and will have erroneous outputs, historically underserved communities that use this technology in place of professional help may be hurt at far greater rates than others.
How can we proactively invest in AI for more equitable and trustworthy outcomes?
With today’s new generative AI products, trust, security and regulatory issues remain top concerns for government healthcare officials and C-suite leaders representing biopharmaceutical companies, health systems, medical device manufacturers and other organizations. Using generative AI requires AI governance, including conversations around appropriate use cases and guardrails around safety and trust (see AI US Blueprint for an AI Bill of Rights, the EU AI ACT and the White House AI Executive Order).
Curating AI responsibly is a sociotechnical challenge that requires a holistic approach. There are many elements required to earn people’s trust, including making sure that your AI model is accurate, auditable, explainable, fair and protective of people’s data privacy. And institutional innovation can play a role to help.
Institutional innovation: A historical note
Institutional change is often preceded by a cataclysmic event. Consider the evolution of the US Food and Drug Administration, whose primary role is to make sure that food, drugs and cosmetics are safe for public use. While this regulatory body’s roots can be traced back to 1848, monitoring drugs for safety was not a direct concern until 1937—the year of the Elixir Sulfanilamide disaster.
Created by a respected Tennessee pharmaceutical firm, Elixir Sulfanilamide was a liquid medication touted to dramatically cure strep throat. As was common for the times, the drug was not tested for toxicity before it went to market. This turned out to be a deadly mistake, as the elixir contained diethylene glycol, a toxic chemical used in antifreeze. Over 100 people died from taking the poisonous elixir, which led to the FDA’s Food, Drug and Cosmetic Act requiring drugs to be labeled with adequate directions for safe usage. This major milestone in FDA history made sure that physicians and their patients could fully trust in the strength, quality and safety of medications—an assurance we take for granted today.
Similarly, institutional innovation is required to ensure equitable outcomes from AI.
5 key steps to make sure generative AI supports the communities that it serves
The use of generative AI in the healthcare and life sciences (HCLS) field requires the same kind of institutional innovation that the FDA required during the Elixir Sulfanilamide disaster. The following recommendations can help make sure that all AI solutions achieve more equitable and just outcomes for vulnerable populations:
- Operationalize principles for trust and transparency. Fairness, explainability and transparency are big words, but what do they mean in terms of functional and non-functional requirements for your AI models? You can say to the world that your AI models are fair, but you must make sure that you train and audit your AI model to serve the most historically under-served populations. To earn the trust of the communities it serves, AI must have proven, repeatable, explained and trusted outputs that perform better than a human.
- Appoint individuals to be accountable for equitable outcomes from the use of AI in your organization. Then give them power and resources to perform the hard work. Verify that these domain experts have a fully funded mandate to do the work because without accountability, there is no trust. Someone must have the power, mindset and resources to do the work necessary for governance.
- Empower domain experts to curate and maintain trusted sources of data that are used to train models. These trusted sources of data can offer content grounding for products that use large language models (LLMs) to provide variations on language for answers that come directly from a trusted source (like an ontology or semantic search).
- Mandate that outputs be auditable and explainable. For example, some organizations are investing in generative AI that offers medical advice to patients or doctors. To encourage institutional change and protect all populations, these HCLS organizations should be subject to audits to ensure accountability and quality control. Outputs for these high-risk models should offer test-retest reliability. Outputs should be 100% accurate and detail data sources along with evidence.
- Require transparency. As HCLS organizations integrate generative AI into patient care (for example, in the form of automated patient intake when checking into a US hospital or helping a patient understand what would happen during a clinical trial), they should inform patients that a generative AI model is in use. Organizations should also offer interpretable metadata to patients that details the accountability and accuracy of that model, the source of the training data for that model and the audit results of that model. The metadata should also show how a user can opt out of using that model (and get the same service elsewhere). As organizations use and reuse synthetically generated text in a healthcare environment, people should be informed of what data has been synthetically generated and what has not.
We believe that we can and must learn from the FDA to institutionally innovate our approach to transforming our operations with AI. The journey to earning people’s trust starts with making systemic changes that make sure AI better reflects the communities it serves.
Learn how to weave responsible AI governance into the fabric of your business
More from Security
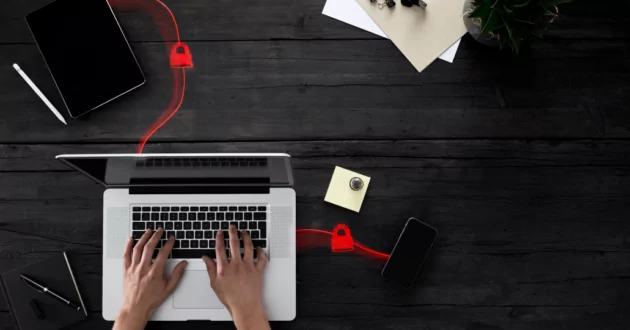
December 13, 2023
The three main types of cryptography
5 min read – Derived from the Greek words for “hidden writing,” cryptography is the science of obscuring transmitted information so that it may only be read by the intended recipient. The applications of cryptography are endless. From the quotidian end-to-end message authentication on WhatsApp to the practical digital signatures on legal forms or even the CPU-draining ciphers used for mining cryptocurrency, cryptography has become an essential aspect of our digital world and a critical cybersecurity component for protecting sensitive data from hackers and…
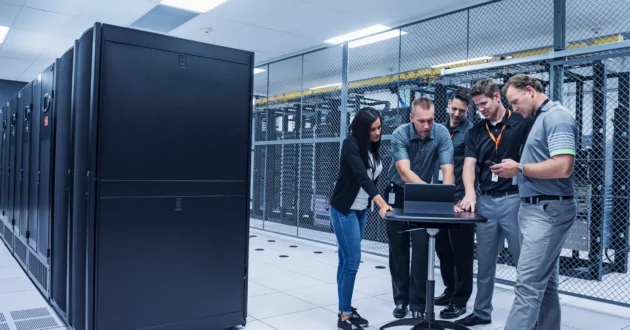
December 8, 2023
How to build a successful risk mitigation strategy
4 min read – As Benjamin Franklin once said, “If you fail to plan, you are planning to fail.” This same sentiment can be true when it comes to a successful risk mitigation plan. The only way for effective risk reduction is for an organization to use a step-by-step risk mitigation strategy to sort and manage risk, ensuring the organization has a business continuity plan in place for unexpected events. Building a strong risk mitigation strategy can set up an organization to have a…
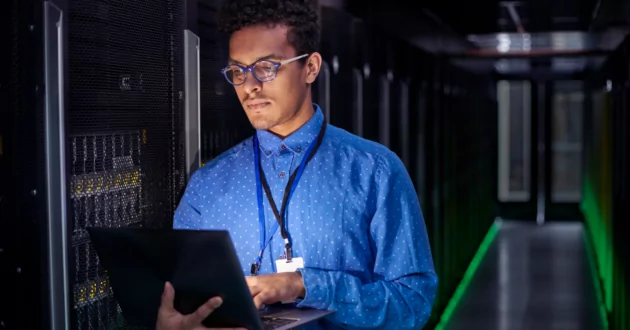
December 8, 2023
Leveraging CISA Known Exploited Vulnerabilities: Why attack surface vulnerability validation is your strongest defense
5 min read – With over 20,000 Common Vulnerabilities and Exposures (CVEs) being published each year1, the challenge of finding and fixing software with known vulnerabilities continues to stretch vulnerability management teams thin. These teams are given the impossible task of driving down risk by patching software across their organization, with the hope that their efforts will help to prevent a cybersecurity breach. Because it is impossible to patch all systems, most teams focus on remediating vulnerabilities that score highly in the Common Vulnerability…
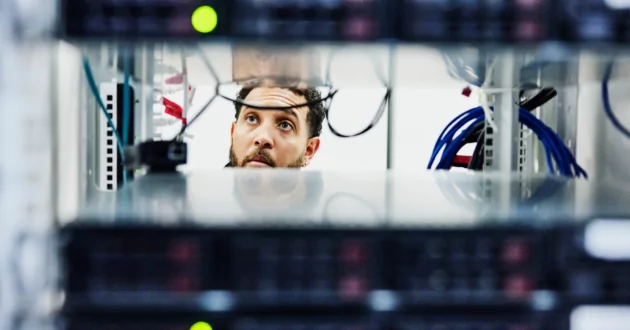
December 7, 2023
How SOAR tools can help companies comply with the latest SEC cybersecurity disclosure rules
3 min read – In July 2023, the Securities and Exchange Commission (SEC) voted to adopt new cybersecurity rules and requirements for all publicly listed companies to address risks. Among the new rules were updated requirements for filing Form 8-K as well as new disclosure obligations for Form 10-K. Under the new rule, public companies will be required to report on Form 8-K within four business days after the company determines it has experienced a material cybersecurity incident. The filed Form 8-K must describe:…
IBM Newsletters
Get our newsletters and topic updates that deliver the latest thought leadership and insights on emerging trends.
Subscribe now
More newsletters
- SEO Powered Content & PR Distribution. Get Amplified Today.
- PlatoData.Network Vertical Generative Ai. Empower Yourself. Access Here.
- PlatoAiStream. Web3 Intelligence. Knowledge Amplified. Access Here.
- PlatoESG. Carbon, CleanTech, Energy, Environment, Solar, Waste Management. Access Here.
- PlatoHealth. Biotech and Clinical Trials Intelligence. Access Here.
- Source: https://www.ibm.com/blog/delivering-responsible-ai-in-the-healthcare-and-life-sciences-industry/