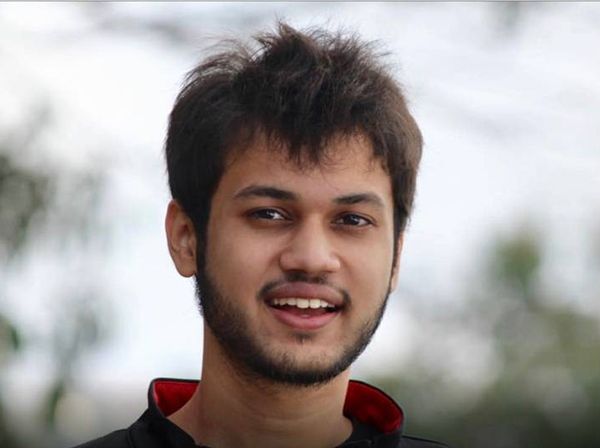
Medical research is dynamic and vast, so institutions have been apprehensive about experimenting with too many Data Management solutions. After all, the risk of exposure and, ultimately, a patient data confidentiality breach can create havoc. At the same time, there’s no denying that data plays an indispensable role in shaping the future of healthcare. In the pursuit of facilitating qualitative and timely care, on-demand data insights are required, and it can’t happen without platform interoperability.
From unraveling the microscopic analysis of diseases to evaluating the effectiveness of treatments, data serves as the cornerstone upon which medical advancements are built. However, amidst the promise lies a complex web of challenges, particularly when dealing with sensitive medical data. The invaluable insights in this data come with ethical, legal, and privacy considerations. The risks of data breaches, unauthorized access, and potential misuse loom large, necessitating stringent security measures and regulatory compliance.
Researchers, policymakers, healthcare enterprises, and patients are all navigating uncharted waters. Data anonymization, however, is emerging as a dependable practice that, to a large extent, fills the gaps and facilitates fully protected data feeds.
Importance of Data Anonymization in Medical Research
Medical research has a great responsibility to advance scientific understanding while preserving patients’ data autonomy at the same time. Personal identifiers can be dissociated through data anonymization, providing the necessary fine balance between scientific goals and privacy rights, and achieving a qualitative data feed without revealing the identities of the people from whom the data was derived.
By protecting sensitive information, researchers can establish trust with other stakeholders, such as healthcare institutions, patients, and others. As a result, patient participation increases, aiding the acquisition of accurate data and enhancing overall research quality.
Anonymization developed as a result of stricter compliance regulations. Along with GDPR, the Health Insurance Portability and Accountability Act (HIPAA) drives the anonymization initiatives in the global healthcare sector. Adhering to these regulations prevents legal repercussions and reinforces the ethical underpinning of research endeavors.
Beyond legal obligations, data anonymization fosters a culture of data sharing and collaboration among researchers. By alleviating concerns about privacy breaches, institutions are more likely to share datasets, accelerating scientific progress. Collaborative efforts become more viable, as researchers can pool resources without compromising patient confidentiality.
Data Anonymization Techniques for Medical Research
Beyond patient privacy, anonymization of data is required to enable healthcare providers to exchange insights with each other seamlessly and boost interoperability. Here are a few important techniques:
Aggregation: The data sets are sourced from multiple points before being categorized into groups based on parameters such as patient’s age, disease, region, etc., to ensure statistical relevance while masking the actual identity.
Pseudonymization: This important technique replaces identifiable information (such as names) with codes. Data scientists can enable reversible or irreversible methods to make re-identification difficult.
Swapping: As the name suggests, swapping interchanges identifiable information to create confusion for attackers. It is a simple technique that preserves the data sets’ overall characteristics without exposing the true values of identifiable information.
K-Anonymity: This technique makes it difficult to point at a specific individual’s personal information. It ensures that the data set of a particular entity is indistinguishable from at least K-1 other entities in the data set.
Data masking: The most important anonymization technique, data masking, covers (masks) the original data with fake data while not touching the underlying data structure. It makes it impossible for the attackers to decipher identifiable information about individuals. Dynamic masking enables access to data sets based on user roles, to ensure that sensitive information is hidden from unauthorized users.
In addition, various cryptographic techniques help enable access to authorized users only. There’s also data truncation, which removes specific fields that could potentially expose identifiable information while leaving the data set’s analytical value intact.
There are additional techniques, but the above-mentioned are the most impactful ones for medical records. That being said, a bigger concern is the quality of underlying Data Management platforms and how each implements anonymization.
Implementing Data Anonymization with Data Fabric
Data Management and data protection go hand in hand. For data sets as sensitive as those in medical research, fabrics are an important platform to achieve the sync. As we know, data fabric architectures streamline the need-to-end processes, including integration for diverse environments, and data anonymization only makes it more effective.
Fabrics provide a unified view of data spread across various sources, formats, and locations. Organizations can effectively harness the data for analytics, innovation, and key decision-making.
Integrating data anonymization tools into the data fabric ecosystem introduces an additional layer of security and compliance, ensuring that sensitive information remains hidden while maintaining data utility.
The integration process involves identifying and selecting appropriate data anonymization techniques, such as pseudonymization, generalization, or noise addition, and seamlessly incorporating them into the data fabric workflow.
A few examples of how data anonymization can be used in medical research:
- A pharmaceutical company uses its data masking technique to protect patient privacy in a clinical trial for a new drug to treat cancer.
- A hospital uses its solution during clinical trial management to improve the efficiency of a clinical trial for a new treatment for Alzheimer’s disease.
- A research institute uses its data-sharing solution to securely share data with researchers worldwide to study the causes of obesity.
Conclusion
Data anonymization is a vast and complicated practice, but it is essential to protect patients’ privacy while still allowing researchers to access the data they need to conduct medical research. Various anonymization techniques are available, and the best approach will vary depending on the specific data and the research goals.
- SEO Powered Content & PR Distribution. Get Amplified Today.
- PlatoData.Network Vertical Generative Ai. Empower Yourself. Access Here.
- PlatoAiStream. Web3 Intelligence. Knowledge Amplified. Access Here.
- PlatoESG. Carbon, CleanTech, Energy, Environment, Solar, Waste Management. Access Here.
- PlatoHealth. Biotech and Clinical Trials Intelligence. Access Here.
- Source: https://www.dataversity.net/leveraging-data-anonymization-for-advancements-in-medical-research/