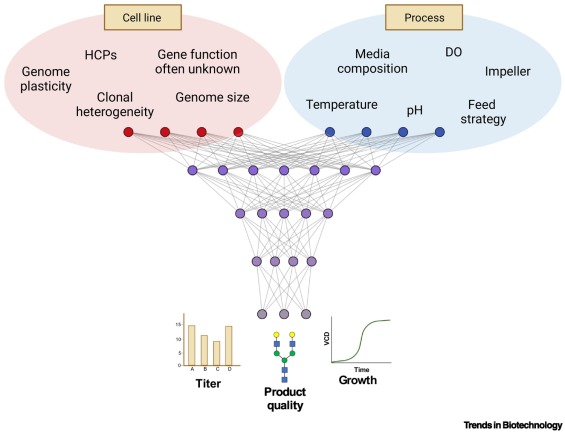
Biomanufacturing: history and perspective.
J. Ind. Microbiol. Biotechnol. 2017; 44: 773-784
Omics-driven biotechnology for industrial applications.
Front. Bioeng. Biotechnol. 2021; 9613307
From omics to cellular mechanisms in mammalian cell factory development.
Curr. Opin. Chem. Eng. 2021; 32100688
CHO-omics review: the impact of current and emerging technologies on Chinese hamster ovary based bioproduction.
Biotechnol. J. 2018; 13e1700227
From random mutagenesis to systems biology in metabolic engineering of mammalian cells.
Pharmaceut. Bioprocess. 2014; 2: 355-358
An optimized genome-wide, virus-free CRISPR screen for mammalian cells.
Cell Rep. Methods. 2021; 1100062
A metabolic CRISPR-Cas9 screen in Chinese hamster ovary cells identifies glutamine-sensitive genes.
Metab. Eng. 2021; 66: 114-122
A pooled CRISPR/AsCpf1 screen using paired gRNAs to induce genomic deletions in Chinese hamster ovary cells.
Biotechnol. Rep. (Amst). 2021; 31e00649
An arrayed CRISPR screen reveals Myc depletion to increase productivity of difficult-to-express complex antibodies in CHO cells.
Synth. Biol. 2022; 7: ysac026
De novo assembly and annotation of the CHOZN® GS genome supports high-throughput genome-scale screening.
Biotechnol. Bioeng. 2022; 119: 3632-3646
A guide to multi-omics data collection and integration for translational medicine.
Comput. Struct. Biotechnol. J. 2023; 21: 134-149
Unveiling the CHO surfaceome: identification of cell surface proteins reveals cell aggregation-relevant mechanisms.
Biotechnol. Bioeng. 2021; 118: 3015-3028
The potential of emerging sub-omics technologies for CHO cell engineering.
Biotechnol. Adv. 2022; 59107978
Multiplex secretome engineering enhances recombinant protein production and purity.
Nat. Commun. 2020; 11: 1908
Applications of proteomic methods for CHO host cell protein characterization in biopharmaceutical manufacturing.
Curr. Opin. Biotechnol. 2018; 53: 144-150
Gene expression profiling and the use of genome-scale in silico models of Escherichia coli for analysis: providing context for content.
J. Bacteriol. 2009; 191: 3437-3444
Optimizing eukaryotic cell hosts for protein production through systems biotechnology and genome-scale modeling.
Biotechnol. J. 2015; 10: 939-949
In silico cell factory design driven by comprehensive genome-scale metabolic models: development and challenges.
Syst. Microbiol. Biomanufact. 2022; (Published online July 22, 2022)
Constraining the metabolic genotype-phenotype relationship using a phylogeny of in silico methods.
Nat. Rev. Microbiol. 2012; 10: 291-305
Analysis of omics data with genome-scale models of metabolism.
Mol. BioSyst. 2013; 9: 167-174
Identifying metabolic features and engineering targets for productivity improvement in CHO cells by integrated transcriptomics and genome-scale metabolic model.
Biochem. Eng. J. 2020; 159107624
Multi-omics profiling of CHO parental hosts reveals cell line-specific variations in bioprocessing traits.
Biotechnol. Bioeng. 2019; 116: 2117-2129
CHO cell productivity improvement by genome-scale modeling and pathway analysis: application to feed supplements.
Biochem. Eng. J. 2020; 160107638
A metabolic network-based approach for developing feeding strategies for CHO cells to increase monoclonal antibody production.
Bioprocess Biosyst. Eng. 2020; 43: 1381-1389
Modulation of nutrient precursors for controlling metabolic inhibitors by genome-scale flux balance analysis.
Biotechnol. Prog. 2022; (Published online November 11, 2022)
A systematic evaluation of methods for tailoring genome-scale metabolic models.
Cell Syst. 2017; 4: 318-329.e6
Guidelines for extracting biologically relevant context-specific metabolic models using gene expression data.
Metab. Eng. 2023; 75: 181-191
Applications of genome-scale metabolic models in biotechnology and systems medicine.
Front. Physiol. 2015; 6: 413
In silico method for modelling metabolism and gene product expression at genome scale.
Nat. Commun. 2012; 3: 929
Recent advances in genome-scale modeling of proteome allocation.
Curr. Opin. Syst. Biol. 2021; 26: 39-45
Genome-scale reconstructions of the mammalian secretory pathway predict metabolic costs and limitations of protein secretion.
Nat. Commun. 2020; 11: 68
Genome-scale modeling of the protein secretory machinery in yeast.
PLoS One. 2013; 8e63284
A genome-scale metabolic network model and machine learning predict amino acid concentrations in Chinese hamster ovary cell cultures.
Biotechnol. Bioeng. 2021; 118: 2118-2123
Kinetic modeling of mammalian cell culture bioprocessing: the quest to advance biomanufacturing.
Biotechnol. J. 2018; 13e1700229
Bioprocess digital twins of mammalian cell culture for advanced biomanufacturing.
Curr. Opin. Chem. Eng. 2021; 33100702
Kinetic models in industrial biotechnology – Improving cell factory performance.
Metab. Eng. 2014; 24: 38-60
Systems analysis of N-glycan processing in mammalian cells.
PLoS One. 2007; 2e713
Model-driven engineering of N-linked glycosylation in Chinese hamster ovary cells.
ACS Synth. Biol. 2019; 8: 2524-2535
Systematic development of temperature shift strategies for Chinese hamster ovary cells based on short duration cultures and kinetic modeling.
MAbs. 2019; 11: 191-204
Predictive macroscopic modeling of cell growth, metabolism and monoclonal antibody production: case study of a CHO fed-batch production.
Metab. Eng. 2021; 66: 204-216
Statistical methods for mining Chinese hamster ovary cell ‘omics data: from differential expression to integrated multilevel analysis of the biological system.
Pharmaceut. Bioprocess. 2014; 2: 469-481
Machine learning for biochemical engineering: a review.
Biochem. Eng. J. 2021; 172108054
The era of big data: genome-scale modelling meets machine learning.
Comput. Struct. Biotechnol. J. 2020; 18: 3287-3300
Using metabolomics to identify cell line-independent indicators of growth inhibition for Chinese hamster ovary cell-based bioprocesses.
Metabolites. 2020; 10: 199
Anticipated cell lines selection in bioprocess scale-up through machine learning on metabolomics dynamics.
IFAC-PapersOnLine. 2021; 54: 85-90
Integrating metabolome dynamics and process data to guide cell line selection in biopharmaceutical process development.
Metab. Eng. 2022; 72: 353-364
Expanded Chinese hamster organ and cell line proteomics profiling reveals tissue-specific functionalities.
Sci. Rep. 2020; 10: 15841
Engineering of Chinese hamster ovary cell lipid metabolism results in an expanded ER and enhanced recombinant biotherapeutic protein production.
Metab. Eng. 2020; 57: 203-216
Metabolic profiling of Chinese hamster ovary cell cultures at different working volumes and agitation speeds using spin tube reactors.
Biotechnol. Prog. 2021; 37e3099
CHOmics: a web-based tool for multi-omics data analysis and interactive visualization in CHO cell lines.
PLoS Comput. Biol. 2020; 16e1008498
Genetic and epigenetic variation across genes involved in energy metabolism and mitochondria of Chinese hamster ovary cell lines.
Biotechnol. J. 2019; 14e1800681
Elucidation of regulatory modes for five two-component systems in Escherichia coli reveals novel relationships.
mSystems. 2020; 5e00980-20
Architecture of the human interactome defines protein communities and disease networks.
Nature. 2017; 545: 505-509
Combined multivariate statistical and flux balance analyses uncover media bottlenecks to the growth and productivity of Chinese hamster ovary cell cultures.
Biotechnol. Bioeng. 2022; 119: 1740-1754
A metabolomics approach to increasing Chinese hamster ovary (CHO) cell productivity.
Metabolites. 2021; 11: 823
Precision oncology beyond targeted therapy: combining omics data with machine learning matches the majority of cancer cells to effective therapeutics.
Mol. Cancer Res. 2018; 16: 269-278
A multi-omics supervised autoencoder for pan-cancer clinical outcome endpoints prediction.
BMC Med. Inform. Decis. Mak. 2020; 20: 129
Multi-omics profiling of a CHO cell culture system unravels the effect of culture pH on cell growth, antibody titer, and product quality.
Biotechnol. Bioeng. 2021; 118: 4305-4316
Understanding the transcriptional response to ER stress in Chinese hamster ovary cells using multiplexed single cell RNA-seq.
bioRxiv. 2022; (Published online March 31, 2022)
SCENIC: single-cell regulatory network inference and clustering.
Nat. Methods. 2017; 14: 1083-1086
scGRNom: a computational pipeline of integrative multi-omics analyses for predicting cell-type disease genes and regulatory networks.
Genome Med. 2021; 13: 95
ChEA3: transcription factor enrichment analysis by orthogonal omics integration.
Nucleic Acids Res. 2019; 47: W212-W224
Causal analysis approaches in Ingenuity Pathway Analysis.
Bioinformatics. 2014; 30: 523-530
The STRING database in 2021: customizable protein-protein networks, and functional characterization of user-uploaded gene/measurement sets.
Nucleic Acids Res. 2021; 49: D605-D612
Systematic evaluation of molecular networks for discovery of disease genes.
Cell Syst. 2018; 6: 484-495.e5
PrePPI: a structure-informed database of protein-protein interactions.
Nucleic Acids Res. 2013; 41: D828-D833
Combating viral contaminants in CHO cells by engineering innate immunity.
Sci. Rep. 2019; 9: 8827
Dysregulation of the secretory pathway connects Alzheimer’s disease genetics to aggregate formation.
Cell Syst. 2021; 12: 873-884.e4
Mathematical modeling and dynamic simulation of metabolic reaction systems using metabolome time series data.
Front. Mol. Biosci. 2016; 3: 15
Construction of feasible and accurate kinetic models of metabolism: A Bayesian approach.
Sci. Rep. 2016; 6: 29635
Systematic analysis of stability patterns in plant primary metabolism.
PLoS One. 2012; 7e34686
iSCHRUNK–In silico approach to characterization and reduction of uncertainty in the kinetic models of genome-scale metabolic networks.
Metab. Eng. 2016; 33: 158-168
Predicting growth conditions from internal metabolic fluxes in an in-silico model of E. coli.
PLoS One. 2014; 9e114608
Combining mechanistic and machine learning models for predictive engineering and optimization of tryptophan metabolism.
Nat. Commun. 2020; 11: 4880
Metabolic network prediction of drug side effects.
Cell Syst. 2016; 2: 209-213
Hybrid models based on machine learning and an increasing degree of process knowledge: application to cell culture processes.
Ind. Eng. Chem. Res. 2022; 61: 8658-8672
Moving towards an era of hybrid modelling: advantages and challenges of coupling mechanistic and data-driven models for upstream pharmaceutical bioprocesses.
Curr. Opin. Chem. Eng. 2021; 32100691
Harnessing the potential of artificial neural networks for predicting protein glycosylation.
Metab. Eng. Commun. 2020; 10e00131
Model-based optimization of antibody galactosylation in CHO cell culture.
Biotechnol. Bioeng. 2019; 116: 1612-1626
Machine and deep learning meet genome-scale metabolic modeling.
PLoS Comput. Biol. 2019; 15e1007084
Model-based assessment of mammalian cell metabolic functionalities using omics data.
Cell Rep. Methods. 2021; 1100040
Assessing key decisions for transcriptomic data integration in biochemical networks.
PLoS Comput. Biol. 2019; 15e1007185
How to train your cell – Towards controlling phenotypes by harnessing the epigenome of Chinese hamster ovary production cell lines.
Biotechnol. Adv. 2022; 56107924
The Cancer Genome Atlas (TCGA): an immeasurable source of knowledge.
Contemp. Oncol. 2015; 19: A68-A77
International network of cancer genome projects.
Nature. 2010; 464: 993-998
An integrated encyclopedia of DNA elements in the human genome.
Nature. 2012; 489: 57-74
New developments on the Encyclopedia of DNA Elements (ENCODE) data portal.
Nucleic Acids Res. 2020; 48: D882-D889
Quantitative feature extraction from the Chinese hamster ovary bioprocess bibliome using a novel meta-analysis workflow.
Biotechnol. Adv. 2016; 34: 621-633
Metabolic flux analysis and metabolic engineering of microorganisms.
Mol. BioSyst. 2008; 4: 113-120
A protocol for generating a high-quality genome-scale metabolic reconstruction.
Nat. Protoc. 2010; 5: 93-121
Current status and applications of genome-scale metabolic models.
Genome Biol. 2019; 20: 121
Systems properties of the Haemophilus influenzae Rd metabolic genotype.
J. Biol. Chem. 1999; 274: 17410-17416
The Escherichia coli MG1655 in silico metabolic genotype: its definition, characteristics, and capabilities.
Proc. Natl. Acad. Sci. U. S. A. 2000; 97: 5528-5533
Genome-scale reconstruction of the Saccharomyces cerevisiae metabolic network.
Genome Res. 2003; 13: 244-253
iBsu1103: a new genome-scale metabolic model of Bacillus subtilis based on SEED annotations.
Genome Biol. 2009; 10: R69
Metabolic engineering of based on genome-scale metabolic model to promote fengycin production.
3 Biotech. 2021; 11: 448
Integrating metabolic modeling and population heterogeneity analysis into optimizing recombinant protein production by Komagataella (Pichia) pastoris.
Appl. Microbiol. Biotechnol. 2018; 102: 63-80
Global reconstruction of the human metabolic network based on genomic and bibliomic data.
Proc. Natl. Acad. Sci. U. S. A. 2007; 104: 1777-1782
A consensus genome-scale reconstruction of Chinese hamster ovary cell metabolism.
Cell Syst. 2016; 3: 434-443.e8
BiGG Models 2020: multi-strain genome-scale models and expansion across the phylogenetic tree.
Nucleic Acids Res. 2020; 48: D402-D406
Reconstructing organisms in silico: genome-scale models and their emerging applications.
Nat. Rev. Microbiol. 2020; 18: 731-743
Kinetic modeling of metabolism: Present and future.
Curr. Opin. Syst. Biol. 2021; 26: 72-78
Cytoscape: a software environment for integrated models of biomolecular interaction networks.
Genome Res. 2003; 13: 2498-2504
- SEO Powered Content & PR Distribution. Get Amplified Today.
- Platoblockchain. Web3 Metaverse Intelligence. Knowledge Amplified. Access Here.
- Minting the Future w Adryenn Ashley. Access Here.
- Source: https://www.cell.com/trends/biotechnology/fulltext/S0167-7799(23)00090-2?rss=yes