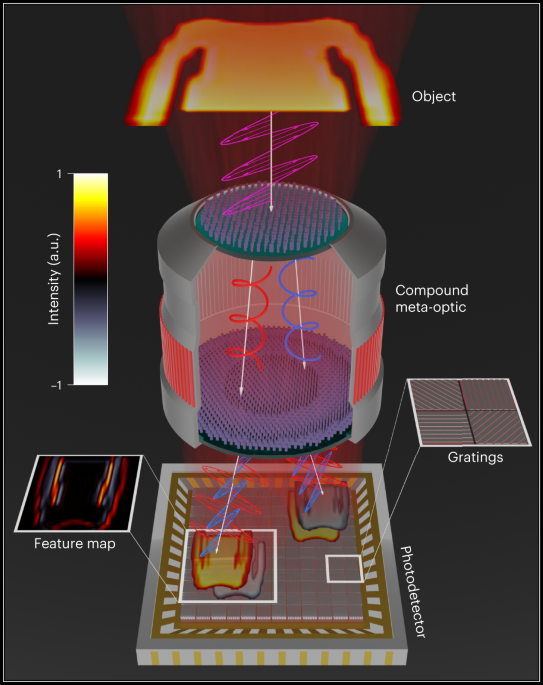
Simonyan, K. & Zisserman, A. Very deep convolutional networks for large-scale image recognition. In 3rd International Conference on Learning Representations 1–14 (ICLR, 2015).
Wang, G. et al. Interactive medical image segmentation using deep learning with image-specific fine tuning. IEEE Trans. Med. Imaging 37, 1562–1573 (2018).
Furui, S., Deng, L., Gales, M., Ney, H. & Tokuda, K. Fundamental technologies in modern speech recognition. IEEE Signal Process Mag. 29, 16–17 (2012).
Sak, H., Senior, A., Rao, K. & Beaufays, F. Fast and accurate recurrent neural network acoustic models for speech recognition. In Proc. Annual Conference of the International Speech Communication Association, INTERSPEECH 1468–1472 (ISCA, 2015).
He, K., Zhang, X., Ren, S. & Sun, J. Deep residual learning for image recognition. In Proc. IEEE Computer Society Conference on Computer Vision and Pattern Recognition 770–778 (IEEE, 2016).
Lecun, Y., Bengio, Y. & Hinton, G. Deep learning. Nature 521, 436–444 (2015).
Mennel, L. et al. Ultrafast machine vision with 2D material neural network image sensors. Nature 579, 62–66 (2020).
Liu, L. et al. Computing systems for autonomous driving: state of the art and challenges. IEEE Internet Things J. 8, 6469–6486 (2021).
Shi, W. et al. LOEN: lensless opto-electronic neural network empowered machine vision. Light Sci. Appl. 11, 121 (2022).
Hamerly, R., Bernstein, L., Sludds, A., Soljačić, M. & Englund, D. Large-scale optical neural networks based on photoelectric multiplication. Phys. Rev. X 9, 021032 (2019).
Wetzstein, G. et al. Inference in artificial intelligence with deep optics and photonics. Nature 588, 39–47 (2020).
Shastri, B. J. et al. Photonics for artificial intelligence and neuromorphic computing. Nat. Photon. 15, 102–114 (2021).
Xue, W. & Miller, O. D. High-NA optical edge detection via optimized multilayer films. J. Optics 23, 125004 (2021).
Wang, T. et al. An optical neural network using less than 1 photon per multiplication. Nat. Commun. 13, 123 (2022).
Wang, T. et al. Image sensing with multilayer nonlinear optical neural networks. Nat. Photon. 17, 8–17 (2023).
Badloe, T., Lee, S. & Rho, J. Computation at the speed of light: metamaterials for all-optical calculations and neural networks. Adv. Photon. 4, 064002 (2022).
Vanderlugt, A. Optical Signal Processing (Wiley, 1993).
Chang, J., Sitzmann, V., Dun, X., Heidrich, W. & Wetzstein, G. Hybrid optical-electronic convolutional neural networks with optimized diffractive optics for image classification. Sci. Rep. 8, 12324 (2018).
Colburn, S., Chu, Y., Shilzerman, E. & Majumdar, A. Optical frontend for a convolutional neural network. Appl. Opt. 58, 3179 (2019).
Zhou, T. et al. Large-scale neuromorphic optoelectronic computing with a reconfigurable diffractive processing unit. Nat. Photon. 15, 367–373 (2021).
Chen, Y. H., Krishna, T., Emer, J. S. & Sze, V. Eyeriss: an energy-efficient reconfigurable accelerator for deep convolutional neural networks. IEEE J. Solid-State Circuits 52, 127–138 (2017).
Neshatpour, K., Homayoun, H. & Sasan, A. ICNN: the iterative convolutional neural network. In ACM Transactions on Embedded Computing Systems 18, 119 (ACM, 2019).
Xu, X. et al. 11 TOPS photonic convolutional accelerator for optical neural networks. Nature 589, 44–51 (2021).
Feldmann, J. et al. Parallel convolutional processing using an integrated photonic tensor core. Nature 589, 52–58 (2021).
Wu, C. et al. Programmable phase-change metasurfaces on waveguides for multimode photonic convolutional neural network. Nat. Commun. 12, 96 (2021).
Zhang, H. et al. An optical neural chip for implementing complex-valued neural network. Nat. Commun. 12, 457 (2021).
Ashtiani, F., Geers, A. J. & Aflatouni, F. An on-chip photonic deep neural network for image classification. Nature 606, 501–506 (2022).
Fu, T. et al. Photonic machine learning with on-chip diffractive optics. Nat. Commun. 14, 70 (2023).
Lin, X. et al. All-optical machine learning using diffractive deep neural networks. Science 361, 1004–1008 (2018).
Qian, C. et al. Performing optical logic operations by a diffractive neural network. Light Sci. Appl. 9, 59 (2020).
Luo, X. et al. Metasurface-enabled on-chip multiplexed diffractive neural networks in the visible. Light Sci. Appl. 11, 158 (2022).
Kwon, H., Arbabi, E., Kamali, S. M., Faraji-Dana, M. S. & Faraon, A. Single-shot quantitative phase gradient microscopy using a system of multifunctional metasurfaces. Nat. Photon. 14, 109–114 (2020).
Xiong, B. et al. Breaking the limitation of polarization multiplexing in optical metasurfaces with engineered noise. Science 379, 294–299 (2023).
Khorasaninejad, M. et al. Metalenses at visible wavelengths: diffraction-limited focusing and subwavelength resolution imaging. Science 352, 1190–1194 (2016).
Kim, J. et al. Scalable manufacturing of high-index atomic layer–polymer hybrid metasurfaces for metaphotonics in the visible. Nat. Mater. 22, 474–481 (2023).
Levanon, N. et al. Angular transmission response of in-plane symmetry-breaking quasi-BIC all-dielectric metasurfaces. ACS Photonics 9, 3642–3648 (2022).
Nolen, J. R., Overvig, A. C., Cotrufo, M. & Alù, A. Arbitrarily polarized and unidirectional emission from thermal metasurfaces. Preprint at https://arxiv.org/abs/2301.12301 (2023).
Guo, C., Xiao, M., Minkov, M., Shi, Y. & Fan, S. Photonic crystal slab Laplace operator for image differentiation. Optica 5, 251–256 (2018).
Cordaro, A. et al. High-index dielectric metasurfaces performing mathematical operations. Nano Lett. 19, 8418–8423 (2019).
Zhou, Y., Zheng, H., Kravchenko, I. I. & Valentine, J. Flat optics for image differentiation. Nat. Photon. 14, 316–323 (2020).
Fu, W. et al. Ultracompact meta-imagers for arbitrary all-optical convolution. Light Sci. Appl. 11, 62 (2022).
Wang, H., Guo, C., Zhao, Z. & Fan, S. Compact incoherent image differentiation with nanophotonic structures. ACS Photonics 7, 338–343 (2020).
Zhang, X., Bai, B., Sun, H. B., Jin, G. & Valentine, J. Incoherent optoelectronic differentiation based on optimized multilayer films. Laser Photon Rev. 16, 2200038 (2022).
Zheng, H. et al. Meta-optic accelerators for object classifiers. Sci. Adv. 8, eabo6410 (2022).
Bernstein, L. et al. Single-shot optical neural network. Sci. Adv. 9, eadg7904 (2023).
Shen, Z. et al. Monocular metasurface camera for passive single-shot 4D imaging. Nat. Commun. 14, 1035 (2023).
LeCun, Y., Bottou, L., Bengio, Y. & Haffner, P. Gradient-based learning applied to document recognition. Proc. IEEE 86, 2278–2323 (1998).
Zheng, H. et al. Compound meta-optics for complete and loss-less field control. ACS Nano 16, 15100–15107 (2022).
Liu, S. et al. More ConvNets in the 2020s: scaling up kernels beyond 51×51 using sparsity. In 11th International Conference on Learning Representations 1–23 (ICLR, 2023).
Barron, J. T. A general and adaptive robust loss function. In Proc. IEEE Computer Society Conference on Computer Vision and Pattern Recognition 4326–4334 (IEEE, 2019).
Dosovitskiy, A. et al. An image is worth 16×16 words: transformers for image recognition at scale. In 9th International Conference on Learning Representations 1–22 (ICLR, 2021).
Stillmaker, A. & Baas, B. Scaling equations for the accurate prediction of CMOS device performance from 180 nm to 7 nm. Integration 58, 74–81 (2017).
McClung, A., Samudrala, S., Torfeh, M., Mansouree, M. & Arbabi, A. Snapshot spectral imaging with parallel metasystems. Sci. Adv. 6, eabc7646 (2020).
Ding, X., Zhang, X., Han, J. & Ding, G. Scaling up your kernels to 31 × 31: revisiting large kernel design in CNNs. In Proc. IEEE Computer Society Conference on Computer Vision and Pattern Recognition 11953–11965 (IEEE, 2022).
Ding, X. et al. RepVgg: making VGG-style ConvNets great again. In Proc. IEEE Computer Society Conference on Computer Vision and Pattern Recognition 13728–13737 (IEEE, 2021).
Li, L. et al. Intelligent metasurface imager and recognizer. Light Sci. Appl. 8, 97 (2019).
Zhao, R. et al. Multichannel vectorial holographic display and encryption. Light Sci. Appl. 7, 95 (2018).
Kim, I. et al. Pixelated bifunctional metasurface-driven dynamic vectorial holographic color prints for photonic security platform. Nat. Commun. 12, 3614 (2021).
Li, L. et al. Metalens-array-based high-dimensional and multiphoton quantum source. Science 368, 1487–1490 (2020).
Hugonin, A. J. P. & Lalanne, P. RETICOLO software for grating analysis. Preprint at https://arxiv.org/abs/2101.00901 (2023).
- SEO Powered Content & PR Distribution. Get Amplified Today.
- PlatoData.Network Vertical Generative Ai. Empower Yourself. Access Here.
- PlatoAiStream. Web3 Intelligence. Knowledge Amplified. Access Here.
- PlatoESG. Carbon, CleanTech, Energy, Environment, Solar, Waste Management. Access Here.
- PlatoHealth. Biotech and Clinical Trials Intelligence. Access Here.
- Source: https://www.nature.com/articles/s41565-023-01557-2