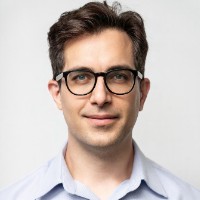
The ability of a computer to generate prose text has recently become good enough to consider for practical business use. So why are most companies not using it, yet? Let’s look at some challenges in implementing these methods. While generative AI (GenAI)
can also generate images, audio, or video, we will focus on its ability to generate text here.
At the heart of GenAI lies a model, which transforms one piece of text into another. The input text is often a question asked or a command given by a human user. The output text is, hopefully, a correct and meaningful response. Most of us have played with
one or more of these models online in a text-messaging environment reminiscent of a conversation. Despite seeming like a conversation, cracks appear signaling to us that we are not talking to a human being.
The first group of challenges lies in how these models were made. They are based on huge text collections from the internet. Much of this text is fictional or contains inappropriate speech such as discrimination. Lots of this text is also subject to copyright
law, which makes the models’ legality somewhat unclear.
The next group of challenges has to do with the very nature of these models. They represent a gigantic probability matrix of what word is most likely to follow a given starting sequence of words. As such, they are not capable of logical reasoning, causal
argumentation, or common sense. The practical upshot is that they occasionally give incorrect or impossible answers — something called a hallucination.
Furthermore, in business practice these models cannot live on their own but must be integrated into a variety of other software tools, often made by other vendors. The GenAI models can then represent a language interface to these software tools to streamline
many tasks. However, the work of integrating GenAI models with legacy software has only just begun and is made complex by the diverse, and quickly changing, landscape of vendors themselves.
Supposing that GenAI were fully integrated into the common software utilities used in the financial services industry, we would still face the challenge of training and change management in the workforce of an industry that prides itself on human intelligence.
Those are all challenges in principle. Let’s put them aside for now and ask what we would employ GenAI to do in financial services.
Some uses are common with other industries like the automation of customer service in answering questions or doing routine tasks like a smart automated hotline. One can send marketing emails to many customers intricately tailored to each individual’s behavior
pattern to advertise specific products and services truly suitable to that person.
It gets more interesting when we realize that GenAI does not just speak human languages but computer languages also. It can translate a question posed in English into SQL, the language of databases, or into JavaScript, the language of web pages. A financial
analyst may ask a question in English, have this put to a database in perfect SQL and the answer transformed into a JavaScript page that displays as an analytics chart. For the financial analyst, the chart appears instantly with trustworthy numerical data.
It’s trustworthy because GenAI did not create the numerical content but rather retrieved it from a well-formed database. The instantaneous answer is a significant gain as all the human work and delay is saved.
GenAI is able to write prose text natively and so can provide a first draft of a financial analysis or report to be corrected by a human. It is well documented that the automation of the first draft can save as much as 40% of the total human labor effort
for the report.
Summing up, the main challenges lie with the models themselves and their integration into other tools. Once integrated, they must be used correctly by a workforce that is willing and trained to do so.
This brings us to the final obstacle to adoption in financial services: Trust. Finance professionals, corporate executives, and governmental regulators alike do not yet quite trust these technologies to be as reliable as we would like them to be to serve
a regulated industry in which large sums of money can be lost in a moment. This must be met with integrations like the one mentioned above to control GenAI with precise databases, and also with better advocacy of the AI industry itself so that understanding
conquers lack of trust.
- SEO Powered Content & PR Distribution. Get Amplified Today.
- PlatoData.Network Vertical Generative Ai. Empower Yourself. Access Here.
- PlatoAiStream. Web3 Intelligence. Knowledge Amplified. Access Here.
- PlatoESG. Carbon, CleanTech, Energy, Environment, Solar, Waste Management. Access Here.
- PlatoHealth. Biotech and Clinical Trials Intelligence. Access Here.
- Source: https://www.finextra.com/blogposting/25582/implementation-challenges-of-genai-in-financial-services?utm_medium=rssfinextra&utm_source=finextrablogs