Ever wonder how does AI generate images that amaze all of us?
AI, or artificial intelligence, is a broad field of computer science that seeks to create intelligent machines capable of performing tasks typically requiring human intelligence. It’s not a single technology, but rather a collection of techniques and approaches that allow machines to learn, reason, and act autonomously.
Although it is a technology that we are inspired by today, this technology, which has attracted a lot of criticism in the field of art and image generation, has improved considerably in imitating humans as of 2024.
But how does AI generate images? Well, let us explain.
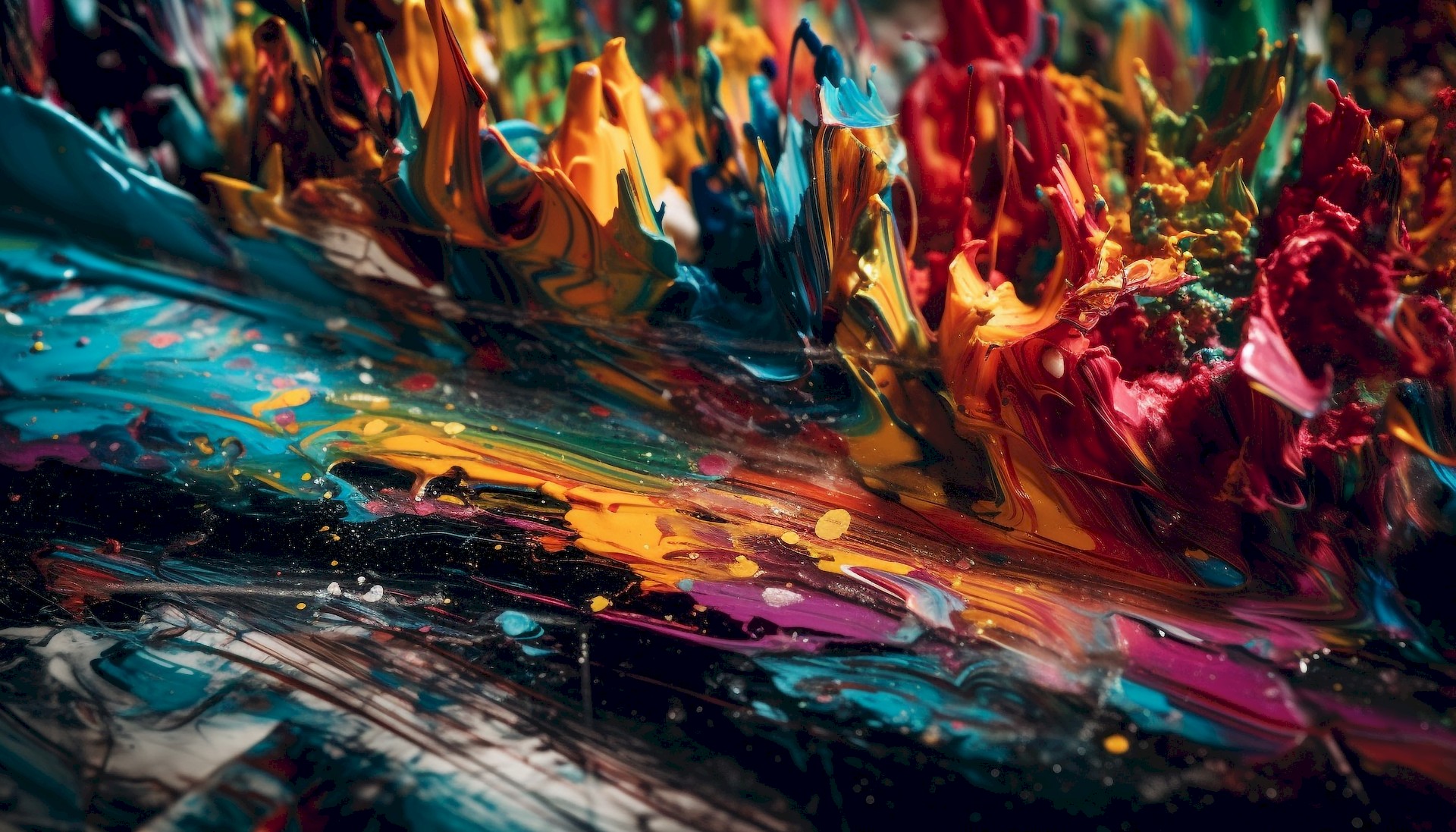
How does AI generate images?
AI possesses the remarkable capability to create visual content through the utilization of diverse methodologies, encompassing a spectrum of techniques. These methods, employed by AI, enable the generation of images in a manner that showcases the versatility and ingenuity embedded within artificial intelligence systems.
If you ever found yourself wondering how does AI generate images, these are the most common methods used by AI systems to generate art pieces we all admire:
- Generative Adversarial Networks (GANs)
- Variational Autoencoders (VAEs)
- Convolutional Neural Networks (CNNs)
- Recurrent Beural Networks (RNNs)
- Image-to-image translation
- Text-to-image synthesis
- Style transfer
Generative Adversarial Networks (GANs)
GANs are a type of deep learning algorithm used for generating new images. They consist of two neural networks: a generator and a discriminator. The generator creates new images, while the discriminator evaluates the generated images and tells the generator whether they are realistic or not. The two networks work together to improve the generator’s ability to create realistic images.
The generator network takes a random noise vector as input and produces a synthetic image. The discriminator network takes the synthetic image and a real image as input and predicts the probability that the image is real. During training, the generator tries to produce images that can fool the discriminator into thinking they are real, while the discriminator tries to correctly classify the images as real or fake.
GANs have been used to generate a wide range of images, including faces, objects, and scenes. They have also been used in various applications such as image-to-image translation, data augmentation, and style transfer.
Although GANs are not the only answer to the question of how does AI generate images, it is a very important element.
Variational Autoencoders (VAEs)
Another way to answer how does AI generate images is to say by Variational Autoencoders (VAEs).
VAEs are another type of deep learning algorithm used for generating new images. They consist of an encoder network and a decoder network. The encoder network maps the input image to a latent space, which is a lower-dimensional representation of the image. The decoder network maps the latent space back to the input image.
During training, the VAE learns to minimize the difference between the input image and the reconstructed image. The VAE also learns a probabilistic distribution over the latent space, which can be used to generate new images.
To generate a new image, the VAE samples a latent code from the probabilistic distribution and passes it through the decoder network. The decoder network generates a new image based on the latent code.
VAEs have been used to generate images that are similar to the training data, but they can also be used to generate images that are not present in the training data. They have been used in various applications such as image generation, image-to-image translation, and data augmentation.
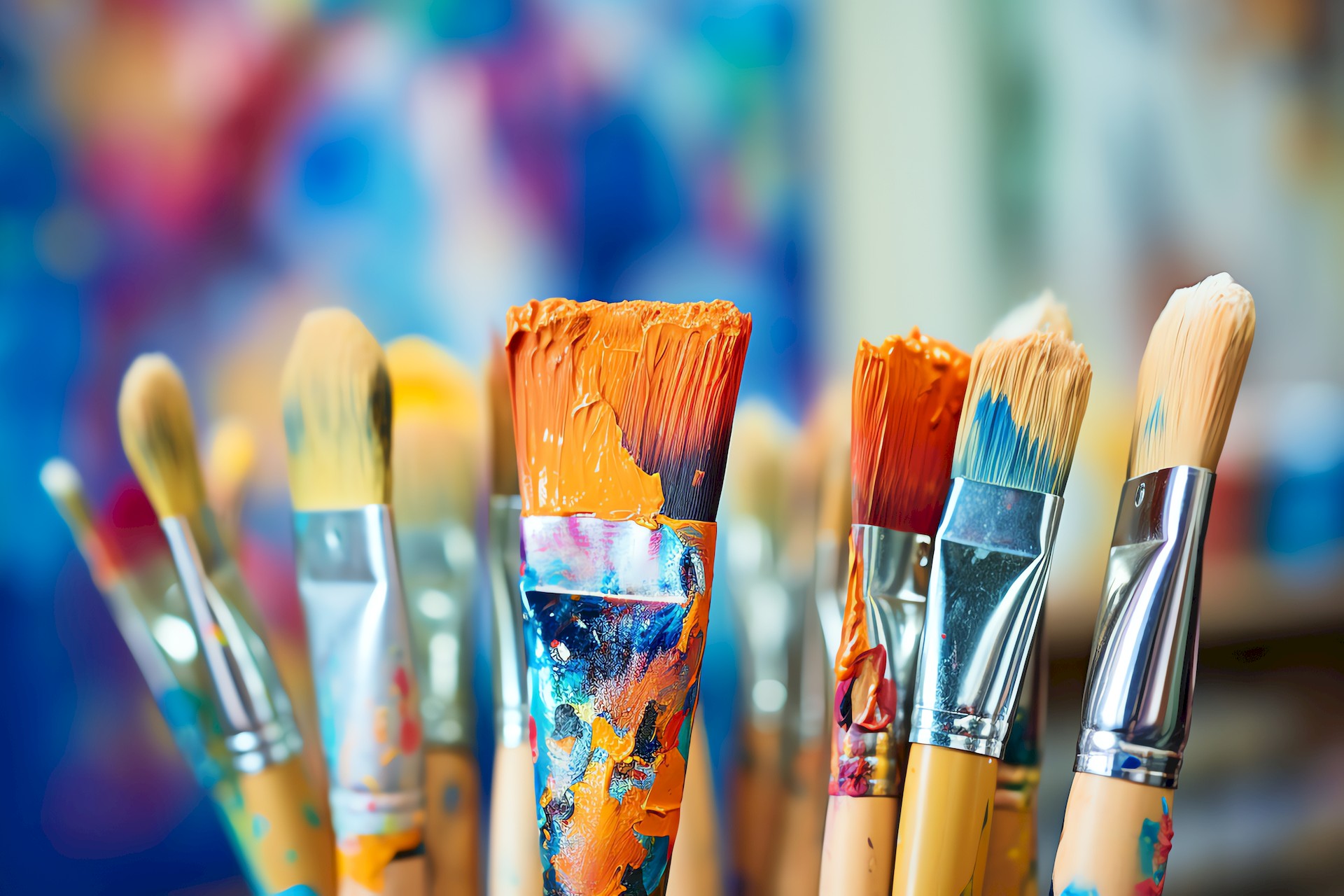
Convolutional Neural Networks (CNNs)
CNNs are a type of neural network that have been widely used for image processing tasks. They can be used to generate new images by learning the patterns and structures of images and then generating new images based on these patterns.
CNNs consist of multiple convolutional layers that learn to detect increasingly complex features within images. The convolutional layers are followed by pooling layers that reduce the spatial dimensions of the feature maps. Finally, fully connected layers are used to make the final predictions.
To generate a new image using a CNN, the network takes a random noise vector as input and passes it through the convolutional and pooling layers. The fully connected layers then generate a new image based on the feature maps produced by the convolutional and pooling layers.
CNNs have been used to generate images that are similar to the training data, but they can also be used to generate images that are not present in the training data. They have been used in various applications such as image generation, image-to-image translation, and data augmentation.
And as a result, the CNN method can also be given as a potential answer to the question of how does AI generate images.
Recurrent Neural Networks (RNNs)
RNNs are a type of neural network that are well-suited for processing sequential data such as text or time-series data. They can also be used to generate images by learning the sequences of pixels in images and then generating new sequences of pixels to create new images.
RNNs consist of a loop of recurrent connections that allow information from previous time steps to influence the current step. This allows the network to capture temporal dependencies in the data.
To generate a new image using an RNN, the network takes a random initialization of the image pixels as input and processes it through the recurrent loop. At each time step, the network applies a nonlinear activation function to the current state of the pixels and uses the output as the new state. This process continues until the desired length of the image is reached.
RNNs have been used to generate images that are similar to the training data, but they can also be used to generate images that are not present in the training data. They have been used in various applications such as image generation, image-to-image translation, and data augmentation.
Image-to-image translation
Image-to-image translation is a technique that involves training a neural network to translate an input image into a new image with desired attributes. For example, translating a photo of a cat to a painting.
This technique can be used to generate new images that are not present in the training data. The network learns to translate the input image into a new image based on the patterns and structures learned from the training data.
Image-to-image translation has been used in various applications such as style transfer, image synthesis, and data augmentation.
Text-to-image synthesis
Text-to-image synthesis is a technique that involves generating an image based on a textual description. For example, generating an image of a cat based on the text “a black cat with white paws”.
This technique can be used to generate new images that are not present in the training data. The network learns to generate images based on the patterns and structures learned from the training data and the textual description.
Text-to-image synthesis has been used in various applications such as image generation, image-to-image translation, and data augmentation.
While the question of how does AI generate images remains to be answered, AI-powered applications such as Adobe Firefly, which specializes in the text-to-image method, are likely to remain on the agenda for a long time to come.

Style transfer
Style transfer is a technique that involves transferring the style of one image to another image. For example, transferring the style of a painting to a photo of a cat.
This technique can be used to generate new images that are not present in the training data. The network learns to transfer the style of the input image to a new image based on the patterns and structures learned from the training data.
Style transfer has been used in various applications such as image generation, image-to-image translation, and data augmentation.
Inspiration of one, hatred of the other
Knowing how does AI generate images is far from understanding the sensitivity of this technology.
AI image generation’s magic sparklers a dazzling array of possibilities, but its glitter also casts shadows of ethical concern. One lurking beast is bias: the algorithms trained on vast datasets often reflect societal prejudices, spitting out images skewed by race, gender, or other factors. This can perpetuate harmful stereotypes and marginalize already vulnerable groups.
Then comes the thorny issue of copyright and authorship. AI art borrows heavily from existing works, raising questions about who truly owns the creation. Should artists whose styles are mimicked be compensated? Or does the AI itself deserve credit? Unresolved legal gray areas abound.
Artists’ case for copyrights against AI faces an uphill battle
Misinformation lurks around the corner too. Hyper-realistic AI-generated images can blur the lines between truth and fiction, fueling the spread of “deepfakes” and manipulated narratives. This can erode trust in media, sow discord, and even influence elections.
Finally, the impact on human creativity deserves a pause. Will AI replace artists, leaving canvases bare and studios silent? Or will it spark new forms of collaboration, amplifying human imagination with its digital brushstrokes? Navigating this new artistic landscape demands careful consideration.
These ethical dilemmas require open dialogue, robust regulations, and responsible development. Only then can AI image generation truly paint a brighter future for art, technology, and society as a whole. Well, at least after this writing you don’t have to wonder how does AI generate images anymore.
Featured image credit: Vecstock/Freepik.
- SEO Powered Content & PR Distribution. Get Amplified Today.
- PlatoData.Network Vertical Generative Ai. Empower Yourself. Access Here.
- PlatoAiStream. Web3 Intelligence. Knowledge Amplified. Access Here.
- PlatoESG. Carbon, CleanTech, Energy, Environment, Solar, Waste Management. Access Here.
- PlatoHealth. Biotech and Clinical Trials Intelligence. Access Here.
- Source: https://dataconomy.com/2024/01/08/how-does-ai-generate-images/