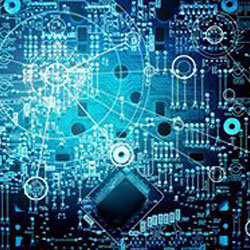
This paper presents a comprehensive literature review for applying large language models (LLM) in multiple aspects of functional verification. Despite the promising advancements offered by this new technology, it is essential to be aware of the inherent limitations of LLMs, especially hallucination that may lead to incorrect predictions. To ensure the quality of LLM outputs, four safeguarding paradigms are recommended. Finally, the paper summarizes the observed trend of LLM development and expresses optimism about their broader applications in verification.
Paradigms of LLM for functional verification
Language models are arguably the most essential types of machine learning (ML) models used for functional verification. This process involves handling numerous forms of textual data, including specifications, source code, test plans, testbenches, logs, and reports. Most of the textual content comprises natural languages, controlled natural languages, or programming languages. Therefore, effective use of language models is critical for the application of AI/ML in functional verification.
Despite these promising advancements offered by this new technology, it is essential to be aware of the inherent limitations of Large Language Models (LLM) that lead to incorrect predictions. In particular, we caution against using raw outputs of LLMs directly in verification.
To counter the limitations and deliver on their promise, the authors recommend four safeguarding paradigms to ensure the quality of LLM outputs:
- Quality gate/guardrail
- Self-check feedback loop
- External agent
- Chain-of-thought
To read more, click here.
- SEO Powered Content & PR Distribution. Get Amplified Today.
- PlatoData.Network Vertical Generative Ai. Empower Yourself. Access Here.
- PlatoAiStream. Web3 Intelligence. Knowledge Amplified. Access Here.
- PlatoESG. Carbon, CleanTech, Energy, Environment, Solar, Waste Management. Access Here.
- PlatoHealth. Biotech and Clinical Trials Intelligence. Access Here.
- Source: https://semiengineering.com/paradigms-of-large-language-model-applications-in-functional-verification/