Dec 25, 2023 |
|
(Nanowerk Spotlight) Two-dimensional (2D) materials like graphene have attracted great interest across science and industry for their remarkable electronic, optical, and mechanical properties. Yet despite intense study, fewer than 100 distinct 2D materials have been successfully synthesized in labs. This severely limits their practical use in transistors, sensors, batteries, and other applications.
|
Now, researchers have developed an artificial intelligence system that automates the discovery and validation of chemically stable 2D materials. This breakthrough technique already found six promising new 2D candidates overlooked by previous manual searches.
|
They report their findings in Advanced Intelligent Systems (“Discovery of 2D Materials using Transformer Network-Based Generative Design”).
|
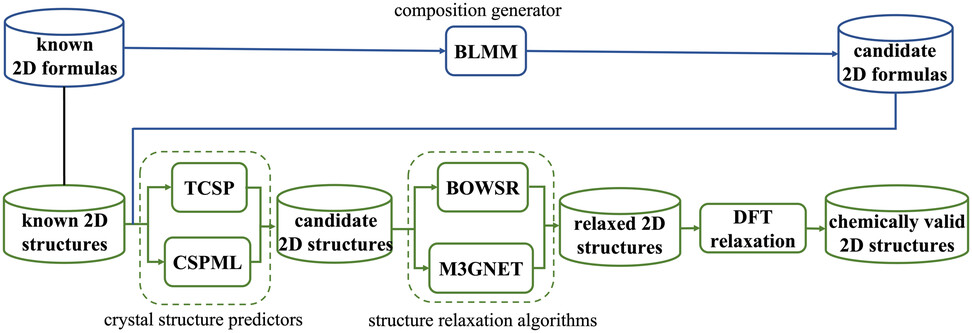 |
Architecture of the material transformer generator (MTG) pipeline. BLMM is a transformer neural network-based composition generator. TCSP and CSPML are template-based crystal structure prediction algorithms, and BOWSR and M3GNET are machine learning potential-based structure relaxing algorithms. DFT relaxation is a first-principles calculation method. (Reprinted with permission from Wiley-VCH Verlag)
|
Historically, new materials were found through painstaking trial-and-error experiments guided by human chemical intuition. But the vast search space of possible stable compounds makes brute-force testing implausible. “There are too many potential new materials to ever synthesize and characterize one-by-one in labs,” explains study co-author Jianjun Hu. “We need computational tools to intelligently explore possibilities.”
|
Over the past decade, high-throughput virtual screening has become viable by leveraging advances in computing power and electronic structure algorithms. But even automated approaches struggle to account for the immense combinatorics of mixing elements into stable 2D geometries. “Machine learning removes humans from the discovery loop and lets algorithms teach themselves by looking across materials data,” says Hu.
|
The new technique, termed Material Transformer Generator (MTG), integrates several AI components. First, it uses a neural sequence model to generate atom combinations that obey charge neutrality requirements. Next, these compositions get fed into specialized crystal predictor algorithms which match them to the geometry of known 2D material templates via element substitution. Two different machine learning-based relaxers then optimize the atomic coordinates of the predicted structures. Finally, density functional theory calculations check if the materials are thermodynamically stable.
|
Remarkably, this automated pipeline found four novel 2D compounds – NiCl4, IrSBr, CuBr3, CoBrCl – confirmed to be thermodynamically stable with density functional theory. Two others, GaBrO and NbBrCl3, exhibit semi-stability very close to ideal values. Researchers also validated the dynamic stability of CuBr3 and GaBrO by demonstrating no imaginary phonon modes. This indicates both can sustain atomic vibrations without breaking down.
|
“The consistent discovery of realistic materials proves these AI techniques can recapitulate human chemical intuition and scientific knowledge to some extent,” Hu says. “But they also explore combinatorics beyond what any human can conceive of.” Notably, CuBr3 and GaBrO have simple structures in hindsight. But nothing in the scientific literature indicated their viability before now.
|
Compared to earlier attempts at AI-driven materials discovery, MTG’s great innovation lies in its end-to-end integration. “Each component individually has precedents in literature,” explains Hu. “Our breakthrough lies in connecting composition, structure prediction, relaxation, and verification with machine learning models.” The researchers benchmarked variants of MTG minus any one component and found greatly reduced discovery rates, proving integration is key.
|
This technology could massively expand the palette of 2D materials available to design next-generation devices. But before wide-scale practical adoption, researchers need to test synthesizing some of the proposed compounds in physical labs. “The ultimate validation requires experimental demonstration,” says Hu. “Now that impetus lies on material chemists to realize the leads highlighted by simulation.”
|
If synthesis succeeds, MTG could initiate an AI-accelerated wave of new 2D materials tailored to specific industrial and scientific applications. Its neural architecture also provides a blueprint to automate discovery in related domains like catalysts, polymers, and quantum materials. “We envision autonomous labs run by artificial scientists continuously proposing and assessing new compounds,” concludes Hu. “This work helps bridge that ambitious long-term vision.”
|
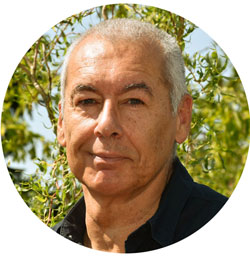
By
Michael
Berger
– Michael is author of three books by the Royal Society of Chemistry: Nano-Society: Pushing the Boundaries of Technology, Nanotechnology: The Future is Tiny, and Nanoengineering: The Skills and Tools Making Technology Invisible Copyright ©
Nanowerk LLC
|
|
- SEO Powered Content & PR Distribution. Get Amplified Today.
- PlatoData.Network Vertical Generative Ai. Empower Yourself. Access Here.
- PlatoAiStream. Web3 Intelligence. Knowledge Amplified. Access Here.
- PlatoESG. Carbon, CleanTech, Energy, Environment, Solar, Waste Management. Access Here.
- PlatoHealth. Biotech and Clinical Trials Intelligence. Access Here.
- Source: https://www.nanowerk.com/spotlight/spotid=64313.php