ऑर्डर देने के लिए एक-पर-एक ग्राहक बातचीत के आज के परिदृश्य में, प्रचलित प्रथा मानव परिचारकों पर निर्भर रहना जारी रखती है, यहां तक कि ड्राइव-थ्रू कॉफी शॉप और फास्ट-फूड प्रतिष्ठानों जैसी सेटिंग्स में भी। यह पारंपरिक दृष्टिकोण कई चुनौतियाँ पेश करता है: यह मैन्युअल प्रक्रियाओं पर बहुत अधिक निर्भर करता है, ग्राहकों की बढ़ती माँगों के साथ कुशलतापूर्वक स्केल करने के लिए संघर्ष करता है, मानवीय त्रुटियों की संभावना का परिचय देता है, और उपलब्धता के विशिष्ट घंटों के भीतर काम करता है। इसके अतिरिक्त, प्रतिस्पर्धी बाजारों में, केवल मैन्युअल प्रक्रियाओं का पालन करने वाले व्यवसायों को कुशल और प्रतिस्पर्धी सेवा प्रदान करना चुनौतीपूर्ण हो सकता है। तकनीकी प्रगति के बावजूद, मानव-केंद्रित मॉडल ऑर्डर प्रोसेसिंग में गहराई से समाया हुआ है, जिससे ये सीमाएँ पैदा होती हैं।
एक-पर-एक ऑर्डर प्रोसेसिंग सहायता के लिए प्रौद्योगिकी का उपयोग करने की संभावना कुछ समय से उपलब्ध है। हालाँकि, मौजूदा समाधान अक्सर दो श्रेणियों में आ सकते हैं: नियम-आधारित सिस्टम जो सेटअप और रखरखाव के लिए पर्याप्त समय और प्रयास की मांग करते हैं, या कठोर सिस्टम जिनमें ग्राहकों के साथ मानव-जैसी बातचीत के लिए आवश्यक लचीलेपन की कमी होती है। परिणामस्वरूप, व्यवसायों और संगठनों को ऐसे समाधानों को तेजी से और कुशलता से लागू करने में चुनौतियों का सामना करना पड़ता है। सौभाग्य से, के आगमन के साथ जनरेटिव ए.आई. और बड़े भाषा मॉडल (एलएलएम), अब स्वचालित सिस्टम बनाना संभव है जो प्राकृतिक भाषा को कुशलतापूर्वक और त्वरित ऑन-रैंप टाइमलाइन के साथ संभाल सके।
अमेज़ॅन बेडरॉक एक पूरी तरह से प्रबंधित सेवा है जो एक ही एपीआई के माध्यम से एआई21 लैब्स, एंथ्रोपिक, कोहेयर, मेटा, स्टेबिलिटी एआई और अमेज़ॅन जैसी अग्रणी एआई कंपनियों से उच्च प्रदर्शन वाले फाउंडेशन मॉडल (एफएम) का विकल्प प्रदान करती है, साथ ही आपको क्षमताओं का एक व्यापक सेट भी प्रदान करती है। सुरक्षा, गोपनीयता और जिम्मेदार AI के साथ जेनेरिक AI एप्लिकेशन बनाने की आवश्यकता है। अमेज़ॅन बेडरॉक के अलावा, आप अन्य AWS सेवाओं का उपयोग कर सकते हैं जैसे अमेज़न SageMaker जम्पस्टार्ट और अमेज़न लेक्स पूरी तरह से स्वचालित और आसानी से अनुकूलनीय जेनरेटिव एआई ऑर्डर प्रोसेसिंग एजेंट बनाने के लिए।
इस पोस्ट में, हम आपको दिखाते हैं कि अमेज़ॅन लेक्स, अमेज़ॅन बेडरॉक और का उपयोग करके भाषण-सक्षम ऑर्डर प्रोसेसिंग एजेंट कैसे बनाया जाए AWS लाम्बा.
समाधान अवलोकन
निम्नलिखित चित्र हमारे समाधान वास्तुकला को दर्शाता है।
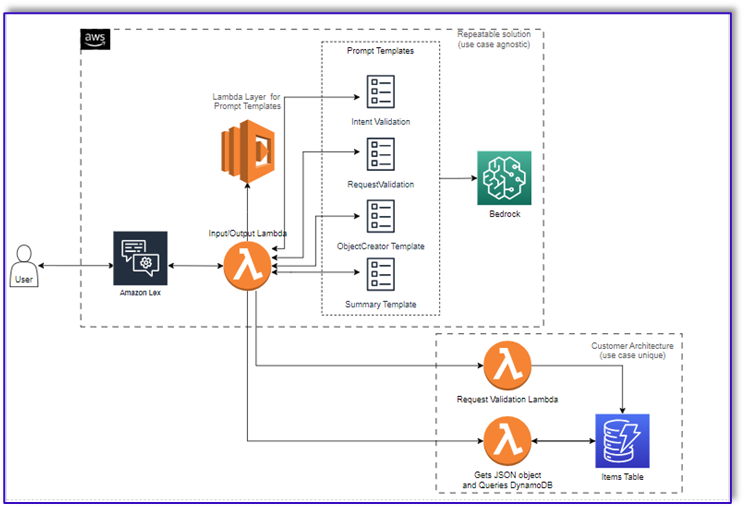
वर्कफ़्लो में निम्नलिखित चरण होते हैं:
- एक ग्राहक Amazon Lex का उपयोग करके ऑर्डर देता है।
- अमेज़ॅन लेक्स बॉट ग्राहक के इरादों की व्याख्या करता है और ट्रिगर करता है
DialogCodeHook
.
- लैम्ब्डा फ़ंक्शन लैम्ब्डा परत से उपयुक्त प्रॉम्प्ट टेम्पलेट खींचता है और संबंधित प्रॉम्प्ट टेम्पलेट में ग्राहक इनपुट जोड़कर मॉडल प्रॉम्प्ट को प्रारूपित करता है।
- RSI
RequestValidation
प्रॉम्प्ट मेनू आइटम के साथ ऑर्डर को सत्यापित करता है और ग्राहक को अमेज़ॅन लेक्स के माध्यम से बताता है कि क्या ऐसा कुछ है जिसे वे ऑर्डर करना चाहते हैं जो मेनू का हिस्सा नहीं है और सिफारिशें प्रदान करेगा। प्रॉम्प्ट ऑर्डर पूर्णता के लिए प्रारंभिक सत्यापन भी करता है।
- RSI
ObjectCreator
प्रॉम्प्ट प्राकृतिक भाषा अनुरोधों को डेटा संरचना (JSON प्रारूप) में परिवर्तित करता है।
- ग्राहक सत्यापनकर्ता लैम्ब्डा फ़ंक्शन ऑर्डर के लिए आवश्यक विशेषताओं का सत्यापन करता है और पुष्टि करता है कि ऑर्डर को संसाधित करने के लिए सभी आवश्यक जानकारी मौजूद है या नहीं।
- एक ग्राहक लैम्ब्डा फ़ंक्शन ऑर्डर को संसाधित करने के लिए इनपुट के रूप में डेटा संरचना लेता है और कुल ऑर्डर को ऑर्केस्ट्रेटिंग लैम्ब्डा फ़ंक्शन में वापस भेज देता है।
- ऑर्केस्ट्रेटिंग लैम्ब्डा फ़ंक्शन ग्राहक डेटाबेस सिस्टम से ऑर्डर कुल सहित अंतिम ऑर्डर सारांश उत्पन्न करने के लिए अमेज़ॅन बेडरॉक एलएलएम एंडपॉइंट को कॉल करता है (उदाहरण के लिए, अमेज़ॅन डायनेमोडीबी).
- ऑर्डर सारांश अमेज़ॅन लेक्स के माध्यम से ग्राहक को वापस सूचित किया जाता है। ग्राहक द्वारा ऑर्डर की पुष्टि करने के बाद, ऑर्डर संसाधित किया जाएगा।
.. पूर्वापेक्षाएँ
यह पोस्ट मानती है कि आपके पास एक सक्रिय AWS खाता है और आप निम्नलिखित अवधारणाओं और सेवाओं से परिचित हैं:
इसके अलावा, लैम्ब्डा फ़ंक्शंस से अमेज़ॅन बेडरॉक तक पहुंचने के लिए, आपको यह सुनिश्चित करना होगा कि लैम्ब्डा रनटाइम में निम्नलिखित लाइब्रेरी हैं:
- boto3>=1.28.57
- awscli>=1.29.57
- बोटोकोर>=1.31.57
यह एक के साथ किया जा सकता है लैम्ब्डा परत या आवश्यक पुस्तकालयों के साथ एक विशिष्ट एएमआई का उपयोग करके।
इसके अलावा, अमेज़ॅन बेडरॉक एपीआई को कॉल करते समय इन लाइब्रेरी की आवश्यकता होती है अमेज़ॅन सैजमेकर स्टूडियो. यह निम्नलिखित कोड के साथ एक सेल चलाकर किया जा सकता है:
%pip install --no-build-isolation --force-reinstall
"boto3>=1.28.57"
"awscli>=1.29.57"
"botocore>=1.31.57"
अंत में, आप निम्नलिखित नीति बनाते हैं और बाद में इसे अमेज़ॅन बेडरॉक तक पहुंचने वाली किसी भी भूमिका से जोड़ते हैं:
{
"Version": "2012-10-17",
"Statement": [
{
"Sid": "Statement1",
"Effect": "Allow",
"Action": "bedrock:*",
"Resource": "*"
}
]
}
एक DynamoDB तालिका बनाएँ
हमारे विशिष्ट परिदृश्य में, हमने अपने ग्राहक डेटाबेस सिस्टम के रूप में एक DynamoDB तालिका बनाई है, लेकिन आप इसका भी उपयोग कर सकते हैं अमेज़न रिलेशनल डेटाबेस सर्विस (अमेज़ॅन आरडीएस)। अपनी DynamoDB तालिका को व्यवस्थित करने के लिए निम्नलिखित चरणों को पूरा करें (या अपने उपयोग के मामले के लिए आवश्यकतानुसार सेटिंग्स को अनुकूलित करें):
- डायनॉम्बीडी कंसोल पर, चुनें टेबल्स नेविगेशन फलक में
- चुनें तालिका बनाएं.
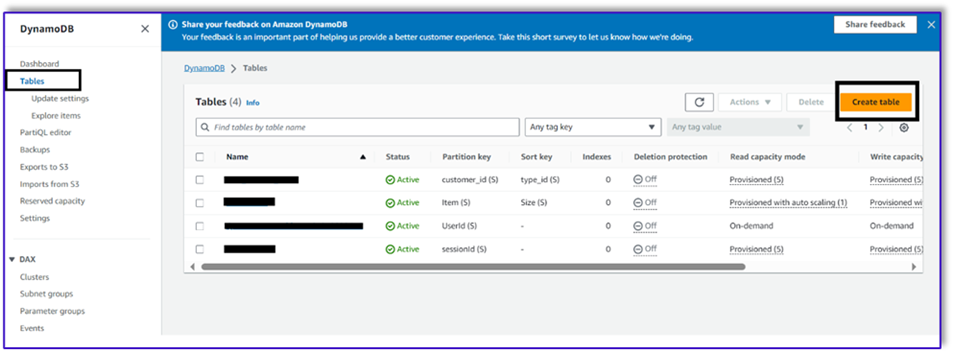
- के लिए तालिका नाम, एक नाम दर्ज करें (उदाहरण के लिए,
ItemDetails
).
- के लिए विभाजन कुंजी, एक कुंजी दर्ज करें (इस पोस्ट के लिए, हम इसका उपयोग करते हैं
Item
).
- के लिए क्रमबद्ध कुंजी, एक कुंजी दर्ज करें (इस पोस्ट के लिए, हम इसका उपयोग करते हैं
Size
).
- चुनें तालिका बनाएं.

अब आप डेटा को DynamoDB तालिका में लोड कर सकते हैं। इस पोस्ट के लिए, हम एक CSV फ़ाइल का उपयोग करते हैं। आप SageMaker नोटबुक में Python कोड का उपयोग करके डेटा को DynamoDB तालिका में लोड कर सकते हैं।
सबसे पहले, हमें देव नामक एक प्रोफ़ाइल स्थापित करने की आवश्यकता है।
- सेजमेकर स्टूडियो में एक नया टर्मिनल खोलें और निम्नलिखित कमांड चलाएँ:
aws configure --profile dev
यह कमांड आपको अपनी AWS एक्सेस कुंजी आईडी, गुप्त एक्सेस कुंजी, डिफ़ॉल्ट AWS क्षेत्र और आउटपुट प्रारूप दर्ज करने के लिए प्रेरित करेगा।
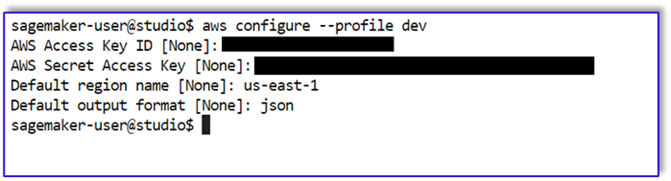
- SageMaker नोटबुक पर वापस लौटें और Python में Boto3 लाइब्रेरी का उपयोग करके DynamoDB से कनेक्शन स्थापित करने के लिए Python कोड लिखें। यह कोड स्निपेट देव नामक एक विशिष्ट AWS प्रोफ़ाइल का उपयोग करके एक सत्र बनाता है और फिर उस सत्र का उपयोग करके एक DynamoDB क्लाइंट बनाता है। डेटा लोड करने के लिए कोड नमूना निम्नलिखित है:
%pip install boto3
import boto3
import csv
# Create a session using a profile named 'dev'
session = boto3.Session(profile_name='dev')
# Create a DynamoDB resource using the session
dynamodb = session.resource('dynamodb')
# Specify your DynamoDB table name
table_name = 'your_table_name'
table = dynamodb.Table(table_name)
# Specify the path to your CSV file
csv_file_path = 'path/to/your/file.csv'
# Read CSV file and put items into DynamoDB
with open(csv_file_path, 'r', encoding='utf-8-sig') as csvfile:
csvreader = csv.reader(csvfile)
# Skip the header row
next(csvreader, None)
for row in csvreader:
# Extract values from the CSV row
item = {
'Item': row[0], # Adjust the index based on your CSV structure
'Size': row[1],
'Price': row[2]
}
# Put item into DynamoDB
response = table.put_item(Item=item)
print(f"Item added: {response}")
print(f"CSV data has been loaded into the DynamoDB table: {table_name}")
वैकल्पिक रूप से, आप उपयोग कर सकते हैं नोएसक्यूएल कार्यक्षेत्र या अन्य उपकरण डेटा को आपकी DynamoDB तालिका में शीघ्रता से लोड करने के लिए।
तालिका में नमूना डेटा डालने के बाद निम्नलिखित एक स्क्रीनशॉट है।
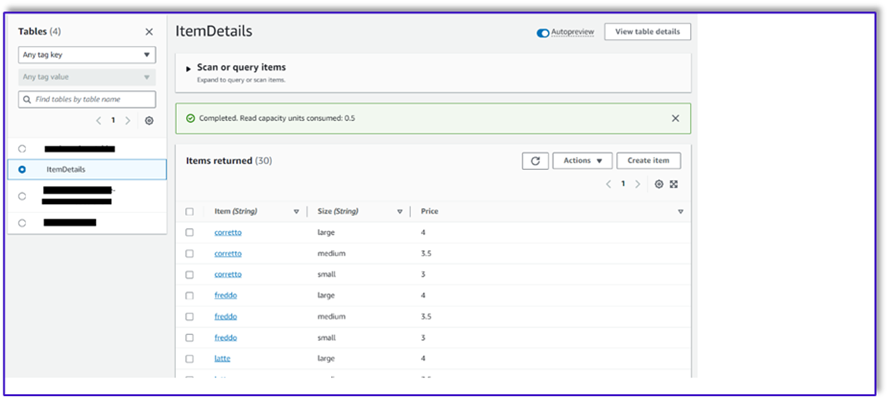
अमेज़ॅन बेडरॉक इनवोकेशन एपीआई का उपयोग करके सेजमेकर नोटबुक में टेम्पलेट बनाएं
इस उपयोग के मामले के लिए अपना त्वरित टेम्पलेट बनाने के लिए, हम अमेज़ॅन बेडरॉक का उपयोग करते हैं। आप अमेज़ॅन बेडरॉक तक पहुंच सकते हैं एडब्ल्यूएस प्रबंधन कंसोल और एपीआई आमंत्रण के माध्यम से। हमारे मामले में, हम न केवल अपना प्रॉम्प्ट टेम्पलेट बनाने के लिए, बल्कि अपना पूरा एपीआई इनवोकेशन कोड बनाने के लिए सेजमेकर स्टूडियो नोटबुक की सुविधा से एपीआई के माध्यम से अमेज़ॅन बेडरॉक तक पहुंचते हैं, जिसे हम बाद में अपने लैम्ब्डा फ़ंक्शन पर उपयोग कर सकते हैं।
- सेजमेकर कंसोल पर, मौजूदा सेजमेकर स्टूडियो डोमेन तक पहुंचें या सेजमेकर नोटबुक से अमेज़ॅन बेडरॉक तक पहुंचने के लिए एक नया डोमेन बनाएं।
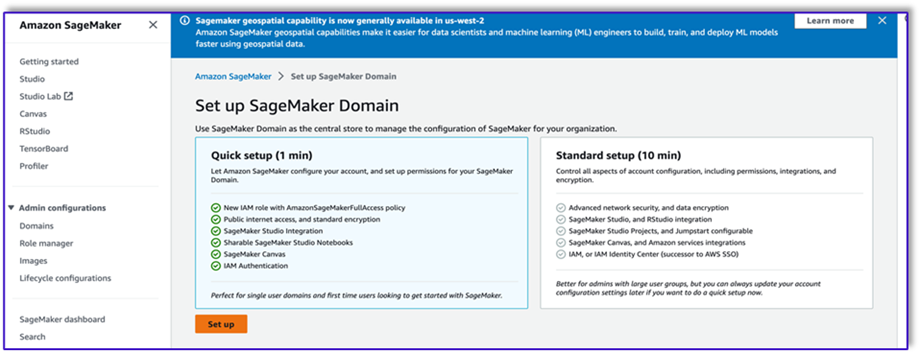
- सेजमेकर डोमेन और उपयोगकर्ता बनाने के बाद, उपयोगकर्ता चुनें और चुनें लांच और स्टूडियो. इससे JupyterLab वातावरण खुल जाएगा।
- जब JupyterLab वातावरण तैयार हो जाए, तो एक नई नोटबुक खोलें और आवश्यक लाइब्रेरी आयात करना शुरू करें।
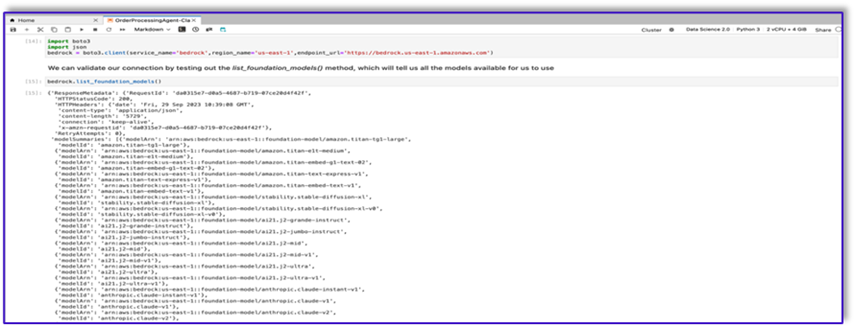
अमेज़ॅन बेडरॉक पायथन एसडीके के माध्यम से कई एफएम उपलब्ध हैं। इस मामले में, हम एंथ्रोपिक द्वारा विकसित एक शक्तिशाली मूलभूत मॉडल क्लाउड V2 का उपयोग करते हैं।
ऑर्डर प्रोसेसिंग एजेंट को कुछ अलग टेम्पलेट की आवश्यकता होती है। यह उपयोग के मामले के आधार पर बदल सकता है, लेकिन हमने एक सामान्य वर्कफ़्लो डिज़ाइन किया है जो कई सेटिंग्स पर लागू हो सकता है। इस उपयोग के मामले के लिए, अमेज़ॅन बेडरॉक एलएलएम टेम्पलेट निम्नलिखित पूरा करेगा:
- ग्राहक के इरादे को मान्य करें
- अनुरोध मान्य करें
- ऑर्डर डेटा संरचना बनाएं
- ग्राहक को ऑर्डर का सारांश भेजें
- मॉडल को लागू करने के लिए, Boto3 से एक बेडरॉक-रनटाइम ऑब्जेक्ट बनाएं।

#Model api request parameters
modelId = 'anthropic.claude-v2' # change this to use a different version from the model provider
accept = 'application/json'
contentType = 'application/json'
import boto3
import json
bedrock = boto3.client(service_name='bedrock-runtime')
आइए इंटेंट वैलिडेटर प्रॉम्प्ट टेम्पलेट पर काम करके शुरुआत करें। यह एक पुनरावृत्तीय प्रक्रिया है, लेकिन एंथ्रोपिक के प्रॉम्प्ट इंजीनियरिंग गाइड के लिए धन्यवाद, आप जल्दी से एक प्रॉम्प्ट बना सकते हैं जो कार्य को पूरा कर सकता है।
- एक उपयोगिता फ़ंक्शन के साथ पहला प्रॉम्प्ट टेम्पलेट बनाएं जो एपीआई इनवोकेशन के लिए बॉडी तैयार करने में मदद करेगा।
Prompt_template_intent_validator.txt के लिए कोड निम्नलिखित है:
"{"prompt": "Human: I will give you some instructions to complete my request.n<instructions>Given the Conversation between Human and Assistant, you need to identify the intent that the human wants to accomplish and respond appropriately. The valid intents are: Greeting,Place Order, Complain, Speak to Someone. Always put your response to the Human within the Response tags. Also add an XML tag to your output identifying the human intent.nHere are some examples:n<example><Conversation> H: hi there.nnA: Hi, how can I help you today?nnH: Yes. I would like a medium mocha please</Conversation>nnA:<intent>Place Order</intent><Response>nGot it.</Response></example>n<example><Conversation> H: hellonnA: Hi, how can I help you today?nnH: my coffee does not taste well can you please re-make it?</Conversation>nnA:<intent>Complain</intent><Response>nOh, I am sorry to hear that. Let me get someone to help you.</Response></example>n<example><Conversation> H: hinnA: Hi, how can I help you today?nnH: I would like to speak to someone else please</Conversation>nnA:<intent>Speak to Someone</intent><Response>nSure, let me get someone to help you.</Response></example>n<example><Conversation> H: howdynnA: Hi, how can I help you today?nnH:can I get a large americano with sugar and 2 mochas with no whipped cream</Conversation>nnA:<intent>Place Order</intent><Response>nSure thing! Please give me a moment.</Response></example>n<example><Conversation> H: hinn</Conversation>nnA:<intent>Greeting</intent><Response>nHi there, how can I help you today?</Response></example>n</instructions>nnPlease complete this request according to the instructions and examples provided above:<request><Conversation>REPLACEME</Conversation></request>nnAssistant:n", "max_tokens_to_sample": 250, "temperature": 1, "top_k": 250, "top_p": 0.75, "stop_sequences": ["nnHuman:", "nnhuman:", "nnCustomer:", "nncustomer:"]}"
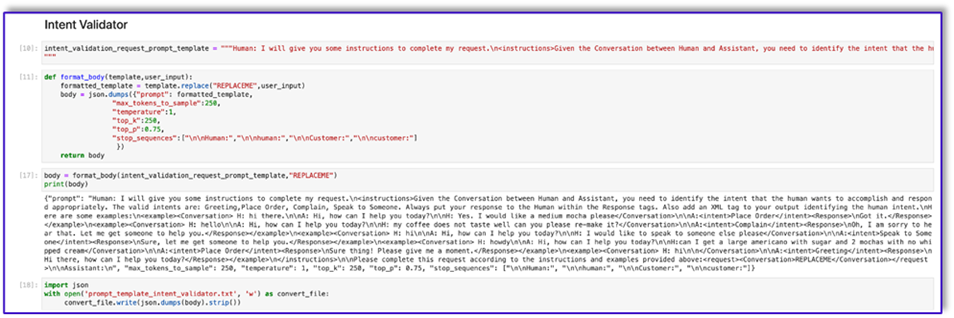

- Amazon S3 पर अपलोड करने और आवश्यकता पड़ने पर लैम्ब्डा फ़ंक्शन से कॉल करने के लिए इस टेम्पलेट को एक फ़ाइल में सहेजें। टेम्प्लेट को टेक्स्ट फ़ाइल में JSON क्रमबद्ध स्ट्रिंग के रूप में सहेजें। पिछला स्क्रीनशॉट इसे पूरा करने के लिए कोड नमूना भी दिखाता है।
- अन्य टेम्पलेट्स के साथ भी यही चरण दोहराएँ।
अन्य टेम्प्लेट के कुछ स्क्रीनशॉट और उनमें से कुछ के साथ अमेज़ॅन बेडरॉक पर कॉल करने पर परिणाम निम्नलिखित हैं।
Prompt_template_request_validator.txt के लिए कोड निम्नलिखित है:
"{"prompt": "Human: I will give you some instructions to complete my request.n<instructions>Given the context do the following steps: 1. verify that the items in the input are valid. If customer provided an invalid item, recommend replacing it with a valid one. 2. verify that the customer has provided all the information marked as required. If the customer missed a required information, ask the customer for that information. 3. When the order is complete, provide a summary of the order and ask for confirmation always using this phrase: 'is this correct?' 4. If the customer confirms the order, Do not ask for confirmation again, just say the phrase inside the brackets [Great, Give me a moment while I try to process your order]</instructions>n<context>nThe VALID MENU ITEMS are: [latte, frappe, mocha, espresso, cappuccino, romano, americano].nThe VALID OPTIONS are: [splenda, stevia, raw sugar, honey, whipped cream, sugar, oat milk, soy milk, regular milk, skimmed milk, whole milk, 2 percent milk, almond milk].nThe required information is: size. Size can be: small, medium, large.nHere are some examples: <example>H: I would like a medium latte with 1 Splenda and a small romano with no sugar please.nnA: <Validation>:nThe Human is ordering a medium latte with one splenda. Latte is a valid menu item and splenda is a valid option. The Human is also ordering a small romano with no sugar. Romano is a valid menu item.</Validation>n<Response>nOk, I got: nt-Medium Latte with 1 Splenda and.nt-Small Romano with no Sugar.nIs this correct?</Response>nnH: yep.nnA:n<Response>nGreat, Give me a moment while I try to process your order</example>nn<example>H: I would like a cappuccino and a mocha please.nnA: <Validation>:nThe Human is ordering a cappuccino and a mocha. Both are valid menu items. The Human did not provide the size for the cappuccino. The human did not provide the size for the mocha. I will ask the Human for the required missing information.</Validation>n<Response>nSure thing, but can you please let me know the size for the Cappuccino and the size for the Mocha? We have Small, Medium, or Large.</Response></example>nn<example>H: I would like a small cappuccino and a large lemonade please.nnA: <Validation>:nThe Human is ordering a small cappuccino and a large lemonade. Cappuccino is a valid menu item. Lemonade is not a valid menu item. I will suggest the Human a replacement from our valid menu items.</Validation>n<Response>nSorry, we don't have Lemonades, would you like to order something else instead? Perhaps a Frappe or a Latte?</Response></example>nn<example>H: Can I get a medium frappuccino with sugar please?nnA: <Validation>:n The Human is ordering a Frappuccino. Frappuccino is not a valid menu item. I will suggest a replacement from the valid menu items in my context.</Validation>n<Response>nI am so sorry, but Frappuccino is not in our menu, do you want a frappe or a cappuccino instead? perhaps something else?</Response></example>nn<example>H: I want two large americanos and a small latte please.nnA: <Validation>:n The Human is ordering 2 Large Americanos, and a Small Latte. Americano is a valid menu item. Latte is a valid menu item.</Validation>n<Response>nOk, I got: nt-2 Large Americanos and.nt-Small Latte.nIs this correct?</Response>nnH: looks correct, yes.nnA:n<Response>nGreat, Give me a moment while I try to process your order.</Response></example>nn</Context>nnPlease complete this request according to the instructions and examples provided above:<request>REPLACEME</request>nnAssistant:n", "max_tokens_to_sample": 250, "temperature": 0.3, "top_k": 250, "top_p": 0.75, "stop_sequences": ["nnHuman:", "nnhuman:", "nnCustomer:", "nncustomer:"]}"
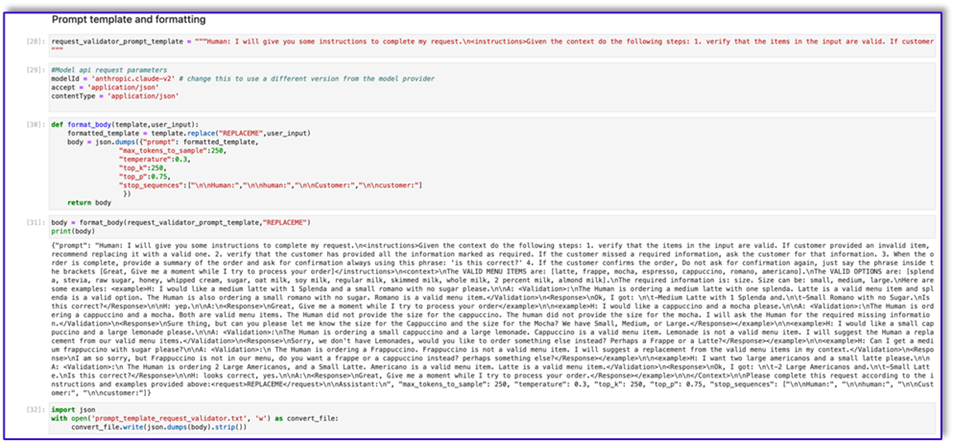
इस टेम्पलेट का उपयोग करते हुए अमेज़ॅन बेडरॉक से हमारी प्रतिक्रिया निम्नलिखित है।

निम्नलिखित के लिए कोड है: prompt_template_object_creator.txt
:
"{"prompt": "Human: I will give you some instructions to complete my request.n<instructions>Given the Conversation between Human and Assistant, you need to create a json object in Response with the appropriate attributes.nHere are some examples:n<example><Conversation> H: I want a latte.nnA:nCan I have the size?nnH: Medium.nnA: So, a medium latte.nIs this Correct?nnH: Yes.</Conversation>nnA:<Response>{"1":{"item":"latte","size":"medium","addOns":[]}}</Response></example>n<example><Conversation> H: I want a large frappe and 2 small americanos with sugar.nnA: Okay, let me confirm:nn1 large frappenn2 small americanos with sugarnnIs this correct?nnH: Yes.</Conversation>nnA:<Response>{"1":{"item":"frappe","size":"large","addOns":[]},"2":{"item":"americano","size":"small","addOns":["sugar"]},"3":{"item":"americano","size":"small","addOns":["sugar"]}}</Response>n</example>n<example><Conversation> H: I want a medium americano.nnA: Okay, let me confirm:nn1 medium americanonnIs this correct?nnH: Yes.</Conversation>nnA:<Response>{"1":{"item":"americano","size":"medium","addOns":[]}}</Response></example>n<example><Conversation> H: I want a large latte with oatmilk.nnA: Okay, let me confirm:nnLarge latte with oatmilknnIs this correct?nnH: Yes.</Conversation>nnA:<Response>{"1":{"item":"latte","size":"large","addOns":["oatmilk"]}}</Response></example>n<example><Conversation> H: I want a small mocha with no whipped cream please.nnA: Okay, let me confirm:nnSmall mocha with no whipped creamnnIs this correct?nnH: Yes.</Conversation>nnA:<Response>{"1":{"item":"mocha","size":"small","addOns":["no whipped cream"]}}</Response>nn</example></instructions>nnPlease complete this request according to the instructions and examples provided above:<request><Conversation>REPLACEME</Conversation></request>nnAssistant:n", "max_tokens_to_sample": 250, "temperature": 0.3, "top_k": 250, "top_p": 0.75, "stop_sequences": ["nnHuman:", "nnhuman:", "nnCustomer:", "nncustomer:"]}"

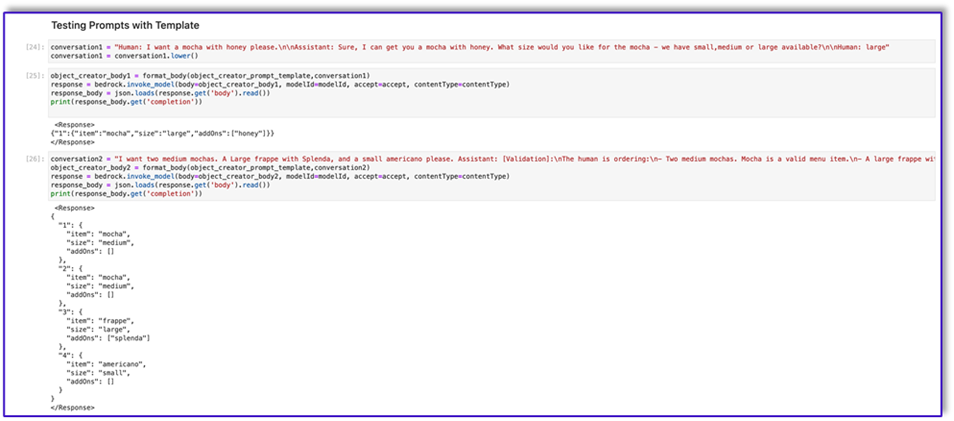
Prompt_template_order_summary.txt के लिए कोड निम्नलिखित है:
"{"prompt": "Human: I will give you some instructions to complete my request.n<instructions>Given the Conversation between Human and Assistant, you need to create a summary of the order with bullet points and include the order total.nHere are some examples:n<example><Conversation> H: I want a large frappe and 2 small americanos with sugar.nnA: Okay, let me confirm:nn1 large frappenn2 small americanos with sugarnnIs this correct?nnH: Yes.</Conversation>nn<OrderTotal>10.50</OrderTotal>nnA:<Response>nHere is a summary of your order along with the total:nn1 large frappenn2 small americanos with sugar.nYour Order total is $10.50</Response></example>n<example><Conversation> H: I want a medium americano.nnA: Okay, let me confirm:nn1 medium americanonnIs this correct?nnH: Yes.</Conversation>nn<OrderTotal>3.50</OrderTotal>nnA:<Response>nHere is a summary of your order along with the total:nn1 medium americano.nYour Order total is $3.50</Response></example>n<example><Conversation> H: I want a large latte with oat milk.nnA: Okay, let me confirm:nnLarge latte with oat milknnIs this correct?nnH: Yes.</Conversation>nn<OrderTotal>6.75</OrderTotal>nnA:<Response>nHere is a summary of your order along with the total:nnLarge latte with oat milk.nYour Order total is $6.75</Response></example>n<example><Conversation> H: I want a small mocha with no whipped cream please.nnA: Okay, let me confirm:nnSmall mocha with no whipped creamnnIs this correct?nnH: Yes.</Conversation>nn<OrderTotal>4.25</OrderTotal>nnA:<Response>nHere is a summary of your order along with the total:nnSmall mocha with no whipped cream.nYour Order total is $6.75</Response>nn</example>n</instructions>nnPlease complete this request according to the instructions and examples provided above:<request><Conversation>REPLACEME</Conversation>nn<OrderTotal>REPLACETOTAL</OrderTotal></request>nnAssistant:n", "max_tokens_to_sample": 250, "temperature": 0.3, "top_k": 250, "top_p": 0.75, "stop_sequences": ["nnHuman:", "nnhuman:", "nnCustomer:", "nncustomer:", "[Conversation]"]}"


जैसा कि आप देख सकते हैं, हमने मेनू आइटम को सत्यापित करने, गुम आवश्यक जानकारी की पहचान करने, डेटा संरचना बनाने और ऑर्डर को सारांशित करने के लिए अपने प्रॉम्प्ट टेम्प्लेट का उपयोग किया है। अमेज़ॅन बेडरॉक पर उपलब्ध मूलभूत मॉडल बहुत शक्तिशाली हैं, इसलिए आप इन टेम्पलेट्स के माध्यम से और भी अधिक कार्य पूरा कर सकते हैं।
आपने संकेतों की इंजीनियरिंग पूरी कर ली है और टेम्प्लेट को टेक्स्ट फ़ाइलों में सहेज लिया है। अब आप अमेज़ॅन लेक्स बॉट और संबंधित लैम्ब्डा फ़ंक्शन बनाना शुरू कर सकते हैं।
शीघ्र टेम्पलेट्स के साथ एक लैम्ब्डा परत बनाएं
अपनी लैम्ब्डा परत बनाने के लिए निम्नलिखित चरणों को पूरा करें:
- सेजमेकर स्टूडियो में, नाम के सबफ़ोल्डर के साथ एक नया फ़ोल्डर बनाएं
python
.
- अपनी प्रॉम्प्ट फ़ाइलों को इसमें कॉपी करें
python
फ़ोल्डर.

- आप निम्न आदेश चलाकर ज़िप लाइब्रेरी को अपने नोटबुक इंस्टेंस में जोड़ सकते हैं।
!conda install -y -c conda-forge zip
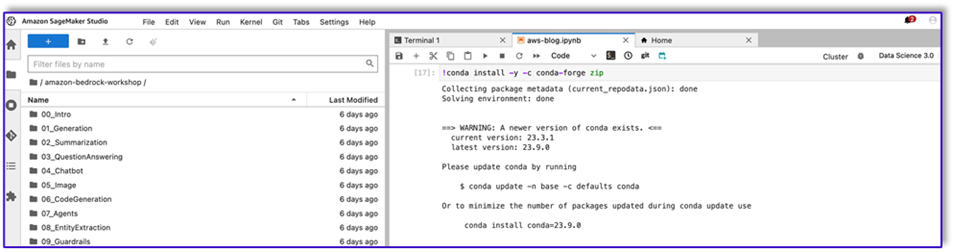
- अब, लैम्ब्डा लेयर पर अपलोड करने के लिए ज़िप फ़ाइल बनाने के लिए निम्न कमांड चलाएँ।
!zip -r prompt_templates_layer.zip prompt_templates_layer/.
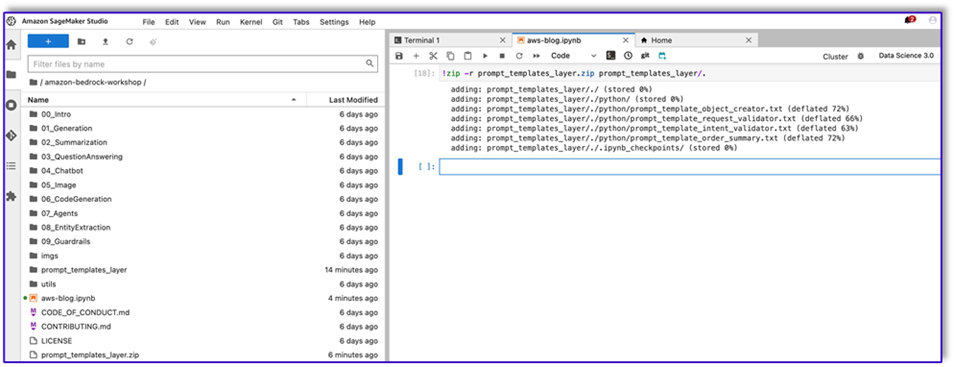
- ज़िप फ़ाइल बनाने के बाद, आप फ़ाइल डाउनलोड कर सकते हैं। लैम्ब्डा पर जाएँ, फ़ाइल को सीधे अपलोड करके या पहले अमेज़न S3 पर अपलोड करके एक नई परत बनाएँ।
- फिर इस नई परत को ऑर्केस्ट्रेशन लैम्ब्डा फ़ंक्शन से जोड़ें।
अब आपकी प्रॉम्प्ट टेम्प्लेट फ़ाइलें आपके लैम्ब्डा रनटाइम वातावरण में स्थानीय रूप से संग्रहीत हैं। यह आपके बॉट रन के दौरान प्रक्रिया को तेज़ कर देगा।
आवश्यक लाइब्रेरीज़ के साथ एक लैम्ब्डा परत बनाएं
आवश्यक पुस्तकालयों के साथ अपनी लैम्ब्डा परत बनाने के लिए निम्नलिखित चरणों को पूरा करें:
- एक खोलें AWS क्लाउड 9 उदाहरण परिवेश, नामक सबफ़ोल्डर के साथ एक फ़ोल्डर बनाएँ
python
.
- के अंदर एक टर्मिनल खोलें
python
फ़ोल्डर.
- टर्मिनल से निम्नलिखित कमांड चलाएँ:
pip install “boto3>=1.28.57” -t .
pip install “awscli>=1.29.57" -t .
pip install “botocore>=1.31.57” -t .
- रन
cd ..
और अपने आप को अपने नए फ़ोल्डर के अंदर रखें जहां आपके पास भी है python
सबफ़ोल्डर।
- निम्न कमांड चलाएं:
- ज़िप फ़ाइल बनाने के बाद, आप फ़ाइल डाउनलोड कर सकते हैं। लैम्ब्डा पर जाएँ, फ़ाइल को सीधे अपलोड करके या पहले अमेज़न S3 पर अपलोड करके एक नई परत बनाएँ।
- फिर इस नई परत को ऑर्केस्ट्रेशन लैम्ब्डा फ़ंक्शन से जोड़ें।
Amazon Lex v2 में बॉट बनाएं
इस उपयोग के मामले के लिए, हम एक अमेज़ॅन लेक्स बॉट बनाते हैं जो किसी भी इंटरफ़ेस से आवाज या टेक्स्ट का उपयोग करके अमेज़ॅन बेडरॉक को कॉल करने के लिए आर्किटेक्चर के लिए एक इनपुट/आउटपुट इंटरफ़ेस प्रदान कर सकता है। क्योंकि एलएलएम इस ऑर्डर प्रोसेसिंग एजेंट के वार्तालाप भाग को संभालेगा, और लैम्ब्डा वर्कफ़्लो को व्यवस्थित करेगा, आप तीन इरादों और बिना स्लॉट के एक बॉट बना सकते हैं।
- अमेज़ॅन लेक्स कंसोल पर, विधि के साथ एक नया बॉट बनाएं एक खाली बॉट बनाएं.
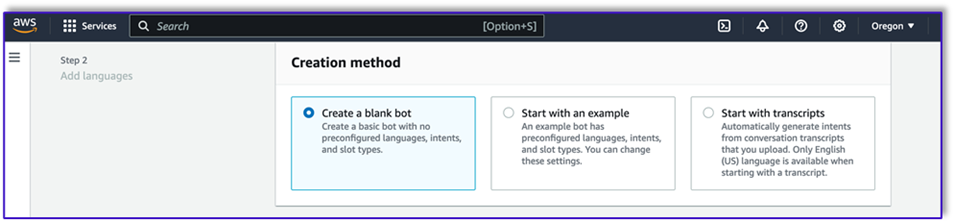
अब आप अंतिम उपयोगकर्ताओं के लिए बॉट के साथ बातचीत शुरू करने के लिए किसी भी उचित प्रारंभिक कथन के साथ एक आशय जोड़ सकते हैं। हम सरल अभिवादन का उपयोग करते हैं और प्रारंभिक बॉट प्रतिक्रिया जोड़ते हैं ताकि अंतिम उपयोगकर्ता अपने अनुरोध प्रदान कर सकें। बॉट बनाते समय, इरादों के साथ लैम्ब्डा कोड हुक का उपयोग करना सुनिश्चित करें; यह एक लैम्ब्डा फ़ंक्शन को ट्रिगर करेगा जो ग्राहक, अमेज़ॅन लेक्स और एलएलएम के बीच वर्कफ़्लो को व्यवस्थित करेगा।
- अपना पहला इरादा जोड़ें, जो वर्कफ़्लो को ट्रिगर करता है और अमेज़ॅन बेडरॉक को कॉल करने और ग्राहक क्या हासिल करने की कोशिश कर रहा है, इसकी पहचान करने के लिए इरादे सत्यापन प्रॉम्प्ट टेम्पलेट का उपयोग करता है। अंतिम उपयोगकर्ताओं के लिए बातचीत शुरू करने के लिए कुछ सरल कथन जोड़ें।

आपको किसी भी बॉट इरादे में किसी स्लॉट या प्रारंभिक रीडिंग का उपयोग करने की आवश्यकता नहीं है। वास्तव में, आपको दूसरे या तीसरे आशय में कथन जोड़ने की आवश्यकता नहीं है। ऐसा इसलिए है क्योंकि एलएलएम पूरी प्रक्रिया के दौरान लैम्ब्डा का मार्गदर्शन करेगा।
- एक पुष्टिकरण संकेत जोड़ें. आप इस संदेश को बाद में लैम्ब्डा फ़ंक्शन में कस्टमाइज़ कर सकते हैं।
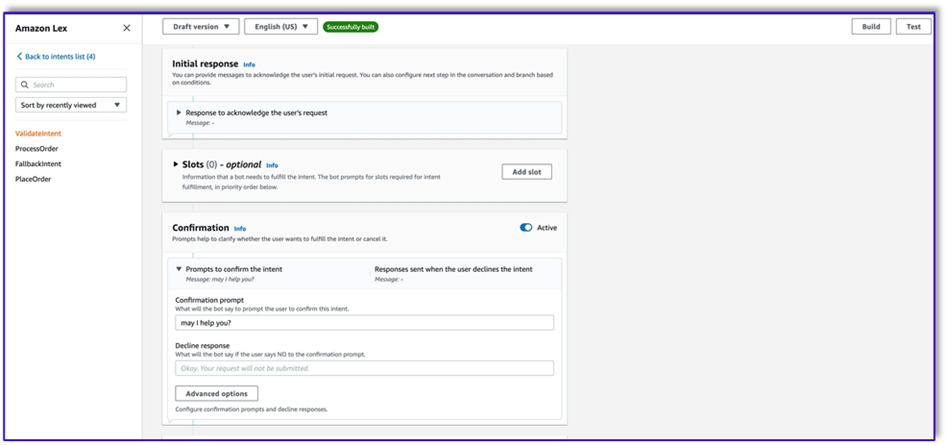
- के अंतर्गत कोड हुक, चुनते हैं आरंभीकरण और सत्यापन के लिए लैम्ब्डा फ़ंक्शन का उपयोग करें.
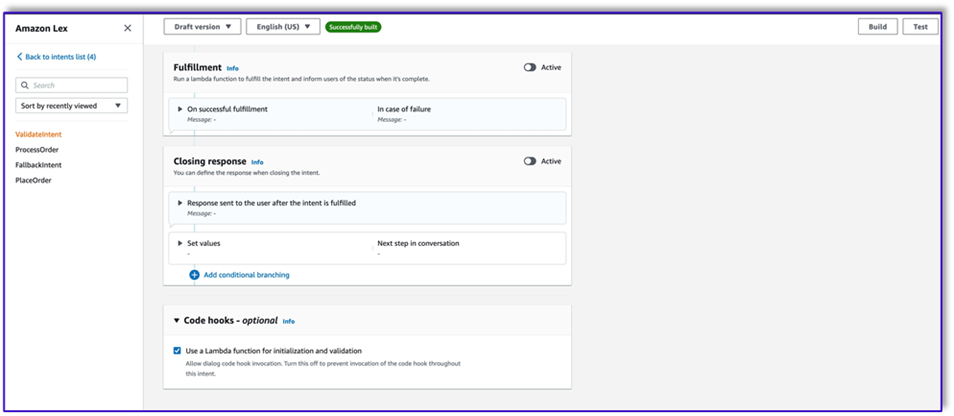
- बिना किसी उच्चारण और बिना किसी प्रारंभिक प्रतिक्रिया के दूसरा इरादा बनाएं। यह है
PlaceOrder
आशय।
जब एलएलएम पहचानता है कि ग्राहक ऑर्डर देने का प्रयास कर रहा है, तो लैम्ब्डा फ़ंक्शन इस इरादे को ट्रिगर करेगा और मेनू के विरुद्ध ग्राहक के अनुरोध को मान्य करेगा, और सुनिश्चित करेगा कि कोई आवश्यक जानकारी गायब नहीं है। याद रखें कि यह सब प्रॉम्प्ट टेम्प्लेट पर है, इसलिए आप प्रॉम्प्ट टेम्प्लेट को बदलकर इस वर्कफ़्लो को किसी भी उपयोग के मामले में अनुकूलित कर सकते हैं।
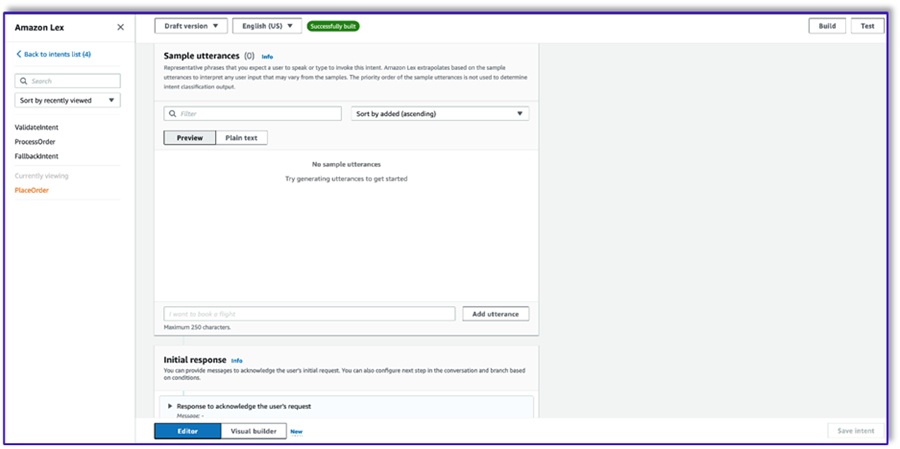
- कोई स्लॉट न जोड़ें, बल्कि एक पुष्टिकरण संकेत और अस्वीकृत प्रतिक्रिया जोड़ें।
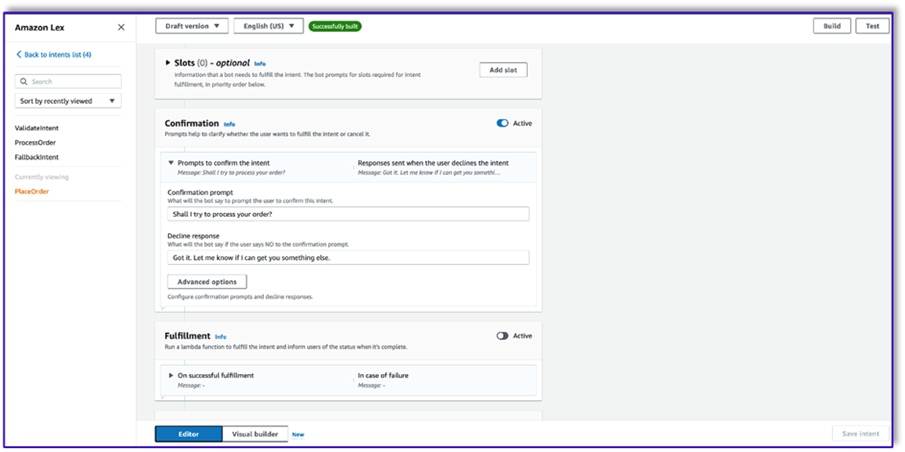
- चुनते हैं आरंभीकरण और सत्यापन के लिए लैम्ब्डा फ़ंक्शन का उपयोग करें.

- नामक तीसरा आशय बनाएँ
ProcessOrder
बिना किसी नमूना कथन और बिना किसी स्लॉट के।
- एक प्रारंभिक प्रतिक्रिया, एक पुष्टिकरण संकेत और एक अस्वीकृति प्रतिक्रिया जोड़ें।
एलएलएम द्वारा ग्राहक के अनुरोध को मान्य करने के बाद, लैम्ब्डा फ़ंक्शन ऑर्डर को संसाधित करने के तीसरे और अंतिम इरादे को ट्रिगर करता है। यहां, लैंबडा डायनेमोडीबी तालिका को क्वेरी करने के लिए ऑर्डर JSON डेटा संरचना उत्पन्न करने के लिए ऑब्जेक्ट क्रिएटर टेम्पलेट का उपयोग करेगा, और फिर कुल ऑर्डर के साथ पूरे ऑर्डर को सारांशित करने के लिए ऑर्डर सारांश टेम्पलेट का उपयोग करेगा ताकि अमेज़ॅन लेक्स इसे ग्राहक तक पहुंचा सके।
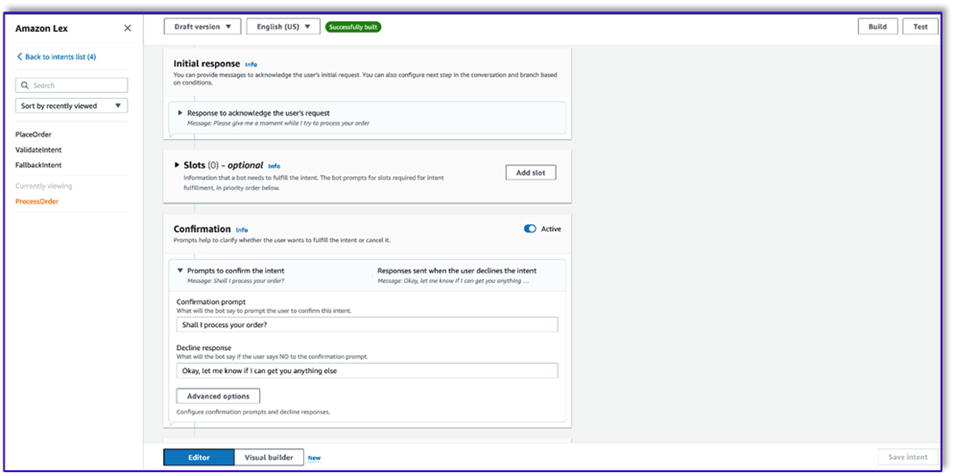
- चुनते हैं आरंभीकरण और सत्यापन के लिए लैम्ब्डा फ़ंक्शन का उपयोग करें. ग्राहक द्वारा अंतिम पुष्टि दिए जाने के बाद ऑर्डर को संसाधित करने के लिए यह किसी भी लैम्ब्डा फ़ंक्शन का उपयोग कर सकता है।

- तीनों इंटेंट बनाने के बाद, विज़ुअल बिल्डर के पास जाएं
ValidateIntent
, एक आशय कदम जोड़ें, और सकारात्मक पुष्टिकरण के आउटपुट को उस चरण से कनेक्ट करें।
- गो-टू इंटेंट जोड़ने के बाद, इसे संपादित करें और इंटेंट नाम के रूप में प्लेसऑर्डर इंटेंट चुनें।

- इसी तरह, विज़ुअल बिल्डर के लिए जाने के लिए
PlaceOrder
आशय और सकारात्मक पुष्टिकरण के आउटपुट को कनेक्ट करें ProcessOrder
जाने का इरादा. के लिए किसी संपादन की आवश्यकता नहीं है ProcessOrder
आशय।
- अब आपको लैम्ब्डा फ़ंक्शन बनाने की आवश्यकता है जो अमेज़ॅन लेक्स को व्यवस्थित करता है और डायनेमोडीबी तालिका को कॉल करता है, जैसा कि निम्नलिखित अनुभाग में बताया गया है।
अमेज़ॅन लेक्स बॉट को व्यवस्थित करने के लिए एक लैम्ब्डा फ़ंक्शन बनाएं
अब आप लैम्ब्डा फ़ंक्शन का निर्माण कर सकते हैं जो अमेज़ॅन लेक्स बॉट और वर्कफ़्लो को व्यवस्थित करता है। निम्नलिखित चरणों को पूरा करें:
- मानक निष्पादन नीति के साथ एक लैम्ब्डा फ़ंक्शन बनाएं और लैम्ब्डा को आपके लिए एक भूमिका बनाने दें।
- अपने फ़ंक्शन की कोड विंडो में, कुछ उपयोगिता फ़ंक्शन जोड़ें जो मदद करेंगे: टेम्पलेट में लेक्स संदर्भ जोड़कर संकेतों को प्रारूपित करें, अमेज़ॅन बेडरॉक एलएलएम एपीआई को कॉल करें, प्रतिक्रियाओं से वांछित पाठ निकालें, और बहुत कुछ। निम्नलिखित कोड देखें:
import json
import re
import boto3
import logging
logger = logging.getLogger()
logger.setLevel(logging.DEBUG)
bedrock = boto3.client(service_name='bedrock-runtime')
def CreatingCustomPromptFromLambdaLayer(object_key,replace_items):
folder_path = '/opt/order_processing_agent_prompt_templates/python/'
try:
file_path = folder_path + object_key
with open(file_path, "r") as file1:
raw_template = file1.read()
# Modify the template with the custom input prompt
#template['inputs'][0].insert(1, {"role": "user", "content": '### Input:n' + user_request})
for key,value in replace_items.items():
value = json.dumps(json.dumps(value).replace('"','')).replace('"','')
raw_template = raw_template.replace(key,value)
modified_prompt = raw_template
return modified_prompt
except Exception as e:
return {
'statusCode': 500,
'body': f'An error occurred: {str(e)}'
}
def CreatingCustomPrompt(object_key,replace_items):
logger.debug('replace_items is: {}'.format(replace_items))
#retrieve user request from intent_request
#we first propmt the model with current order
bucket_name = 'your-bucket-name'
#object_key = 'prompt_template_order_processing.txt'
try:
s3 = boto3.client('s3')
# Retrieve the existing template from S3
response = s3.get_object(Bucket=bucket_name, Key=object_key)
raw_template = response['Body'].read().decode('utf-8')
raw_template = json.loads(raw_template)
logger.debug('raw template is {}'.format(raw_template))
#template_json = json.loads(raw_template)
#logger.debug('template_json is {}'.format(template_json))
#template = json.dumps(template_json)
#logger.debug('template is {}'.format(template))
# Modify the template with the custom input prompt
#template['inputs'][0].insert(1, {"role": "user", "content": '### Input:n' + user_request})
for key,value in replace_items.items():
raw_template = raw_template.replace(key,value)
logger.debug("Replacing: {} nwith: {}".format(key,value))
modified_prompt = json.dumps(raw_template)
logger.debug("Modified template: {}".format(modified_prompt))
logger.debug("Modified template type is: {}".format(print(type(modified_prompt))))
#modified_template_json = json.loads(modified_prompt)
#logger.debug("Modified template json: {}".format(modified_template_json))
return modified_prompt
except Exception as e:
return {
'statusCode': 500,
'body': f'An error occurred: {str(e)}'
}
def validate_intent(intent_request):
logger.debug('starting validate_intent: {}'.format(intent_request))
#retrieve user request from intent_request
user_request = 'Human: ' + intent_request['inputTranscript'].lower()
#getting current context variable
current_session_attributes = intent_request['sessionState']['sessionAttributes']
if len(current_session_attributes) > 0:
full_context = current_session_attributes['fullContext'] + 'nn' + user_request
dialog_context = current_session_attributes['dialogContext'] + 'nn' + user_request
else:
full_context = user_request
dialog_context = user_request
#Preparing validation prompt by adding context to prompt template
object_key = 'prompt_template_intent_validator.txt'
#replace_items = {"REPLACEME":full_context}
#replace_items = {"REPLACEME":dialog_context}
replace_items = {"REPLACEME":dialog_context}
#validation_prompt = CreatingCustomPrompt(object_key,replace_items)
validation_prompt = CreatingCustomPromptFromLambdaLayer(object_key,replace_items)
#Prompting model for request validation
intent_validation_completion = prompt_bedrock(validation_prompt)
intent_validation_completion = re.sub(r'["]','',intent_validation_completion)
#extracting response from response completion and removing some special characters
validation_response = extract_response(intent_validation_completion)
validation_intent = extract_intent(intent_validation_completion)
#business logic depending on intents
if validation_intent == 'Place Order':
return validate_request(intent_request)
elif validation_intent in ['Complain','Speak to Someone']:
##adding session attributes to keep current context
full_context = full_context + 'nn' + intent_validation_completion
dialog_context = dialog_context + 'nnAssistant: ' + validation_response
intent_request['sessionState']['sessionAttributes']['fullContext'] = full_context
intent_request['sessionState']['sessionAttributes']['dialogContext'] = dialog_context
intent_request['sessionState']['sessionAttributes']['customerIntent'] = validation_intent
return close(intent_request['sessionState']['sessionAttributes'],intent_request['sessionState']['intent']['name'],'Fulfilled','Close',validation_response)
if validation_intent == 'Greeting':
##adding session attributes to keep current context
full_context = full_context + 'nn' + intent_validation_completion
dialog_context = dialog_context + 'nnAssistant: ' + validation_response
intent_request['sessionState']['sessionAttributes']['fullContext'] = full_context
intent_request['sessionState']['sessionAttributes']['dialogContext'] = dialog_context
intent_request['sessionState']['sessionAttributes']['customerIntent'] = validation_intent
return close(intent_request['sessionState']['sessionAttributes'],intent_request['sessionState']['intent']['name'],'InProgress','ConfirmIntent',validation_response)
def validate_request(intent_request):
logger.debug('starting validate_request: {}'.format(intent_request))
#retrieve user request from intent_request
user_request = 'Human: ' + intent_request['inputTranscript'].lower()
#getting current context variable
current_session_attributes = intent_request['sessionState']['sessionAttributes']
if len(current_session_attributes) > 0:
full_context = current_session_attributes['fullContext'] + 'nn' + user_request
dialog_context = current_session_attributes['dialogContext'] + 'nn' + user_request
else:
full_context = user_request
dialog_context = user_request
#Preparing validation prompt by adding context to prompt template
object_key = 'prompt_template_request_validator.txt'
replace_items = {"REPLACEME":dialog_context}
#validation_prompt = CreatingCustomPrompt(object_key,replace_items)
validation_prompt = CreatingCustomPromptFromLambdaLayer(object_key,replace_items)
#Prompting model for request validation
request_validation_completion = prompt_bedrock(validation_prompt)
request_validation_completion = re.sub(r'["]','',request_validation_completion)
#extracting response from response completion and removing some special characters
validation_response = extract_response(request_validation_completion)
##adding session attributes to keep current context
full_context = full_context + 'nn' + request_validation_completion
dialog_context = dialog_context + 'nnAssistant: ' + validation_response
intent_request['sessionState']['sessionAttributes']['fullContext'] = full_context
intent_request['sessionState']['sessionAttributes']['dialogContext'] = dialog_context
return close(intent_request['sessionState']['sessionAttributes'],'PlaceOrder','InProgress','ConfirmIntent',validation_response)
def process_order(intent_request):
logger.debug('starting process_order: {}'.format(intent_request))
#retrieve user request from intent_request
user_request = 'Human: ' + intent_request['inputTranscript'].lower()
#getting current context variable
current_session_attributes = intent_request['sessionState']['sessionAttributes']
if len(current_session_attributes) > 0:
full_context = current_session_attributes['fullContext'] + 'nn' + user_request
dialog_context = current_session_attributes['dialogContext'] + 'nn' + user_request
else:
full_context = user_request
dialog_context = user_request
# Preparing object creator prompt by adding context to prompt template
object_key = 'prompt_template_object_creator.txt'
replace_items = {"REPLACEME":dialog_context}
#object_creator_prompt = CreatingCustomPrompt(object_key,replace_items)
object_creator_prompt = CreatingCustomPromptFromLambdaLayer(object_key,replace_items)
#Prompting model for object creation
object_creation_completion = prompt_bedrock(object_creator_prompt)
#extracting response from response completion
object_creation_response = extract_response(object_creation_completion)
inputParams = json.loads(object_creation_response)
inputParams = json.dumps(json.dumps(inputParams))
logger.debug('inputParams is: {}'.format(inputParams))
client = boto3.client('lambda')
response = client.invoke(FunctionName = 'arn:aws:lambda:us-east-1:<AccountNumber>:function:aws-blog-order-validator',InvocationType = 'RequestResponse',Payload = inputParams)
responseFromChild = json.load(response['Payload'])
validationResult = responseFromChild['statusCode']
if validationResult == 205:
order_validation_error = responseFromChild['validator_response']
return close(intent_request['sessionState']['sessionAttributes'],'PlaceOrder','InProgress','ConfirmIntent',order_validation_error)
#invokes Order Processing lambda to query DynamoDB table and returns order total
response = client.invoke(FunctionName = 'arn:aws:lambda:us-east-1: <AccountNumber>:function:aws-blog-order-processing',InvocationType = 'RequestResponse',Payload = inputParams)
responseFromChild = json.load(response['Payload'])
orderTotal = responseFromChild['body']
###Prompting the model to summarize the order along with order total
object_key = 'prompt_template_order_summary.txt'
replace_items = {"REPLACEME":dialog_context,"REPLACETOTAL":orderTotal}
#order_summary_prompt = CreatingCustomPrompt(object_key,replace_items)
order_summary_prompt = CreatingCustomPromptFromLambdaLayer(object_key,replace_items)
order_summary_completion = prompt_bedrock(order_summary_prompt)
#extracting response from response completion
order_summary_response = extract_response(order_summary_completion)
order_summary_response = order_summary_response + '. Shall I finalize processing your order?'
##adding session attributes to keep current context
full_context = full_context + 'nn' + order_summary_completion
dialog_context = dialog_context + 'nnAssistant: ' + order_summary_response
intent_request['sessionState']['sessionAttributes']['fullContext'] = full_context
intent_request['sessionState']['sessionAttributes']['dialogContext'] = dialog_context
return close(intent_request['sessionState']['sessionAttributes'],'ProcessOrder','InProgress','ConfirmIntent',order_summary_response)
""" --- Main handler and Workflow functions --- """
def lambda_handler(event, context):
"""
Route the incoming request based on intent.
The JSON body of the request is provided in the event slot.
"""
logger.debug('event is: {}'.format(event))
return dispatch(event)
def dispatch(intent_request):
"""
Called when the user specifies an intent for this bot. If intent is not valid then returns error name
"""
logger.debug('intent_request is: {}'.format(intent_request))
intent_name = intent_request['sessionState']['intent']['name']
confirmation_state = intent_request['sessionState']['intent']['confirmationState']
# Dispatch to your bot's intent handlers
if intent_name == 'ValidateIntent' and confirmation_state == 'None':
return validate_intent(intent_request)
if intent_name == 'PlaceOrder' and confirmation_state == 'None':
return validate_request(intent_request)
elif intent_name == 'PlaceOrder' and confirmation_state == 'Confirmed':
return process_order(intent_request)
elif intent_name == 'PlaceOrder' and confirmation_state == 'Denied':
return close(intent_request['sessionState']['sessionAttributes'],intent_request['sessionState']['intent']['name'],'Fulfilled','Close','Got it. Let me know if I can help you with something else.')
elif intent_name == 'PlaceOrder' and confirmation_state not in ['Denied','Confirmed','None']:
return close(intent_request['sessionState']['sessionAttributes'],intent_request['sessionState']['intent']['name'],'Fulfilled','Close','Sorry. I am having trouble completing the request. Let me get someone to help you.')
logger.debug('exiting intent {} here'.format(intent_request['sessionState']['intent']['name']))
elif intent_name == 'ProcessOrder' and confirmation_state == 'None':
return validate_request(intent_request)
elif intent_name == 'ProcessOrder' and confirmation_state == 'Confirmed':
return close(intent_request['sessionState']['sessionAttributes'],intent_request['sessionState']['intent']['name'],'Fulfilled','Close','Perfect! Your order has been processed. Please proceed to payment.')
elif intent_name == 'ProcessOrder' and confirmation_state == 'Denied':
return close(intent_request['sessionState']['sessionAttributes'],intent_request['sessionState']['intent']['name'],'Fulfilled','Close','Got it. Let me know if I can help you with something else.')
elif intent_name == 'ProcessOrder' and confirmation_state not in ['Denied','Confirmed','None']:
return close(intent_request['sessionState']['sessionAttributes'],intent_request['sessionState']['intent']['name'],'Fulfilled','Close','Sorry. I am having trouble completing the request. Let me get someone to help you.')
logger.debug('exiting intent {} here'.format(intent_request['sessionState']['intent']['name']))
raise Exception('Intent with name ' + intent_name + ' not supported')
def prompt_bedrock(formatted_template):
logger.debug('prompt bedrock input is:'.format(formatted_template))
body = json.loads(formatted_template)
modelId = 'anthropic.claude-v2' # change this to use a different version from the model provider
accept = 'application/json'
contentType = 'application/json'
response = bedrock.invoke_model(body=body, modelId=modelId, accept=accept, contentType=contentType)
response_body = json.loads(response.get('body').read())
response_completion = response_body.get('completion')
logger.debug('response is: {}'.format(response_completion))
#print_ww(response_body.get('completion'))
#print(response_body.get('results')[0].get('outputText'))
return response_completion
#function to extract text between the <Response> and </Response> tags within model completion
def extract_response(response_completion):
if '<Response>' in response_completion:
customer_response = response_completion.replace('<Response>','||').replace('</Response>','').split('||')[1]
logger.debug('modified response is: {}'.format(response_completion))
return customer_response
else:
logger.debug('modified response is: {}'.format(response_completion))
return response_completion
#function to extract text between the <Response> and </Response> tags within model completion
def extract_intent(response_completion):
if '<intent>' in response_completion:
customer_intent = response_completion.replace('<intent>','||').replace('</intent>','||').split('||')[1]
return customer_intent
else:
return customer_intent
def close(session_attributes, intent, fulfillment_state, action_type, message):
#This function prepares the response in the appropiate format for Lex V2
response = {
"sessionState": {
"sessionAttributes":session_attributes,
"dialogAction": {
"type": action_type
},
"intent": {
"name":intent,
"state":fulfillment_state
},
},
"messages":
[{
"contentType":"PlainText",
"content":message,
}]
,
}
return response
- इस फ़ंक्शन में आपके द्वारा पहले बनाई गई लैम्ब्डा परत संलग्न करें।
- इसके अतिरिक्त, परत को आपके द्वारा बनाए गए प्रॉम्प्ट टेम्प्लेट में संलग्न करें।
- लैम्ब्डा निष्पादन भूमिका में, अमेज़ॅन बेडरॉक तक पहुंचने के लिए नीति संलग्न करें, जो पहले बनाई गई थी।

लैम्ब्डा निष्पादन भूमिका में निम्नलिखित अनुमतियाँ होनी चाहिए।

अमेज़ॅन लेक्स बॉट में ऑर्केस्ट्रेशन लैम्ब्डा फ़ंक्शन संलग्न करें
- पिछले अनुभाग में फ़ंक्शन बनाने के बाद, अमेज़ॅन लेक्स कंसोल पर वापस लौटें और अपने बॉट पर नेविगेट करें।
- के अंतर्गत भाषाऐं नेविगेशन फलक में, चुनें अंग्रेज़ी.
- के लिए स्रोत, अपना ऑर्डर प्रोसेसिंग बॉट चुनें।
- के लिए लैम्ब्डा फ़ंक्शन संस्करण या उपनाम, चुनें $नवीनतम.
- चुनें सहेजें.

सहायक लैम्ब्डा फ़ंक्शन बनाएं
अतिरिक्त लैम्ब्डा फ़ंक्शन बनाने के लिए निम्नलिखित चरणों को पूरा करें:
- आपके द्वारा पहले बनाई गई DynamoDB तालिका को क्वेरी करने के लिए एक लैम्ब्डा फ़ंक्शन बनाएं:
import json
import boto3
import logging
logger = logging.getLogger()
logger.setLevel(logging.DEBUG)
# Initialize the DynamoDB client
dynamodb = boto3.resource('dynamodb')
table = dynamodb.Table('your-table-name')
def calculate_grand_total(input_data):
# Initialize the total price
total_price = 0
try:
# Loop through each item in the input JSON
for item_id, item_data in input_data.items():
item_name = item_data['item'].lower() # Convert item name to lowercase
item_size = item_data['size'].lower() # Convert item size to lowercase
# Query the DynamoDB table for the item based on Item and Size
response = table.get_item(
Key={'Item': item_name,
'Size': item_size}
)
# Check if the item was found in the table
if 'Item' in response:
item = response['Item']
price = float(item['Price'])
total_price += price # Add the item's price to the total
return total_price
except Exception as e:
raise Exception('An error occurred: {}'.format(str(e)))
def lambda_handler(event, context):
try:
# Parse the input JSON from the Lambda event
input_json = json.loads(event)
# Calculate the grand total
grand_total = calculate_grand_total(input_json)
# Return the grand total in the response
return {'statusCode': 200,'body': json.dumps(grand_total)}
except Exception as e:
return {
'statusCode': 500,
'body': json.dumps('An error occurred: {}'.format(str(e)))
- पर नेविगेट करें विन्यास लैम्ब्डा फ़ंक्शन में टैब करें और चुनें अनुमतियाँ.
- एक संसाधन-आधारित नीति विवरण संलग्न करें जो ऑर्डर प्रोसेसिंग लैम्ब्डा फ़ंक्शन को इस फ़ंक्शन को लागू करने की अनुमति देता है।
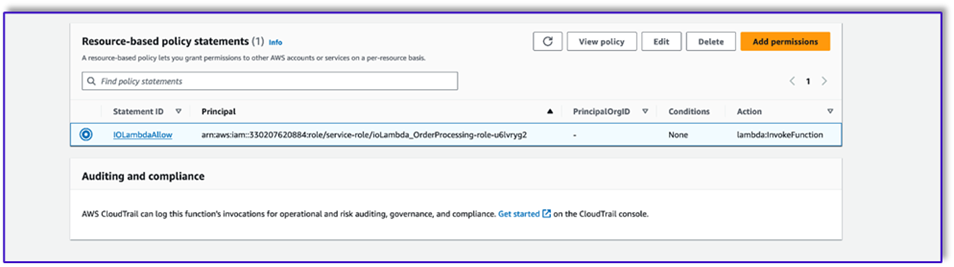
- इस लैम्ब्डा फ़ंक्शन के लिए IAM निष्पादन भूमिका पर नेविगेट करें और DynamoDB तालिका तक पहुंचने के लिए एक नीति जोड़ें।
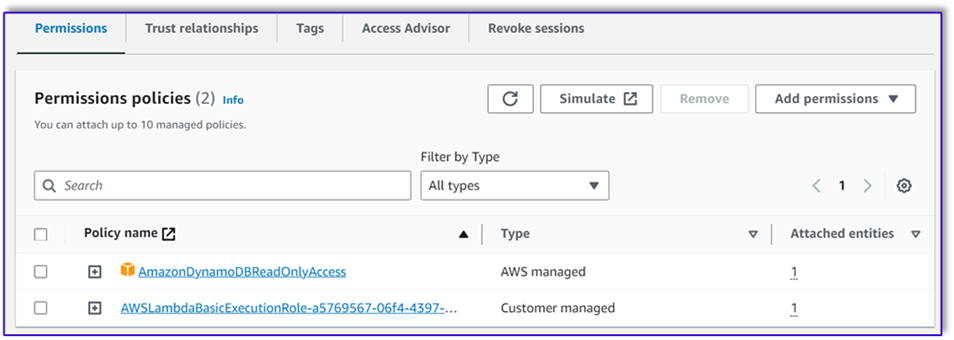
- यह सत्यापित करने के लिए एक और लैम्ब्डा फ़ंक्शन बनाएं कि क्या ग्राहक से सभी आवश्यक विशेषताएँ पारित की गई हैं। निम्नलिखित उदाहरण में, यदि आकार विशेषता किसी ऑर्डर के लिए कैप्चर की गई है तो हम सत्यापित करते हैं:
import json
import logging
logger = logging.getLogger()
logger.setLevel(logging.DEBUG)
def lambda_handler(event, context):
# Define customer orders from the input event
customer_orders = json.loads(event)
# Initialize a list to collect error messages
order_errors = {}
missing_size = []
error_messages = []
# Iterate through each order in customer_orders
for order_id, order in customer_orders.items():
if "size" not in order or order["size"] == "":
missing_size.append(order['item'])
order_errors['size'] = missing_size
if order_errors:
items_missing_size = order_errors['size']
error_message = f"could you please provide the size for the following items: {', '.join(items_missing_size)}?"
error_messages.append(error_message)
# Prepare the response message
if error_messages:
response_message = "n".join(error_messages)
return {
'statusCode': 205,
'validator_response': response_message
}
else:
response_message = "Order is validated successfully"
return {
'statusCode': 200,
'validator_response': response_message
}
- पर नेविगेट करें विन्यास लैम्ब्डा फ़ंक्शन में टैब करें और चुनें अनुमतियाँ.
- एक संसाधन-आधारित नीति विवरण संलग्न करें जो ऑर्डर प्रोसेसिंग लैम्ब्डा फ़ंक्शन को इस फ़ंक्शन को लागू करने की अनुमति देता है।
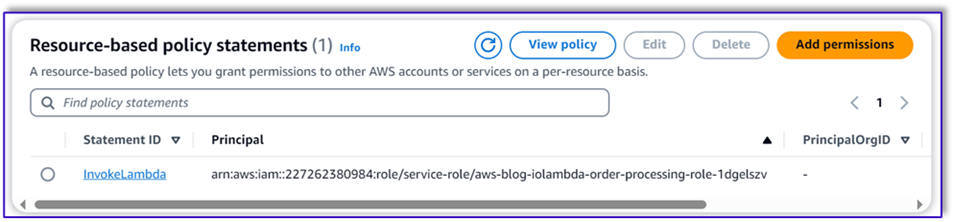
समाधान का परीक्षण करें
अब हम ग्राहकों द्वारा अमेज़ॅन लेक्स के माध्यम से दिए गए ऑर्डर के उदाहरण के साथ समाधान का परीक्षण कर सकते हैं।
हमारे पहले उदाहरण के लिए, ग्राहक ने फ्रैप्पुकिनो मांगा, जो मेनू में नहीं है। मॉडल ऑर्डर वैलिडेटर टेम्पलेट की मदद से सत्यापन करता है और मेनू के आधार पर कुछ सिफारिशें सुझाता है। ग्राहक द्वारा अपने ऑर्डर की पुष्टि करने के बाद, उन्हें ऑर्डर की कुल राशि और ऑर्डर सारांश के बारे में सूचित किया जाता है। ग्राहक की अंतिम पुष्टि के आधार पर ऑर्डर संसाधित किया जाएगा।
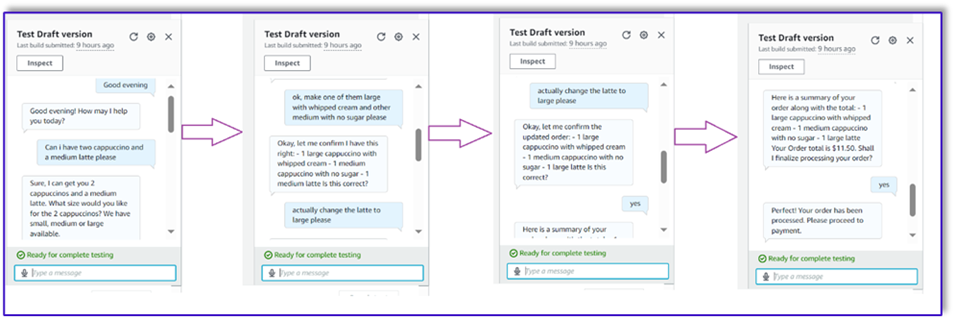
हमारे अगले उदाहरण में, ग्राहक बड़े कैप्पुकिनो का ऑर्डर दे रहा है और फिर आकार को बड़े से मध्यम में संशोधित कर रहा है। मॉडल सभी आवश्यक परिवर्तनों को कैप्चर करता है और ग्राहक से ऑर्डर की पुष्टि करने का अनुरोध करता है। मॉडल कुल ऑर्डर और ऑर्डर सारांश प्रस्तुत करता है, और ग्राहक की अंतिम पुष्टि के आधार पर ऑर्डर को संसाधित करता है।

हमारे अंतिम उदाहरण के लिए, ग्राहक ने कई वस्तुओं के लिए ऑर्डर दिया और कुछ वस्तुओं का आकार गायब है। मॉडल और लैम्ब्डा फ़ंक्शन यह सत्यापित करेगा कि ऑर्डर को संसाधित करने के लिए सभी आवश्यक विशेषताएँ मौजूद हैं या नहीं और फिर ग्राहक से छूटी हुई जानकारी प्रदान करने के लिए कहेंगे। ग्राहक द्वारा अनुपलब्ध जानकारी (इस मामले में, कॉफ़ी का आकार) प्रदान करने के बाद, उन्हें ऑर्डर का कुल और ऑर्डर सारांश दिखाया जाता है। ग्राहक की अंतिम पुष्टि के आधार पर ऑर्डर संसाधित किया जाएगा।
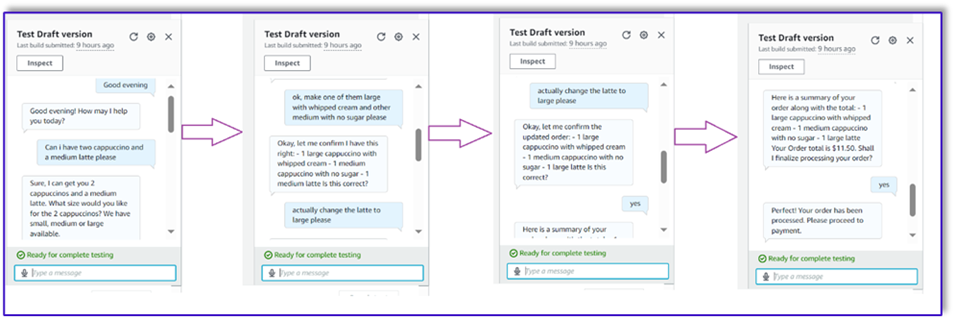
एलएलएम की सीमाएँ
एलएलएम आउटपुट स्वभाव से स्टोकेस्टिक होते हैं, जिसका अर्थ है कि हमारे एलएलएम के परिणाम प्रारूप में या यहां तक कि असत्य सामग्री (मतिभ्रम) के रूप में भिन्न हो सकते हैं। इसलिए, डेवलपर्स को इन परिदृश्यों को संभालने और खराब अंतिम-उपयोगकर्ता अनुभव से बचने के लिए अपने पूरे कोड में एक अच्छे त्रुटि प्रबंधन तर्क पर भरोसा करने की आवश्यकता है।
क्लीन अप
यदि आपको अब इस समाधान की आवश्यकता नहीं है, तो आप निम्नलिखित संसाधनों को हटा सकते हैं:
- लैम्ब्डा कार्य करता है
- अमेज़ॅन लेक्स बॉक्स
- डायनेमोडी टेबल
- S3 बाल्टी
इसके अतिरिक्त, यदि एप्लिकेशन की अब आवश्यकता नहीं है तो सेजमेकर स्टूडियो इंस्टेंस को बंद कर दें।
लागत मूल्यांकन
इस समाधान द्वारा उपयोग की जाने वाली मुख्य सेवाओं के मूल्य निर्धारण की जानकारी के लिए, निम्नलिखित देखें:
ध्यान दें कि आप प्रावधान की आवश्यकता के बिना क्लाउड v2 का उपयोग कर सकते हैं, इसलिए कुल लागत न्यूनतम रहेगी। लागत को और कम करने के लिए, आप DynamoDB तालिका को ऑन-डिमांड सेटिंग के साथ कॉन्फ़िगर कर सकते हैं।
निष्कर्ष
इस पोस्ट में दिखाया गया है कि अमेज़ॅन लेक्स, अमेज़ॅन बेडरॉक और अन्य एडब्ल्यूएस सेवाओं का उपयोग करके भाषण-सक्षम एआई ऑर्डर प्रोसेसिंग एजेंट कैसे बनाया जाए। हमने दिखाया कि कैसे क्लाउड जैसे शक्तिशाली जेनरेटिव एआई मॉडल के साथ त्वरित इंजीनियरिंग व्यापक प्रशिक्षण डेटा की आवश्यकता के बिना ऑर्डर प्रोसेसिंग के लिए मजबूत प्राकृतिक भाषा समझ और वार्तालाप प्रवाह को सक्षम कर सकती है।
समाधान आर्किटेक्चर लचीले और स्केलेबल कार्यान्वयन को सक्षम करने के लिए लैम्ब्डा, अमेज़ॅन एस 3 और डायनेमोडीबी जैसे सर्वर रहित घटकों का उपयोग करता है। Amazon S3 में प्रॉम्प्ट टेम्प्लेट संग्रहीत करने से आप विभिन्न उपयोग मामलों के लिए समाधान को अनुकूलित कर सकते हैं।
अगले कदमों में ग्राहक अनुरोधों और किनारे के मामलों की एक विस्तृत श्रृंखला को संभालने के लिए एजेंट की क्षमताओं का विस्तार करना शामिल हो सकता है। प्रॉम्प्ट टेम्प्लेट एजेंट के कौशल को पुनरावृत्तीय रूप से बेहतर बनाने का एक तरीका प्रदान करते हैं। अतिरिक्त अनुकूलन में ऑर्डर डेटा को इन्वेंट्री, सीआरएम या पीओएस जैसे बैकएंड सिस्टम के साथ एकीकृत करना शामिल हो सकता है। अंत में, एजेंट को अमेज़ॅन लेक्स की मल्टी-चैनल क्षमताओं का उपयोग करके विभिन्न ग्राहक टचप्वाइंट जैसे मोबाइल ऐप, ड्राइव-थ्रू, कियोस्क और अन्य पर उपलब्ध कराया जा सकता है।
अधिक जानने के लिए, निम्नलिखित संबंधित संसाधन देखें:
- मल्टी-चैनल बॉट्स की तैनाती और प्रबंधन:
- क्लाउड और अन्य मॉडलों के लिए शीघ्र इंजीनियरिंग:
- स्केलेबल एआई सहायकों के लिए सर्वर रहित वास्तुशिल्प पैटर्न:
लेखक के बारे में
मौमिता दत्ता अमेज़ॅन वेब सर्विसेज में पार्टनर सॉल्यूशन आर्किटेक्ट हैं। अपनी भूमिका में, वह स्केलेबल और पुन: प्रयोज्य संपत्ति विकसित करने के लिए भागीदारों के साथ मिलकर सहयोग करती है जो क्लाउड तैनाती को सुव्यवस्थित करती है और परिचालन दक्षता को बढ़ाती है। वह एआई/एमएल समुदाय की सदस्य हैं और एडब्ल्यूएस में जेनरेटिव एआई विशेषज्ञ हैं। अपने ख़ाली समय में, वह बागवानी और साइकिल चलाना पसंद करती हैं।
फर्नांडो लैमोग्लिया अमेज़ॅन वेब सर्विसेज में एक पार्टनर सॉल्यूशंस आर्किटेक्ट है, जो व्यावसायिक इकाइयों में अत्याधुनिक एआई समाधानों के विकास और अपनाने की अगुवाई में एडब्ल्यूएस भागीदारों के साथ मिलकर काम कर रहा है। क्लाउड आर्किटेक्चर, जेनरेटिव एआई, मशीन लर्निंग और डेटा एनालिटिक्स में विशेषज्ञता वाला एक रणनीतिक नेता। वह गो-टू-मार्केट रणनीतियों को क्रियान्वित करने और संगठनात्मक लक्ष्यों के अनुरूप प्रभावशाली एआई समाधान प्रदान करने में माहिर हैं। अपने खाली समय में वह अपने परिवार के साथ समय बिताना और दूसरे देशों की यात्रा करना पसंद करते हैं।
मितुल पटेल अमेज़ॅन वेब सर्विसेज में एक वरिष्ठ समाधान वास्तुकार हैं। क्लाउड टेक्नोलॉजी इनेबलर के रूप में अपनी भूमिका में, वह ग्राहकों के साथ उनके लक्ष्यों और चुनौतियों को समझने के लिए काम करते हैं, और एडब्ल्यूएस पेशकशों के साथ उनके उद्देश्य को प्राप्त करने के लिए निर्देशात्मक मार्गदर्शन प्रदान करते हैं। वह एआई/एमएल समुदाय के सदस्य और एडब्ल्यूएस में जेनेरेटिव एआई एंबेसडर हैं। अपने खाली समय में, वह लंबी पैदल यात्रा और फुटबॉल खेलना पसंद करते हैं।